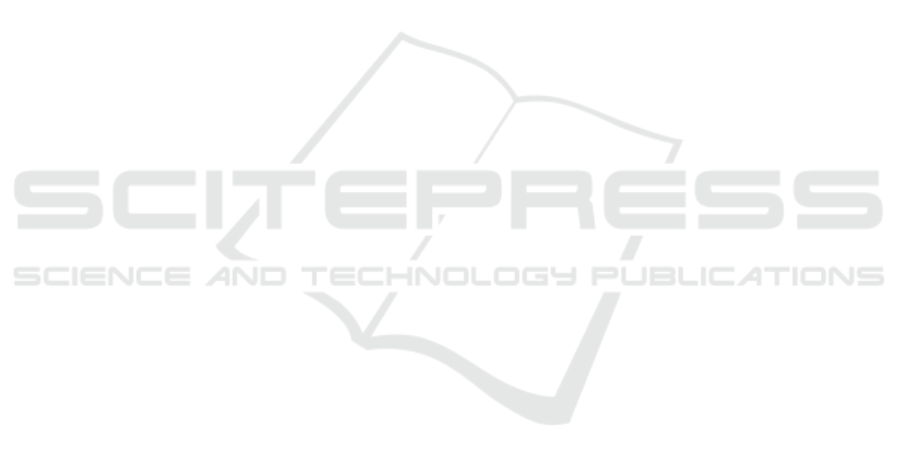
Development (CNPq - 304843/2016-4) and FAPERJ
(202.673/2018).
REFERENCES
OTexts, “Forecasting: Principles and Practice, Advanced
Forecasting Methods, Dynamic Regression”. Acessed:
October 2018. https://otexts.org/fpp2/dynamic.html
Almutairi, A; Hassan Ahmed, M.; Salama, M. M. A., 2016.
“ Use of MCMC to incorporate a wind power model for
the evaluation of generating capacity adequacy”.
Electric Power Systems Research, v. 133, p. 63–70,
2016.
Alexiadis, M. C. et al. Short-term forecasting os Wind
speed and related electrical power. Solar Energy, v. 83,
p. 61–68, 1998.
Almutairi, A.; Hassan Ahmed, M.; Salama, M. M. A. Use
of MCMC to incorporate a wind power model for the
evaluation of generating capacity adequacy. Electric
Power Systems Research, v. 133, p. 63–70, 2016.
Aneel. Big - Banco de Informações de Geração [Generation
Information Bank]. Available in:
<http://www2.aneel.gov.br/aplicacoes/capacidadebrasi
l/capacidadebrasil.cfm>. Access in: 5 nov. 2017.
Billinton, R.; Chen, H.; Ghajar, R. Time-series models for
reliability evaluation of power systems including wind
energy. Microeletronic Reliability, v. 36, n. 9, p. 1253–
1261, 1996.
Castino, F.; Festa, R.; Ratto, C. F. Stochastic modelling of
Wind velocities time series. Journal of Wind
Engineering and Industrial Aerodynamics, v. 74–76, p.
141–151, 1998.
CCEE. Virtual Library. Available in:
<https://www.ccee.org.br/portal/faces/acesso_rapido_
header_publico_nao_logado/biblioteca_virtual?palavra
chave=Conjunto de arquivos para
cálculo&_afrLoop=870851647413887&_adf.ctrl-
state=9ll9uhpyu_27#!%40%40%3F_afrLoop%3D870
851647413887%26palavrachave%3DConjunto%2Bde
%2Barquivos%2Bpara%2Bc%25C3%25A1lculo%26_
adf.ctrl-state%3D9ll9uhpyu_31>. Access in: 8 dec.
2017.
Iversen, E. et al. Short-term probabilistic forecasting of
wind speed using stochastic differential equations.
International Journal of Forecasting, v. 32, n. 3, p. 981–
990, 2016.
Jung, J.; Broadwater, R. Current status and future advances
for Wind speed and power forecasting. Renewable and
Sustainable Energy Reviews, v. 31, p. 762–777, 2014.
Landry, M. et al. Probabilistic gradient boosting machines
for GEFCom2014 wind forecasting. International
Journal of Forecasting, v. 32, n. 3, p. 1061–1066, 2016.
Leite Da Silva, A. M.; Melo, A. C. G.; Cunha, S. H. F.
Frequency and duration method for reliability
evaluation of large-scale hydrothermal generating
systems. Generation Transmission and Distribution,
IEE Proceedings C, v. 138, n. 1, p. 94–102, 1991.
Manwell, J. F.; Mcgowan, J. G.; Rogers, A. L. Wind Energy
Explained: Theory, Design and Application. [s.l: s.n.].
Oliveira, F. L. C.; Souza, R. C.; Marcato, A. L. M. A time
series model for building scenarios trees applied to
stochastic optimisation. International Journal of
Electrical Power and Energy Systems, v. 67, p. 315–
323, 2015.
ONS. Histórico da Operação - Geração de Energia
Available: <http://www.ons.org.br/Paginas/resultados-
da-operacao/historico-da-
operacao/geracao_energia.aspxx>
Papaefthymiou, G.; Klöckl, B. MCMC for wind power
simulation. IEEE Transactions on Energy Conversion,
v. 23, n. 1, p. 234–240, 2008.
Pinson, P. Wind energy: forecasting challenges for its
operational management. Statistical Science, v. 28, n. 4,
p. 564–585, 2013.
Saha, S. et al. NCEP Climate Forecast System Version 2
(CFSv2) Selected Hourly Time-Series Products.
Available: <https://doi.org/10.5065/D6N877VB>.
Access in: 3 mar. 2017.
Suomalainen, K. et al. Correlation analysis on wind and
hydro resources with electricity demand and prices in
New Zealand. Applied Energy, v. 137, p. 445–462,
2015.
Zhang, Y.; Wang, J.; Wang, X. Review on probabilistic
forecasting of wind power generation. Renewable and
Sustainable Energy Reviews2, v. 32, p. 255–270, 2014.
Improvements in the Current Brazil’s Energy Dispatch Optimization: Load Forecast and Wind Power
405