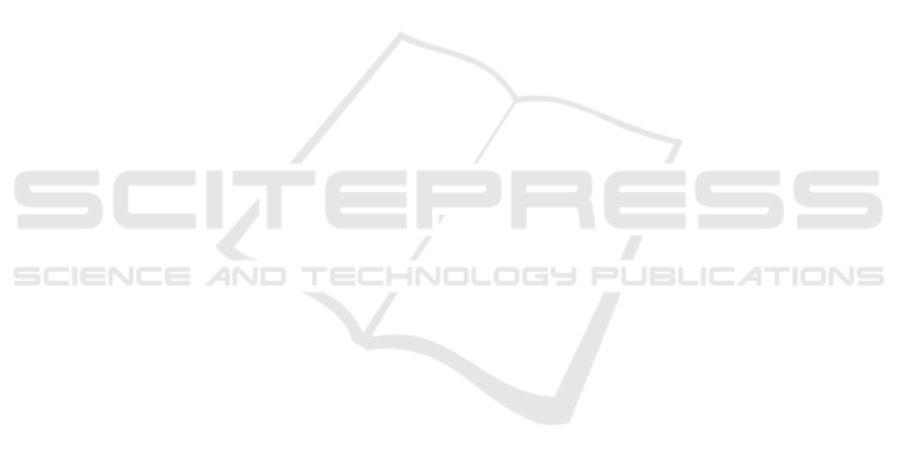
asures evaluated on synthesized data of five different
patients, where the VAs and AAs are separated using
the three introduced approaches TMS, ICA and the
LRSD. The patients are sorted based on the regula-
rity in their VA-containing segments, where the mean
square error between the average of all segments and
the pure VA denoted by MSE
v
is used as the indicator
of the regularity. For all patients, the added pure VA
overlaps with several randomly selected AAs, with
different levels of overlap, except for patient 2 where
we purposely chose samples with no overlap with
AA. The performance of ICA in our simulations is
relatively bad. This might be partly due to (i) the low
quality of the ECG signal we had access to in this
experiment and (ii) the errors in handling the permu-
tation and scaling issues in ICA.
As can be seen, the results with respect to simi-
larity and MSE are quite comparable for all patients,
however as the regularity in VA-containing segments
decreases, the LRSD outperforms the other two ap-
proaches in almost all measures. Only for the first pa-
tient with very regular segments, TMS performs bet-
ter than the proposed LRSD. As previously mentio-
ned, the smoothness is of high importance since the
added distortions might be misinterpreted as fracti-
onated electrograms. However our simulations on
synthesized data show that if the AA is already fracti-
onated, the added distortions after employing TMS
and ICA completely change the morphology of pure
AA, while the LRSD preserves the pure AA morpho-
logy, resulting in better performances.
4 CONCLUSION
In this study we proposed a new framework for re-
moval of VA from atrial electrograms, which is based
on interpolation and subtraction followed by low-rank
and sparse matrix decomposition. The proposed fra-
mework is of low complexity, does not require high
resolution multi-channel recordings, or a calibration
step for each individual patient. The approach outper-
forms the reference methods TMS and ICA with re-
spect to instrumental measures. In particular in cases
where the ventricular activities are less regular. It pro-
vides smooth AA estimates, which is of high impor-
tance for signal processing applications that are based
on the fractionation evaluation in atrial activities.
REFERENCES
Ahmad, A., Salinet, J., Brown, P., Tuan, J. H., Stafford, P.,
Ng, G. A., and Schlindwein, F. S. (2011). Qrs sub-
traction for atrial electrograms: flat, linear and spline
interpolations. Medical & biological engineering &
computing, 49(11):1321–1328.
Boyd, S., Parikh, N., Chu, E., Peleato, B., Eckstein, J.,
et al. (2011). Distributed optimization and statistical
learning via the alternating direction method of mul-
tipliers. Foundations and Trends
R
in Machine lear-
ning, 3(1):1–122.
Hu, Y., Zhang, D., Ye, J., Li, X., and He, X. (2013). Fast
and accurate matrix completion via truncated nuclear
norm regularization. IEEE transactions on pattern
analysis and machine intelligence, 35(9):2117–2130.
Petrutiu, S., Ng, J., Nijm, G. M., Al-Angari, H., Swiryn,
S., and Sahakian, A. V. (2006). Atrial fibrillation and
waveform characterization. IEEE engineering in me-
dicine and biology magazine, 25(6):24–30.
Rieta, J. J., Castells, F., S
´
anchez, C., Zarzoso, V., and
Millet, J. (2004). Atrial activity extraction for
atrial fibrillation analysis using blind source separa-
tion. IEEE Transactions on Biomedical Engineering,
51(7):1176–1186.
Rieta, J. J. and Hornero, F. (2007). Comparative study of
methods for ventricular activity cancellation in atrial
electrograms of atrial fibrillation. Physiological mea-
surement, 28(8):925.
Shkurovich, S., Sahakian, A. V., and Swiryn, S. (1998).
Detection of atrial activity from high-voltage leads of
implantable ventricular defibrillators using a cancel-
lation technique. IEEE Transactions on Biomedical
Engineering, 45(2):229–234.
Xue, Z., Dong, J., Zhao, Y., Liu, C., and Chellali, R. (2018).
Low-rank and sparse matrix decomposition via the
truncated nuclear norm and a sparse regularizer. The
Visual Computer, pages 1–18.
Yaksh, A., van der Does, L. J., Kik, C., Knops, P., Oei, F. B.,
van de Woestijne, P. C., Bekkers, J. A., Bogers, A. J.,
Allessie, M. A., and de Groot, N. M. (2015). A novel
intra-operative, high-resolution atrial mapping appro-
ach. Journal of Interventional Cardiac Electrophysio-
logy, 44(3):221–225.
BIOSIGNALS 2019 - 12th International Conference on Bio-inspired Systems and Signal Processing
184