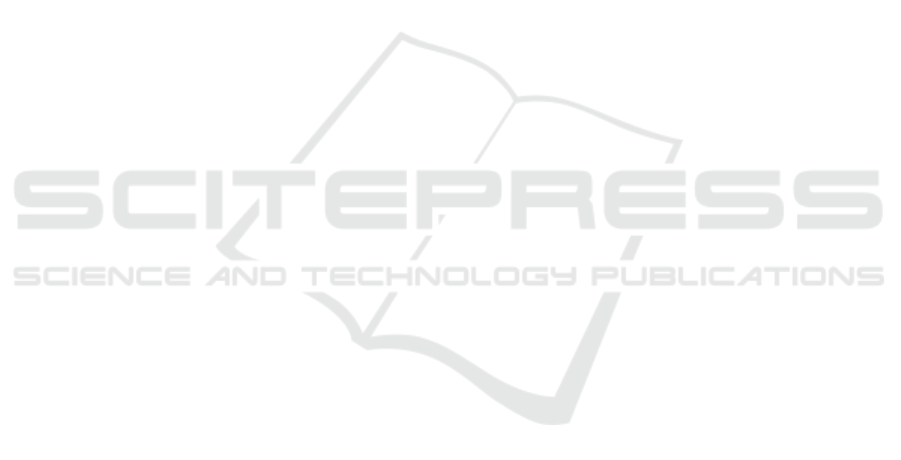
From a geo-scientist’s point of view, the similarity
of ABMs and the concept of VAs or IOAs is a further
interesting aspect: by coupling individual but
corresponding ABM agents and VAs/IOAs, they
could facilitate a quasi in-situ validation of an ABM
simulation unlike the post-simulation validation, as it
is still done today. The latter also has a high potential
to improve our understanding of the environment and
the Earth system, especially in conjunction with time
series of remote sensing data. A further interesting
aspect of coupling agent-based image analysis with
ABMs is their consideration of scale: here
hierarchically organized VAs/IOAs could support the
validation of aggregation and emergence processes of
individual agents in ABMs, such as urbanisation (de-
)forestation or the evolvement of swarms.
REFERENCES
Adhikari, S., Southworth, J., 2012. Simulating Forest Cover
Changes of Bannerghatta National Park Based on a CA-
Markov Model: A Remote Sensing Approach. In:
Remote Sensing 4 (10): 3215–43.
Anders, N. S., Seijmonsbergen, A. C., Bouten W. 2015.
Rule Set Transferability for Object-Based Feature
Extraction: An Example for Cirque Mapping. In:
Photogrammetric Engineering & Remote Sensing 81
(6): 507–14.
Arvor, D., Durieux, L., Andrés, S., Laporte, M.-A., 2013.
Advances in Geographic Object-Based Image Analysis
with Ontologies: A Review of Main Contributions and
Limitations from a Remote Sensing Perspective. In:
ISPRS Journal of Photogrammetry and Remote Sensing
82: 125–37.
Baatz, M., Schäpe, A., 2000. Multiresolution
Segmentation: An Optimization Approach for High
Quality Multi-Scale Image Segmentation. In J. Strobl,
T. Blaschke, G. Griesebner (Eds.): Angewandte
Geographische Informations-Verarbeitung XII,
Wichmann Verlag, Karlsruhe (2000), pp. 12-23.
Belgiu, M., Hofer B., Hofmann, P., 2014. Coupling
Formalized Knowledge Bases with Object-Based
Image Analysis. In: Remote Sensing Letters 5 (6): 530–
38.
Benz, U. C., Hofmann, P., Willhauck, G., Lingenfelder, I.,
Heynen, M., 2004. Multi-Resolution, Object-Oriented
Fuzzy Analysis of Remote Sensing Data for GIS-Ready
Information. In: ISPRS Journal of Photogrammetry and
Remote Sensing 58 (3–4): 239–58.
Biswas, G., Leelawong, K., Schwartz, D., Vye, N., 2005.
