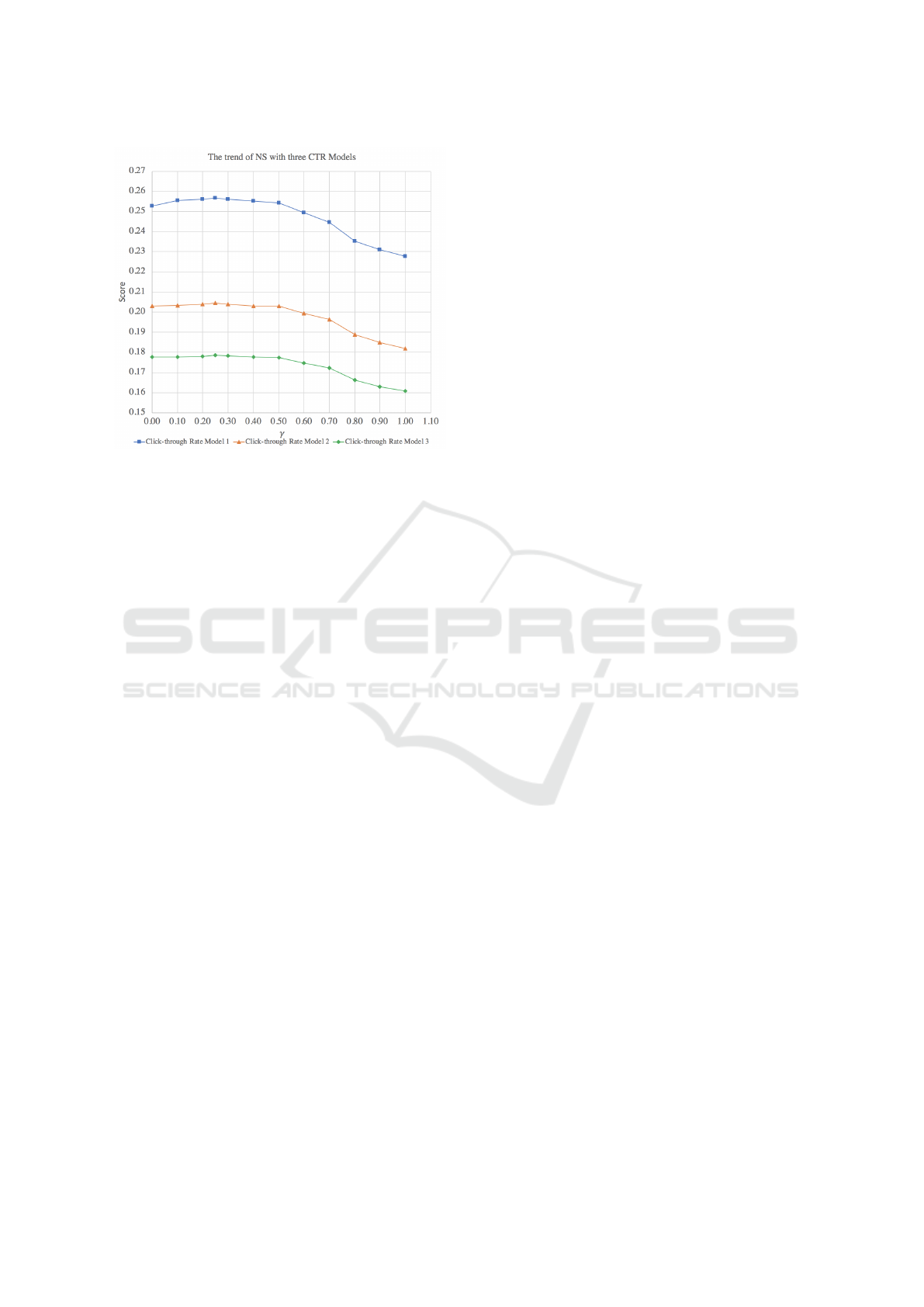
Figure 7: The trend of NS when γ varies from 0 to 1 with
three CTR models.
5.2 Adjusting γ
The parameter γ represents how much the website
prefer EOP rather than EOC. Higher EOP brings the
website more instant income while higher EOC in-
creases the recommendation accuracy, which leads to
better user experience. The larger γ is, the higher EOP
will be and the lower EOC will be.
