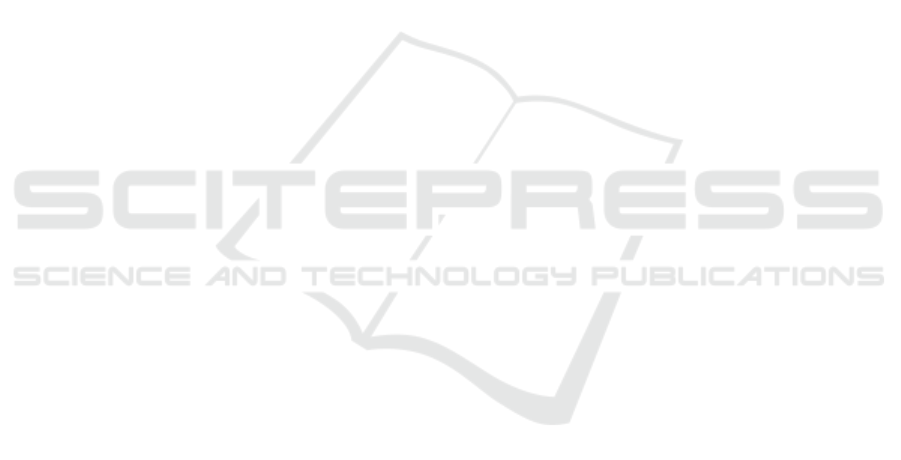
size and direction of an object in the image.
Consequently, there is a need to explore and evaluate
a range of pre-processing procedures to introduce the
necessary privacy required and tackle image factors.
6 CONCLUSIONS
In this paper, the performance of existing commercial
AIA systems, as well as the proposed multi-
algorithmic approach were evaluated. The
experimental results using two datasets show that the
proposed method outperforms the existing AIA
systems. The proposed method annotated the image
with many correct and accurate words that reflecting
image content and will later improve the retrieval
performance. The results also argued that the
proposed approach improved the efficiency and
accuracy of the image annotation comparable to the
state of the art works.
Future work, however, needs to seek, explore and
evaluate a range of pre-processing procedures to
achieve the necessary privacy. Furthermore,
additional research in image enhancement should be
conducted to improve image quality that would
improve the annotation systems performance, thereby
improving the performance of the multi-algorithmic
approach.
REFERENCES
Bahrami, S. and Abadeh, M.S., 2014. Automatic Image
Annotation Using an Evolutionary Algorithm ( IAGA
). 2014 7th International Symposium on
Telecommunications (IST’2014), pp.320–325.
Bhargava, A., 2014. An Object Based Image Retrieval
Framework Based on Automatic Image Annotation.
Calrifai, 2018. API | Clarifai. Available at:
https://www.clarifai.com/api.
Al Fahdi, M. et al., 2016. A suspect-oriented intelligent and
automated computer forensic analysis. Digital
Investigation, 18, pp.65–76. Available at:
http://linkinghub.elsevier.com/retrieve/pii/S17422876
16300792.
Forensicsciencesimplified.org, 2016. Forensic Audio and
Video Analysis: How It’s Done. Available at:
http://www.forensicsciencesimplified.org/av/how.html
.
Google Cloud Platform, 2017. Vision API - Image Content
Analysis | Google Cloud Platform. Available at:
https://cloud.google.com/vision/ [Accessed April 10,
2017].
Hidajat, M., 2015. Annotation Based Image Retrieval using
GMM and Spatial Related Object Approaches. , 8(8),
pp.399–408.
Hou, A. and Wang, C., 2014. Automatic Semantic
Annotation for Image Retrieval Based on Multiple
Kernel Learning. , (Lemcs).
Imagga.com, 2016. imagga - powerful image recognition
APIs for automated categorization andamp; tagging.
Available at: https://imagga.com/.
Inoue, M., 2004. On the need for annotation-based image
retrieval. in: IRiX’04: Proceedings of the ACM-SIGIR
Workshop on Information Retrieval in Context,
Sheffield, UK, pp.44–46. Available at:
https://pdfs.semanticscholar.org/af7c/e5f0531a660782
535dd954445c530a0c34b0.pdf [Accessed June 23,
2017].
Lee, J. et al., 2011. Image Retrieval in Forensics:
Application to Tattoo Image Database. IEEE
Multimedia.
Li, Z. et al., 2012. Combining Generative/Discriminative
Learning for Automatic Image Annotation and
Retrieval. International Journal of Intelligence Science,
2(3), pp.55–62. Available at:
http://www.scirp.org/journal/PaperDownload.aspx?D
OI=10.4236/ijis.2012.23008.
Majidpour, J. et al., 2015. Interactive tool to improve the
automatic image annotation using MPEG-7 and multi-
class SVM. In 2015 7th Conference on Information and
Knowledge Technology (IKT). IEEE, pp. 1–7.
Available at:
http://ieeexplore.ieee.org/document/7288777/.
Microsoft Cognitive Services, 2017. Microsoft Cognitive
Services - Computer Vision API. Available at:
https://www.microsoft.com/cognitive-services/en-
us/computer-vision-api [Accessed April 10, 2017].
Murthy, V.N., Can, E.F. and Manmatha, R., 2014. A Hybrid
Model for Automatic Image Annotation. In
Proceedings of International Conference on
Multimedia Retrieval - ICMR ’14. New York, New
York, USA: ACM Press, pp. 369–376. Available at:
http://dl.acm.org/citation.cfm?doid=2578726.2578774.
Oujaoura, M., Minaoui, B. and Fakir, M., 2014. Combined
descriptors and classifiers for automatic image
annotation.
Redi, J.A., Taktak, W. and Dugelay, J.-L., 2011. Digital
image forensics: a booklet for beginners. Multimedia
Tools and Applications, 51(1), pp.133–162. Available
at: http://link.springer.com/10.1007/s11042-010-0620-
1.
Singh, A., 2015. Exploring Forensic Video And Image
Analysis. Available at:
https://www.linkedin.com/pulse/exploring-forensic-
video-image-analysis-ashish-singh.
Sreedhanya, S. and Chhaya, S.P., 2017. Automatic Image
Annotation Using Modified Multi-label Dictionary
Learning. International Journal of Engineering and
Techniques, 3(5). Available at:
http://www.ijetjournal.org [Accessed March 9, 2018].
Sumathi, T. and Hemalatha, M., 2011. A combined
hierarchical model for automatic image annotation and
retrieval. In 2011 Third International Conference on
Advanced Computing. IEEE, pp. 135–139. Available
Identification and Extraction of Digital Forensic Evidence from Multimedia Data Sources using Multi-algorithmic Fusion
447