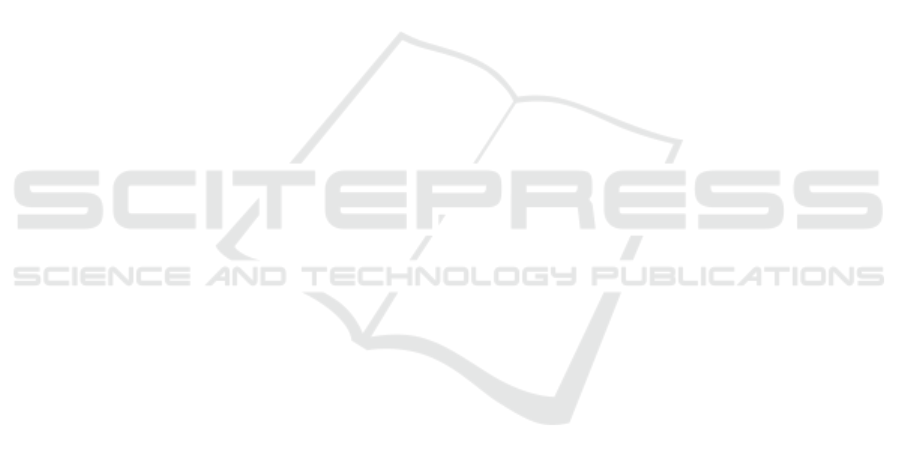
that it is a promising new tool for central banks to
monitor the financial activities of banks.
There are several ways in which our method can
be improved. Our classifiers were trained on data of
only seven banks. It is to be expected that the classi-
fiers perform much better when they are trained on a
larger dataset containing a more diverse set of stress
events. Moreover, the generation of the stress classes
by our news analysis still involved a lot of manual
work. Future research could investigate whether this
step can be automated by applying techniques of nat-
ural language processing or sentiment analysis.
REFERENCES
Altman, E. I. (1968). Financial ratios, discriminant anal-
ysis and the prediction of corporate bankruptcy. The
Journal of Finance, 23(4):589–609.
Arciero, L., Heijmans, R., Heuver, R., Massarenti, M., Pi-
cillo, C., and Vacirca, F. (2016). How to measure
the unsecured money market: the Eurosystem’s im-
plementation and validation using TARGET2 data. In-
ternational Journal of Central Banking.
Beaver, W. H. (1966). Financial ratios as predictors of fail-
ure. Journal of Accounting Research, pages 71–111.
BIS (2008). Principles for sound liquidity risk management
and supervision. Bank for International Settlements.
Bottou, L. (2004). Stochastic learning. In Advanced Lec-
tures on Machine Learning, pages 146–168.
Chamon, M., Manasse, P., and Prati, A. (2007). Can we pre-
dict the next capital account crisis? IMF Staff Papers,
54(2):270–305.
Davis, E. P. and Karim, D. (2008). Comparing early warn-
ing systems for banking crises. Journal of Financial
stability, 4(2):89–120.
Demirg-Kunt, A. and Detragiache, E. (1998). The deter-
minants of banking crises in developing and devel-
oped countries. Staff Papers (International Monetary
Fund), 45(1):81–109.
ECB (2018). TARGET2 user information guide v12.0. ECB
Website.
Fioramanti, M. (2008). Predicting sovereign debt crises
using artificial neural networks: a comparative ap-
proach. Journal of Financial Stability, 4(2):149–164.
Furfine, C. H. (1999). The microstructure of the federal
funds market. Financial Markets, Institutions & In-
struments, 8(5):24–44.
Glorot, X., Bordes, A., and Bengio, Y. (2011). Deep sparse
rectifier neural networks. In Proceedings of the Four-
teenth International Conference on Artificial Intelli-
gence and Statistics, pages 315–323.
Heijmans, R. and Heuver, R. (2014). Is this bank ill? the
diagnosis of doctor TARGET2. The Journal of Finan-
cial Market Infrastructures, 2 nr 3:3–36.
Hirschman, A. O. (1945). National power and the structure
of foreign trade. University of California Press.
Kaminsky, G. L. and Reinhart, C. M. (1999). The
twin crises: the causes of banking and balance-of-
payments problems. The American Economic Review,
89(3):473–500.
Krogh, A. and Hertz, J. A. (1992). A simple weight decay
can improve generalization. In Advances in Neural
Information Processing Systems 4, pages 950–957.
Kuhn, M. (2008). Building predictive models in R using the
caret package. Journal of Statistical Software, 28.
Kumar, P. R. and Ravi, V. (2007). Bankruptcy predic-
tion in banks and firms via statistical and intelligent
techniques–a review. European Journal of Opera-
tional Research, 180(1):1–28.
LeCun, Y., Bottou, L., Orr, G. B., and M
¨
uller, K. R. (1998).
Efficient BackProp, pages 9–50. Springer Berlin Hei-
delberg.
Min, J. H. and Lee, Y.-C. (2005). Bankruptcy prediction
using support vector machine with optimal choice of
kernel function parameters. Expert Systems with Ap-
plications, 28(4):603–614.
Niculescu-Mizil, A. and Caruana, R. (2005). Predicting
good probabilities with supervised learning. In Pro-
ceedings of the 22nd International Conference on Ma-
chine Learning, pages 625–632.
Tam, K. Y. (1991). Neural network models and the predic-
tion of bank bankruptcy. Omega, 19(5):429–445.
Triepels, R., Daniels, H., and Heijmans, R. (2018). De-
tection and explanation of anomalous payment behav-
ior in real-time gross settlement systems. In Lec-
ture Notes in Business Information Processing, vol-
ume 321, pages 145–161.
Werbos, P. J. (1982). Applications of advances in nonlin-
ear sensitivity analysis. In System Modeling and Op-
timization, pages 762–770.
ICAART 2019 - 11th International Conference on Agents and Artificial Intelligence
274