In practical, NS(R
γ
) is a good way to determine
the specific value of γ, since it takes both EOP and
EOC into consideration. When NS(R
γ
) reaches a
maximum point, further increasing EOP will lead the
EOC decreasing drastically, which results in a worse
overall performance and vice versa. As a result, the
suggested value of γ should be the value that maxi-
mizes NS(R
γ
).
6 CONCLUSIONS
According to the results in section 4, we can conclude
that the novel method PPAARM can find a better so-
lution for placement-and-profit-aware recommenda-
tion problem than traditional methods. This method
gets much higher EOP (Expectation of Profit, a met-
ric of profit) than traditional ARM method with only
little EOC (Expectation of Click Rate, a metric of re-
ommendation accuracy) losses. It can also get much
higher EOC than the traditional WARM method with
only slight decrease in EOP. Further, experiment re-
sults show that PPAARM is robust with different CTR
models.
REFERENCES
Agrawal, R., Imieli
´
nski, T., and Swami, A. (1993). Min-
ing association rules between sets of items in large
databases. SIGMOD Rec., 22(2):207–216.
Agrawal, R. and Srikant, R. (1994). Fast algorithms for
mining association rules in large databases. In Pro-
ceedings of the 20th International Conference on Very
Large Data Bases, VLDB ’94, pages 487–499, San
Francisco, CA, USA. Morgan Kaufmann Publishers
Inc.
Amazon.com (August, 2018). Items Related to Your Pur-
chased. http://www.amazon.com.
Association, A. M. et al. (2014). Dictionary.
Brijs, T., Swinnen, G., Vanhoof, K., and Wets, G. (1999).
Using association rules for product assortment deci-
sions: A case study. In Knowledge Discovery and
Data Mining, pages 254–260.
Cai, C. H., Fu, A. W.-C., Cheng, C., and Kwong, W. (1998).
Mining association rules with weighted items. In
Database Engineering and Applications Symposium,
1998. Proceedings. IDEAS’98. International, pages
68–77. IEEE.
Chuklin, A., Markov, I., and Rijke, M. d. (2015). Click
models for web search. Synthesis Lectures on Infor-
mation Concepts, Retrieval, and Services, 7(3):1–115.
Cox, D. (1958-01-01). The regression analysis of binary
sequences. Journal of the Royal Statistical, Society,
Series B, Methodological, 20(2):215–232.
Fournier-Viger, P., Lin, J. C.-W., Gomariz, A., Gueniche,
T., Soltani, A., Deng, Z., and Lam, H. T. (2016).
The spmf open-source data mining library version 2.
In Machine Learning and Knowledge Discovery in
Databases, volume 9853 of Lecture Notes in Com-
puter Science, pages 36–40. Springer International
Publishing, Cham.
Fournier-Viger, P., Wu, C.-W., and VS, T. (2012). Min-
ing Top-k Association Rules(MTAR). In Advances in
Artificial Intelligence, pages 61–73. Springer, Berlin,
Heidelberg.
Han, J., Pei, J., Yin, Y., and Mao, R. (2004). Min-
ing frequent patterns without candidate generation:
A frequent-pattern tree approach. Data Mining and
Knowledge Discovery, 8(1):53–87.
McMahan, H. B., Holt, G., Sculley, D., Young, M., Ebner,
D., Grady, J., Nie, L., Phillips, T., Davydov, E.,
Golovin, D., et al. (2013). Ad click prediction: a view
from the trenches. In Proceedings of the 19th ACM
SIGKDD international conference on Knowledge dis-
covery and data mining, pages 1222–1230. ACM.
Nguyen, L. T., Vo, B., Nguyen, L. T., Fournier-Viger, P.,
and Selamat, A. (2017). An Efficient Top-k Aas-
sociation Rrule Mmining algorithm. applied intelli-
gence(ETARM). Applied Intelligence, 48:1148–1160.
Robinson, G. B. (1999). Automated collaborative filtering
in world wide web advertising. US Patent 5,918,014.
Schafer, J. B., Frankowski, D., Herlocker, J., and Sen, S.
(2007). Collaborative Filtering Recommender Sys-
tems, pages 291–324. Springer Berlin Heidelberg,
Berlin, Heidelberg.
ICAART 2019 - 11th International Conference on Agents and Artificial Intelligence
646