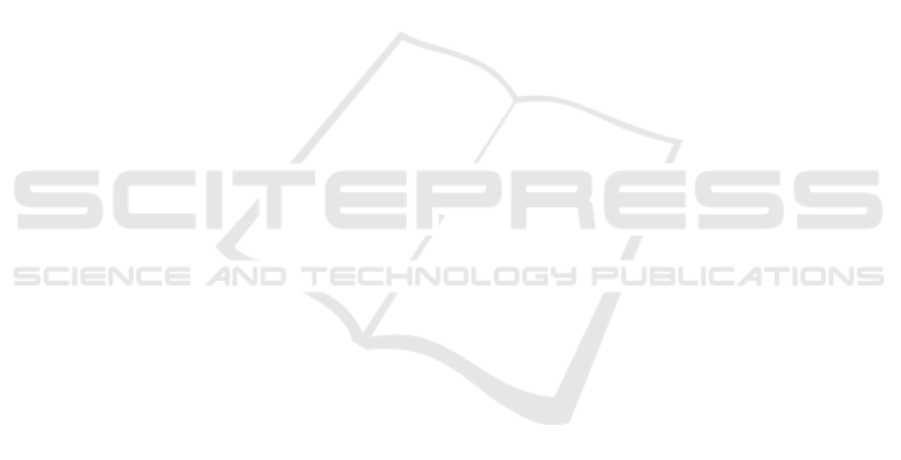
maps. After this detection, the attempt of matching
among these candidates is realized and then the gen-
erated maps are evaluated through a metric of similar-
ity.
The quantitative analysis of matchings expressed
the difference in precision between the mapping with
only one robot and the result of the matching do not
have considerable differences. Thus, matching can be
considered a viable tool for mapping processes with
more than one robot, but it remains to verify the navi-
gability of both maps, which should be done in future
work.
Through this method, good results were obtained,
in order to make possible future tests in real robots
and the continuity of the research. In order to improve
the results obtained, it is suggested to apply proba-
bilistic functions to verify if there is success or failure
in the matching process, in addition to trying to in-
crease the number of success cases.
ACKNOWLEDGEMENTS
The authors acknowledge FAPEMA, CAPES and
CNPq for their financial support in the development
of this work. Special thanks to CEUMA, UEMA and
UFMA for their technical support.
REFERENCES
Birk, A. and Carpin, S. (2006). Merging occupancy grid
maps from multiple robots. Proceedings of the IEEE,
94(7):1384–1397.
Blanco, J.-L., Gonzlez-Jimnez, J., and Fernndez-Madrigal,
J.-A. (2013). A robust, multi-hypothesis approach
to matching occupancy grid maps. Robotica,
31(5):687701.
Bresson, G., Aufrere, R., and Chapuis, R. (2013). Consis-
tent multi-robot decentralized SLAM with unknown
initial positions. Information Fusion (FUSION), 2013,
pages 372–379.
Cunningham, A., Wurm, K. M., Burgard, W., and Del-
laert, F. (2012). Fully distributed scalable smooth-
ing and mapping with robust multi-robot data asso-
ciation. Proceedings - IEEE International Conference
on Robotics and Automation, pages 1093–1100.
Dantas, D. d. O. (2017). Implementao de um sistema aut-
nomo de construo de estrutura usando aprendizado
por reforo. Master’s thesis. DEPARTAMENTO DE
INFORMTICA/CCET.
das Grac¸as Medeiros, N., da Silva, E. A., and Nogueira,
J. R. (2002). Segmentac¸
˜
ao morfol
´
ogica de imagens
utilizando o gradiente morfol
´
ogico multi-escala. Re-
vista Brasileira de Cartografia, (54).
Elfes, A. (1989). Occupancy Grids: A Probabilistic Frame-
work for Robot Perception and Navigation. PhD the-
sis, Pittsburgh, PA, USA. AAI9006205.
Gonzalez, R. C. and Woods, R. E. (2010). Processamento
digital de imagens . Pearson Prentice Hall.
Grisetti, G., Stachniss, C., and Burgard, W. (2007).
Improved techniques for grid mapping with rao-
blackwellized particle filters. IEEE Trans Robot 23.
Harris, C. and Stephens, M. (1988). A combined corner and
edge detector. In Alvey vision conference, volume 15,
page 50. Citeseer.
Jaccard, P. (1912). The distribution of the flora in the alpine
zone. New phytologist, 11(2):37–50.
Jelinek, L. (2016). Vrep map generator. Accesso: 15-03-
2018.
Jian, L., Chen, Z., Qiang, I., Heng, W., and Manzhen, M.
(2017). Vision feature extraction algorithm for occu-
pancy grid maps merging. In Proceedings of the 2017
2Nd International Conference on Communication and
Information Systems, ICCIS 2017, pages 290–293,
New York, NY, USA. ACM.
Koch, P., May, S., Schmidpeter, M., K
¨
uhn, M., Pfitzner, C.,
Merkl, C., Koch, R., Fees, M., Martin, J., Ammon, D.,
and N
¨
uchter, A. (2016). Multi-robot localization and
mapping based on signed distance functions. Journal
of Intelligent & Robotic Systems, 83(3):409–428.
Lopes, F. M. (2012). Um modelo perceptivo de limiarizac¸
˜
ao
de imagens digitais.
Milsztajn, F. and de Geus, K. (2015). Segmentao de tecidos
cerebrais em imagens tridimensionais do crebro.
ROS (201?). Documentation = http://wiki.ros.org/, note =
Accessed: 2017-04-09,.
Shi, J. and Tomasi, C. (1994). Good features to track. In
1994 Proceedings of IEEE Conference on Computer
Vision and Pattern Recognition, pages 593–600.
Thrun, S. and Liu, Y. (2005). Multi-robot SLAM with
Sparse Extended Information Filers. The Interna-
tional Journal of Robotics Research, 15:254–266.
u. N. Jafri, S. R. and Chellali, R. (2013). A distributed multi
robot slam system for environment learning. In 2013
IEEE Workshop on Robotic Intelligence in Informa-
tionally Structured Space (RiiSS), pages 82–88.
Map Matching for SLAM with Multiple Robots in Different Moments
217