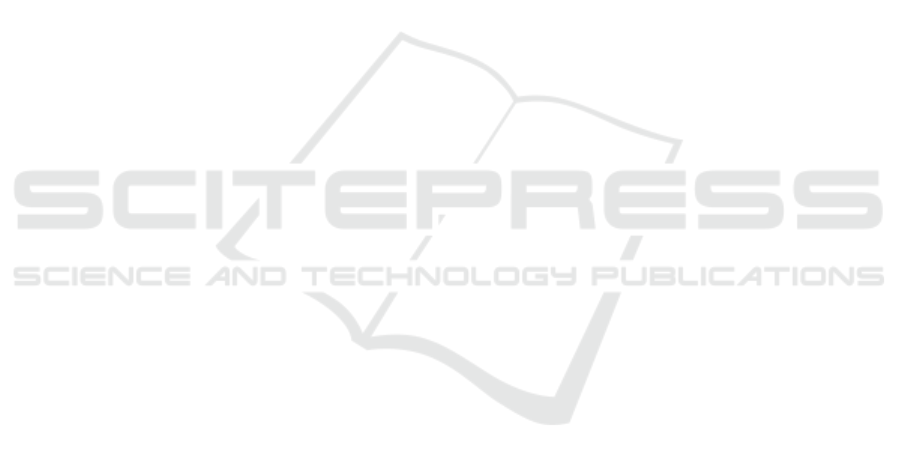
in offline analysis but misses in the live analysis.
Table 4 shows the preliminary results of on-going
experiments with a higher intensity scenario (X = 5s),
a more in-depth vulnerability scanning and more rules
activated on Suricata. The analysis of benign traffic
shows none of those alerts. In these experiments, a
few interesting results appear. Alert 2200069 shows
that there are attacks that Suricata does not detect dur-
ing a live analysis. Alert 2200074 produces more
alerts in mixed traffic than in an attack without be-
nign traffic. On the contrary, we detect fewer alerts
2230010 and 2230015 than expected with the addition
of benign traffic. The analysis of those experiments is
still on-going, but an improvement of the current lim-
itations of the prototype could allow a deeper under-
standing of the behavior of the IDS.
5 CONCLUSION
In this paper, we defined a methodology for the eval-
uation of services and security products. We defined
a set of properties that the evaluation of each target
must respect. Most evaluation tools can only cover
some of those properties, and the challenge of the
evaluator is to successfully compose and orchestrate
the large variety of tools to cover all properties.
Using our recently proposed method to generate
evaluation data, we showed how we could design an
experiment using our network simulation that respects
all the properties of the evaluation of services and se-
curity products. However, our methodology requires
some preparation efforts from the evaluator. The eval-
uator needs to provide model data and scenarios and
must choose an appropriate data generating function.
He must also make topology choice (the use of exter-
nal components, selection and composition of ground
truth, actors interacting with the target, etc.) accord-
ing to his goals and his evaluation target. However,
this method is still at its initial stage. The prepara-
tion effort of the evaluator can be greatly reduce with
the development of further data generating functions,
tools to identify time-sensitive inputs, and the accu-
mulation of model data. Those improvements were
discussed in the previous paper.
To illustrate the proposed evaluation methodol-
ogy, we presented the experimental results of an eval-
uation of a network-based IDS. We evaluate this IDS
with our network simulation using only benign traffic,
only malicious traffic, and mixed traffic. After inci-
dentally evaluating the workload processing capacity
of the external service of our topology, we observed
that the separate evaluation of benign traffic and ma-
licious traffic gave slightly different results than with
mixed traffic. In particular, we observe a difference
in behavior between a live and offline analysis most
likely due to the stress of consequent benign traffic.
However, we also notice that our current proto-
type has limitations and does not support more intense
evaluations of the security product. A more advanced
prototype of the simulation could also provide more
development of the model and the scope of possible
evaluations. It would also be interesting to extend the
experimental results to similar security products and
products of different types.
REFERENCES
Axelsson, S. (2000). The base-rate fallacy and the difficulty
of intrusion detection. ACM Transactions on Informa-
tion and System Security (TISSEC), 3(3):186–205.
Bajan, P.-M., Kiennert, C., and Debar, H. (2018). A new
approach of network simulation for data generation in
evaluating security products. In Internet Monitoring
and Protection, 2018. ICIMP 2018. Thirteenth Inter-
national Conference on. IARIA.
Cowan, C., Arnold, S., Beattie, S., Wright, C., and Viega,
J. (2003). Defcon capture the flag: Defending vulner-
able code from intense attack. In DARPA Information
Survivability Conference and Exposition, 2003. Pro-
ceedings, volume 1, pages 120–129. IEEE.
Cunningham, R. K., Lippmann, R. P., Fried, D. J.,
Garfinkel, S. L., Graf, I., Kendall, K. R., Webster,
S. E., Wyschogrod, D., and Zissman, M. A. (1999).
Evaluating intrusion detection systems without attack-
ing your friends: The 1998 darpa intrusion detection
evaluation. Technical report, Massachusetts Inst. of
Tech. Lexington Lincoln Lab.
Fontugne, R., Borgnat, P., Abry, P., and Fukuda, K. (2010).
Mawilab: combining diverse anomaly detectors for
automated anomaly labeling and performance bench-
marking. In Proceedings of the 6th International
COnference, page 8. ACM.
Garcia-Alfaro, J., Cuppens, F., Cuppens-Boulahia, N., and
Preda, S. (2011). Mirage: a management tool for the
analysis and deployment of network security policies.
In Data Privacy Management and Autonomous Spon-
taneous Security, pages 203–215. Springer.
Gogolla, M. and Hilken, F. (2016). Model validation and
verification options in a contemporary uml and ocl
analysis tool. Modellierung 2016.
Mell, P., Hu, V., Lippmann, R., Haines, J., and Zissman, M.
(2003). An overview of issues in testing intrusion de-
tection systems. Technical report, NIST Interagency.
Migault, D., Girard, C., and Laurent, M. (2010). A perfor-
mance view on dnssec migration. In Network and Ser-
vice Management (CNSM), 2010 International Con-
ference on, pages 469–474. IEEE.
Nahum, E. M., Tracey, J., and Wright, C. P. (2007). Evalu-
ating sip server performance. In ACM SIGMETRICS
Performance Evaluation Review, volume 35, pages
349–350. ACM.
Methodology of a Network Simulation in the Context of an Evaluation: Application to an IDS
387