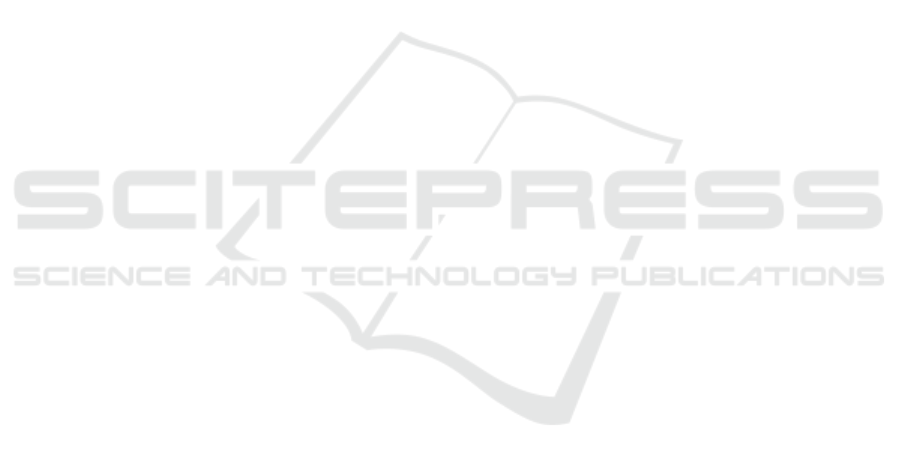
formation obtained by the question processing. The
module was trained on the development set of 50% of
the SQAD questions and evaluated with the testing set
of the same size. The resulting precision was 89% for
question types and 85% for answer types with the re-
spective recall of 88% and 85%. The combined over-
all F1 measure was 83%. The error analysis of the
detection module directs the future work to employ-
ment of named entity recognition and word embed-
ding similarity score for question keywords missing
in the Czech Wordnet.
The open domain question answering system
AQA was evaluated with the previous version of the
SQAD database, where it was able to point at the cor-
rect answer in 46%. The newly implemented question
and answer type detection module aims at improving
this result in the AQA evaluation. Apart from the an-
swer type extraction module, a new module for AQA
answer selection is currently in development and it is
also planned for evaluation with the new SQAD v2.1
benchmark dataset.
ACKNOWLEDGEMENTS
This work has been partly supported by the Czech
Science Foun-dation under the project GA18-23891S.
REFERENCES
Horák, A. and Medved’, M. (2014). SQAD: Simple Ques-
tion Answering Database. In Eighth Workshop on Re-
cent Advances in Slavonic Natural Language Process-
ing, RASLAN 2014, pages 121–128, Brno. Tribun EU.
Hu, M., Peng, Y., Huang, Z., Yang, N., Zhou, M., et al.
(2018). Read + Verify: Machine reading compre-
hension with unanswerable questions. arXiv preprint
arXiv:1808.05759.
Medved’, M. and Horák, A. (2016). AQA: Automatic
Question Answering System for Czech. In Sojka, P.
et al., editors, Text, Speech, and Dialogue 19th Inter-
national Conference, TSD 2016 Brno, Czech Repub-
lic, September 12–16, 2016 Proceedings, pages 270–
278, Switzerland. Springer International Publishing.
Medved’, M. and Horák, A. (2018). Sentence and word
embedding employed in open question-answering. In
Proceedings of the 10th International Conference on
Agents and Artificial Intelligence (ICAART 2018),
pages 486–492, Setúbal, Portugal. SCITEPRESS -
Science and Technology Publications.
Pamela, F., Danilo, G., Bernardo, M., Anselmo, P., Rodrigo,
Á., and Sutcliffe, R. (2010). Evaluating multilingual
question answering systems at clef. In Seventh confer-
ence on International Language Resources and Eval-
uation (LREC’10).
Rajpurkar, P., Zhang, J., Lopyrev, K., and Liang, P. (2016).
SQuAD: 100,000+ questions for machine comprehen-
sion of text. In Proceedings of the 2016 Conference
on Empirical Methods in Natural Language Process-
ing, EMNLP 2016, pages 2383–2392. Association for
Computational Linguistics.
Rambousek, A., Pala, K., and Tuka
ˇ
cová, S. (2017).
Overview and Future of Czech Wordnet. In McCrae,
J. P., Bond, F., Buitelaar, P., Cimiano, P., 4, T. D.,
Gracia, J., Kernerman, I., Ponsoda, E. M., Ordan, N.,
and Piasecki, M., editors, LDK Workshops: OntoLex,
TIAD and Challenges for Wordnets, pages 146–151,
Galway, Ireland. CEUR-WS.org.
Šmerk, P. (2009). Fast Morphological Analysis of Czech. In
Proceedings of Recent Advances in Slavonic Natural
Language Processing, RASLAN 2009, pages 13–16.
Šmerk, Pavel (2010). K poˇcítaˇcové morfologické analýze
ˇceštiny (in Czech, Towards Computational Morpho-
logical Analysis of Czech). PhD thesis, Faculty of In-
formatics, Masaryk University.
Šulganová, T., Medved’, M., and Horák, A. (2017). En-
largement of the Czech Question-Answering Dataset
to SQAD v2.0. In Proceedings of Recent Advances
in Slavonic Natural Language Processing, RASLAN
2017, pages 79–84.
Wang, W., Yan, M., and Wu, C. (2018). Multi-granularity
hierarchical attention fusion networks for reading
comprehension and question answering. In Proceed-
ings of the 56th Annual Meeting of the Association for
Computational Linguistics (Volume 1: Long Papers),
volume 1, pages 1705–1714.
ICAART 2019 - 11th International Conference on Agents and Artificial Intelligence
706