Learning by Teaching: A New Agent Paradigm for
Educational Software.” In: Applied Artificial
Intelligence 19 (3–4): 363–92.
Blaschke, T., Strobl, J., 2001. What’s Wrong with
Pixels?: Some Recent Developments Interfacing
Remote Sensing and GIS. In: GeoBIT, 6 (6): 12–17.
Blaschke, T. 2010. Object Based Image Analysis for
Remote Sensing. In: ISPRS Journal of Photogrammetry
and Remote Sensing 65 (1): 2–16.
Blaschke, T., Hay, G. J., Kelly, M., Lang, S., Hofmann, P.,
Addink, E., Feitosa, R. Q., Van der Meer, F., Van der
Werff, H., van Coillie, F., 2014. Geographic Object-
Based Image Analysis–towards a New Paradigm. In:
ISPRS Journal of Photogrammetry and Remote Sensing
87: 180–91.
Borna, K., Moore, A. B., Sirguey, P., 2014. Towards a
Vector Agent Modelling Approach for Remote Sensing
Image Classification. In: Journal of Spatial Science 59
(2): 283–96.
Borna, K., Moore, A. B., Sirguey, P., 2016. An Intelligent
Geospatial Processing Unit for Image Classification
Based on Geographic Vector Agents (GVAs). In:
Transactions in GIS 20 (3): 368–81.
Borna, K., Sirguey, P., Moore, A. B., 2015. An Intelligent
Vector Agent Processing Unit for Geographic Object-
Based Image Analysis. In: Geoscience and Remote
Sensing Symposium (IGARSS), 2015 IEEE
International, 3053–56. IEEE.
Boulton, G., 2018. The Challenges of a Big Data Earth. In:
Big Earth Data 2 (1): 1–7.
Bovenkamp, E. G. P., Dijkstra, J., Bosch, J. G., Reiber, J.
H. C., 2004. Multi-Agent Segmentation of IVUS
Images. In: Pattern Recognition 37 (4): 647–63.
Burnett, C., Blaschke, T., 2003. A Multi-Scale
Segmentation/Object Relationship Modelling
Methodology for Landscape Analysis. In: Ecological
Modelling 168 (3): 233–49.
Canty, M. J. 2014. Image Analysis, Classification and
Change Detection in Remote Sensing: With Algorithms
for ENVI/IDL and Python. CRC Press.
Colwell, R. N. 1968. Remote Sensing of Natural Resources.
In: Scientific American 218 (1): 54–71.
Guo, H. 2017. Big Earth Data: A New Frontier in Earth and
Information Sciences. In: Big Earth Data 1 (1–2): 4–
20.
Heutte, L., Nosary, A., Paquet, T., 2004. A Multiple Agent
Architecture for Handwritten Text Recognition. In:
Pattern Recognition 37 (4): 665–74.
Hofmann, P., 2017. A Fuzzy Belief-Desire-Intention Model
for Agent-Based Image Analysis. In: Modern Fuzzy
Control Systems and Its Applications. InTech. 281-296.
Hofmann, P., Andrejchenko, V., Lettmayer, P.,
Schmitzberger, M., Gruber, M., Ozan, I., Belgiu, M.,
Graf, R., Lampoltshammer, T. J., Wegenkittl, S., 2016.
Agent Based Image Analysis (ABIA)-Preliminary
Research Results from an Implemented Framework. In:
GEOBIA 2016: Solutions and Synergies.
Hofmann, P., Blaschke, T., Strobl, J., 2011. Quantifying the
Robustness of Fuzzy Rule Sets in Object-Based Image
Analysis. In: International Journal of Remote Sensing
32 (22): 7359–81.
Hofmann, P., Lettmayer, P., Blaschke, T., Belgiu, M.,
Wegenkittl, S., Graf, R., Lampoltshammer, T. J.,
Andrejchenko, V., 2014. ABIA – A Conceptual
Framework for Agent Based Image Analysis. In: South-
ICAART 2019 - 11th International Conference on Agents and Artificial Intelligence
184