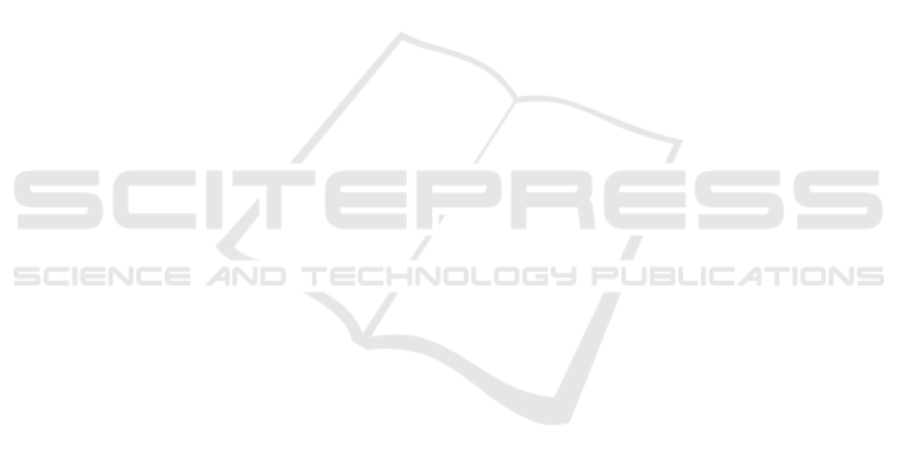
pre-casters with quantitative evidence for the
importance of integrating the risk management with
the PSC. Since allocating resources to conduct the
risk management procedures can help pre-casters not
only in minimizing the probability and impact of the
identified risks, but also in making better operational
decisions such as determining the optimum PCs
sequence to ensure higher service level with
minimum production cost. Hence, investing in
making a reliable risk management plan has dual
benefits to precasters. This can be backed by the study
findings that found that conducting simulation
optimization without considering risks in the PSC, to
find the optimum PCs sequence, may backfire and
lead to PCs sequences which cause high production
costs and vast deviations from the contracted due
dates. Moreover, this study provides the practitioners
with the way to link Arena
®
model with Optquest
®
to
solve precast flow shop sequencing problem.
However, using Optquest
®
for Arena
®
to solve this
problem when considering risks in the PSC took long
time due to high variability. This issue may be
worsened in case of using larger number of decision
variables and replications, which poses a problem for
pre-casters who need quicker way to make such
operational decisions. To remedy this, using another
simulation optimization methods such as response
surface methodology may be fruitful in order to
shorten the optimization time.
In line with this study, other research questions
need to be answered such as how considering the PSC
risks can influence other operational decisions such
as resource allocation and inventory management in
the precast plants.
REFERENCES
Automation, R., 2013. OptQuest for Arena, Rockwell
Automation.
Chan, W.-T., Hu, H., 2001. An application of genetic
algorithms to precast production scheduling.
Computers & Structures, 79(17), 1605–1616.
https://doi.org/10.1016/S0045-7949(01)00036-0
Guide, A., 2001. Project Management Body of Knowledge
(PMBOK® GUIDE). Project Management Institute.
Hu, H., 2007. A study of resource planning for precast
production. Architectural Science Review, 50(2), 106–
114. https://doi.org/10.3763/asre.2007.5016
Ko, C. H., Wang, S. F., 2011. Precast production scheduling
using multi-objective genetic algorithms. Expert
Systems with Applications, 38(7), 8293–8302.
https://doi.org/10.1016/j.eswa.2011.01.013
Law, A. M., 2007. Simulation modeling and analysis,
McGraw-Hill New York, 4
th
edition.
Marler, R. T., Arora, J. S., 2005. Function-transformation
methods for multi-objective optimization. Engineering
Optimization, 37(6), 551–570.
Michalska, K. Mazurkiewicz, J., 2011. Functional and
dependability approach to transport services using
modelling language. International Conference on
Computational Collective Intelligence, Springer, pp.
180–90.
Pheng, L. S., Chuan, C. J., 2001. Just-in-Time Management
of Precast Concrete Components. Journal of
Construction Engineering and Management, 127(6),
494–501. https://doi.org/10.1061/(ASCE)0733-
9364(2001)127:6(494)
Sacks, R., Easman, C. M., Lee, G., 2004. Process model
perspectives on management and engineering
procedures in the precast/prestressed concrete industry.
Journal of Construction Engineering and Management,
130(2), 206–215.
Simchi-Levi, D., Kaminsky, P., Simchi-Levi, E., 2000.
Designing and managing the supply chain. Concepts,
Strategies, and Case Studies.
Tuncel, G., Alpan, G., 2010. Risk assessment and
management for supply chain networks: A case study.
Computers in Industry, 61(3), 250–259.
https://doi.org/10.1016/j.compind.2009.09.008
Wang, Z., Hu, H., 2017. Improved Precast Production –
Scheduling Model Considering the Whole Supply
Chain. Journal of Computing in Civil Engineering,
31(4), 1–12. https://doi.org/10.1061/(ASCE)CP.1943-
5487.0000667.
Wang, Z., Hu, H., 2018. Dynamic response to demand
variability for precast production rescheduling with
multiple lines. International Journal of Production
Research, (January), 1–16.
https://doi.org/10.1080/00207543.2017.1414970
Wang, Z., Hu, H., Gong, J., 2018a. Framework for
modeling operational uncertainty to optimize offsite
production scheduling of precast components.
Automation in Construction, 86(April 2017), 69–80.
https://doi.org/10.1016/j.autcon.2017.10.026
Wang, Z., Hu, H., Gong, J., 2018b. Simulation based
multiple disturbances evaluation in the precast supply
chain for improved disturbance prevention. Journal of
Cleaner Production, 177(March), 232–244.
https://doi.org/10.1016/j.jclepro.2017.12.188
Yang, Z., Ma, Z., Wu, S., 2016. Optimized flowshop
scheduling of multiple production lines for precast
production. Automation in Construction, 72, 321–329.
https://doi.org/10.1016/j.autcon.2016.08.021
Yenisey, M. M., Yagmahan, B., 2014. Multi-objective
permutation flow shop scheduling problem: Literature
review, classification and current trends. Omega, 45,
119–135.
A Simulation based Optimization Study for Optimum Sequencing of Precast Components Considering Supply Chain Risks
337