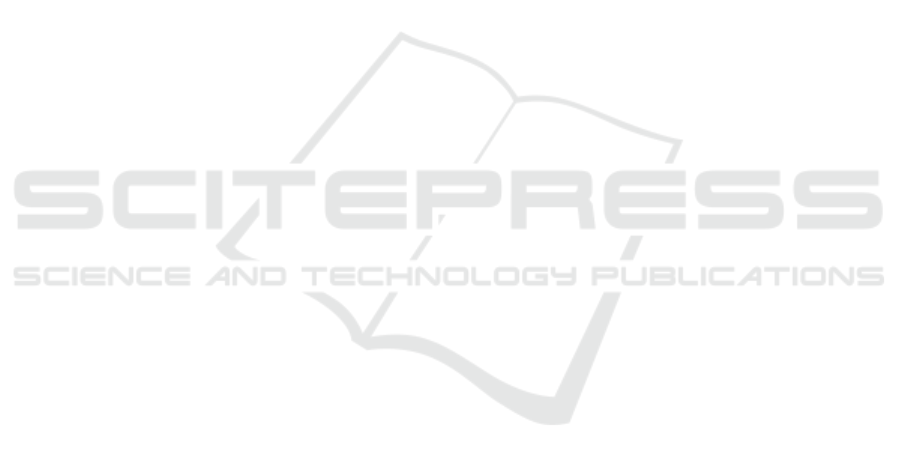
auto-encoder approach for document image binariza-
tion. Pattern Recognition.
Farahmand, A., Sarrafzadeh, H., and Shanbehzadeh, J.
(2017). Noise removal and binarization of scanned
document images using clustering of features.
Gatos, B., Ntirogiannis, K., and Pratikakis, I. (2009). Ic-
dar 2009 document image binarization contest (dibco
2009). In Document Analysis and Recognition, 2009.
ICDAR’09. 10th International Conference on, pages
1375–1382. IEEE.
Goodfellow, I., Pouget-Abadie, J., Mirza, M., Xu, B.,
Warde-Farley, D., Ozair, S., Courville, A., and Ben-
gio, Y. (2014). Generative adversarial nets. In
Advances in neural information processing systems,
pages 2672–2680.
Gulrajani, I., Ahmed, F., Arjovsky, M., Dumoulin, V., and
Courville, A. C. (2017). Improved training of wasser-
stein gans. In Advances in Neural Information Pro-
cessing Systems, pages 5767–5777.
Hedjam, R., Nafchi, H. Z., Kalacska, M., and Cheriet, M.
(2015). Influence of color-to-gray conversion on the
performance of document image binarization: toward
a novel optimization problem. IEEE transactions on
image processing, 24(11):3637–3651.
Isola, P., Zhu, J.-Y., Zhou, T., and Efros, A. A. (2017).
Image-to-image translation with conditional adversar-
ial networks. arXiv preprint.
Jenckel, M., Bukhari, S. S., and Dengel, A. (2016). anyocr:
A sequence learning based ocr system for unlabeled
historical documents. In Pattern Recognition (ICPR),
2016 23rd International Conference on, pages 4035–
4040. IEEE.
Johnson, J., Alahi, A., and Fei-Fei, L. (2016). Perceptual
losses for real-time style transfer and super-resolution.
In European Conference on Computer Vision, pages
694–711. Springer.
Kim, T., Cha, M., Kim, H., Lee, J. K., and Kim, J.
(2017). Learning to discover cross-domain relations
with generative adversarial networks. arXiv preprint
arXiv:1703.05192.
Level Otsu, N. (1979). A threshold selection method from
gray-level histogram. IEEE Trans. Syst. Man Cybern,
9(1):62–66.
Mitianoudis, N. and Papamarkos, N. (2015). Document
image binarization using local features and gaussian
mixture modeling. Image and Vision Computing,
38:33–51.
Niblack, W. (1986). An introduction to digital image pro-
cessing, volume 34. Prentice-Hall Englewood Cliffs.
Ntirogiannis, K., Gatos, B., and Pratikakis, I. (2014).
Icfhr2014 competition on handwritten document im-
age binarization (h-dibco 2014). In Frontiers in
Handwriting Recognition (ICFHR), 2014 14th Inter-
national Conference on, pages 809–813. IEEE.
Pardhi, S. and Kharat, G. (2017). An improved binarization
method for degraded document. In National Confer-
ence MOMENTUM, volume 17.
Pastor-Pellicer, J., Zamora-Mart
´
ınez, F., Espa
˜
na-Boquera,
S., and Castro-Bleda, M. J. (2013). F-measure as
the error function to train neural networks. In Inter-
national Work-Conference on Artificial Neural Net-
works, pages 376–384. Springer.
Peng, X., Cao, H., and Natarajan, P. (2017). Using con-
volutional encoder-decoder for document image bina-
rization. In Document Analysis and Recognition (IC-
DAR), 2017 14th IAPR International Conference on,
volume 1, pages 708–713. IEEE.
Phillips, I. (1996). Users reference manual for the uw en-
glish/technical document image database iii. UW-III
English/Technical Document Image Database Man-
ual.
Pratikakis, I., Zagoris, K., Barlas, G., and Gatos, B. (2017).
Icdar2017 competition on document image binariza-
tion (dibco 2017). In 2017 14th IAPR International
Conference on Document Analysis and Recognition
(ICDAR), pages 1395–1403. IEEE.
Rice, S. V., Jenkins, F. R., and Nartker, T. A. (1996). The
fifth annual test of OCR accuracy. Information Sci-
ence Research Institute.
Sauvola, J. and Pietik
¨
ainen, M. (2000). Adaptive document
image binarization. Pattern recognition, 33(2):225–
236.
Tensmeyer, C. and Martinez, T. (2017). Document im-
age binarization with fully convolutional neural net-
works. In Document Analysis and Recognition (IC-
DAR), 2017 14th IAPR International Conference on,
volume 1, pages 99–104. IEEE.
Wang, T.-C., Liu, M.-Y., Zhu, J.-Y., Tao, A., Kautz, J., and
Catanzaro, B. (2018). High-resolution image synthe-
sis and semantic manipulation with conditional gans.
In IEEE Conference on Computer Vision and Pattern
Recognition (CVPR), volume 1, page 5.
Westphal, F., Lavesson, N., and Grahn, H. (2018). Doc-
ument image binarization using recurrent neural net-
works. In 2018 13th IAPR International Workshop on
Document Analysis Systems (DAS), pages 263–268.
IEEE.
Zemouri, E., Chibani, Y., and Brik, Y. (2014). Enhance-
ment of historical document images by combining
global and local binarization technique. International
Journal of Information and Electronics Engineering,
4(1):1.
Zhu, J.-Y., Park, T., Isola, P., and Efros, A. A. (2017).
Unpaired image-to-image translation using cycle-
consistent adversarial networks. arXiv preprint.
ICPRAM 2019 - 8th International Conference on Pattern Recognition Applications and Methods
154