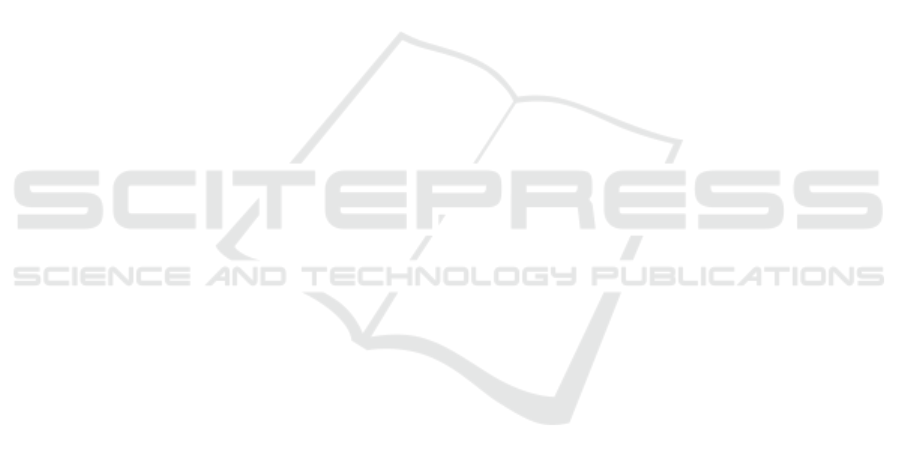
statistical features of signals which remain mostly un-
changed even between different types of cars, and
hence can be used to locate the signals in the CAN
log. We demonstrated that the extracted signals can
be used to effectively identify drivers in a dataset of
33 drivers. Although our results need to be evalu-
ated on a larger and more diverse dataset, our findings
show that driver re-identification can be performed
without the nuisance of signal extraction or agree-
ments with a manufacturer. This means that not re-
vealing the exact signal location in CAN logs is not
sufficient to provide any privacy guarantee in prac-
tice. Car companies should devise more principled
(perhaps cryptographic) approaches to hide signals,
and/or to anonymize their CAN logs so that drivers
cannot be re-identified.
ACKNOWLEDGEMENTS
This work has been partially funded by the Eu-
ropean Social Fund via the project EFOP-3.6.2-
16-2017-00002, by the European Commission via
the H2020-ECSEL-2017 project SECREDAS (Grant
Agreement no. 783119) and the Higher Education
Excellence Program of the Ministry of Human Ca-
pacities in the frame of Artificial Intelligence re-
search area of Budapest University of Technology and
Economics (BME FIKP-MI/FM). Gergely Acs has
been supported by the Premium Post Doctorate Re-
search Grant of the Hungarian Academy of Sciences
(MTA). Gergely Bicz
´
ok has been supported by the
J
´
anos Bolyai Research Scholarship of the Hungarian
Academy of Sciences.
REFERENCES
Bagnall, A., Bostrom, A., Large, J., and Lines, J. (2016).
The great time series classification bake off: An exper-
imental evaluation of recently proposed algorithms.
extended version. arXiv preprint arXiv:1602.01711.
Enev, M., Takakuwa, A., Koscher, K., and Kohno, T.
(2016). Automobile driver fingerprinting. Proceed-
ings on Privacy Enhancing Technologies, 2016(1):34–
50.
European Union (2016a). Recital 26 of Regula-
tion (EU) 2016/679 of the European Parliament
and of the Council of 27 April 2016 on the
protection of natural persons with regard to the
processing of personal data and on the free
movement of such data, and repealing Direc-
tive 95/46/EC (General Data Protection Regulation),
OJ L119, 4.5.2016. http://eur-lex.europa.eu/legal-
content/en/TXT/?uri=CELEX%3A32016R0679.
European Union (2016b). Regulation (EU) 2016/679 of
the European Parliament and of the Council of 27
April 2016 on the protection of natural persons with
regard to the processing of personal data and on the
free movement of such data, and repealing Directive
95/46/EC (General Data Protection Regulation). Offi-
cial Journal of the European Union, L119:1–88.
Fugiglando, U., Massaro, E., Santi, P., Milardo, S., Abida,
K., Stahlmann, R., Netter, F., and Ratti, C. (2018).
Driving behavior analysis through can bus data in an
uncontrolled environment. IEEE Transactions on In-
telligent Transportation Systems, (99).
Fugiglando, U., Santi, P., Milardo, S., Abida, K., and Ratti,
C. (2017). Characterizing the driver dna through can
bus data analysis. In Proceedings of the 2nd ACM
International Workshop on Smart, Autonomous, and
Connected Vehicular Systems and Services, pages 37–
41. ACM.
Geurts, P. (2001). Pattern extraction for time series clas-
sification. In European Conference on Principles of
Data Mining and Knowledge Discovery, pages 115–
127. Springer.
Hallac, D., Sharang, A., Stahlmann, R., Lamprecht, A.,
Huber, M., Roehder, M., Leskovec, J., et al. (2016).
Driver identification using automobile sensor data
from a single turn. In Intelligent Transportation Sys-
tems (ITSC), 2016 IEEE 19th International Confer-
ence on, pages 953–958. IEEE.
Markovitz, M. and Wool, A. (2017). Field classification,
modeling and anomaly detection in unknown can bus
networks. Vehicular Communications, 9:43–52.
Miyajima, C., Nishiwaki, Y., Ozawa, K., Wakita, T., Itou,
K., Takeda, K., and Itakura, F. (2007). Driver mod-
eling based on driving behavior and its evaluation
in driver identification. Proceedings of the IEEE,
95(2):427–437.
Salvador, S. and Chan, P. (2007). Toward accurate dynamic
time warping in linear time and space. Intelligent Data
Analysis, 11(5):561–580.
Sija, B. D., Goo, Y.-H., Shim, K.-S., Hasanova, H., and
Kim, M.-S. (2018). A survey of automatic protocol re-
verse engineering approaches, methods, and tools on
the inputs and outputs view. Security and Communi-
cation Networks, 2018.
Szalay, Z., K
´
anya, Z., Lengyel, L., Ekler, P., Ujj, T.,
Balogh, T., and Charaf, H. (2015). Ict in road ve-
hicles—reliable vehicle sensor information from obd
versus can. In Models and Technologies for Intelligent
Transportation Systems (MT-ITS), 2015 International
Conference on, pages 469–476. IEEE.
Voss, W. (2008). A comprehensible guide to controller area
network. Copperhill Media.
Extracting Vehicle Sensor Signals from CAN Logs for Driver Re-identification
145