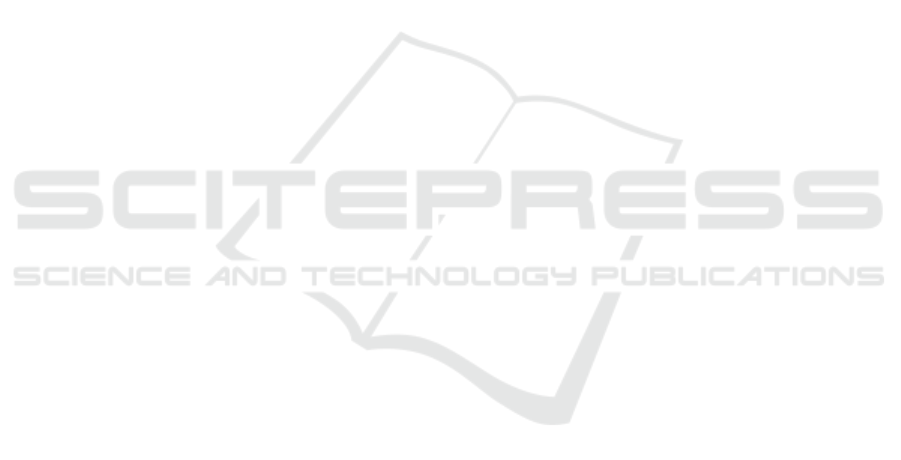
his dialectic method of inquiry, which is the founda-
tion of the modern scientific method. This is why we
found his name appropriate for a database designed
for image and video forensic.
SOCRatES is a publicly available database in-
tended for source digital camera identification on
smartphones. In other fields, several databases are
merged together to have a wider pool of data. This is
done in particular for developing and benchmarking
of deep-learning based techniques that require thou-
sands of images and are the trend at the moment.
SOCRatES can be used alone or in combination with
other image or video databases in order to widen the
data pool. Also, its challenging data samples, make it
very suitable as testing set.
In this paper the SOCRatES database is described
and baseline performances are obtained by testing two
well-known techniques based on the Sensor Pattern
Noise computation. The latter is a technique to iden-
tify, given a picture, its source digital camera. In par-
ticular, this technique can distinguish devices of the
same make and model.
Another important feature of SOCRatES, is the
presence of both images and videos captured with
each device. This allows the study of source cam-
era recognition on strongly compressed videos, which
is still an open issue, as for the study of asymmetric
comparison between videos and still images.
SOCRatES is made freely available to other re-
searchers for scientific purposes at the following
URL: http://socrates.eurecom.fr/.
REFERENCES
C¸ eliktutan, O., Sankur, B., and Avcibas, I. (2008). Blind
identification of source cell-phone model. IEEE
Trans. Information Forensics and Security, 3(3):553–
566.
Choi, K. S., Lam, E. Y., and Wong, K. K. (2006). Source
camera identification using footprints from lens aber-
ration. In Digital Photography II, volume 6069, page
60690J. International Society for Optics and Photon-
ics.
Chuang, W.-H., Su, H., and Wu, M. (2011). Exploring
compression effects for improved source camera iden-
tification using strongly compressed video. In Im-
age Processing (ICIP), 2011 18th IEEE International
Conference on, pages 1953–1956. IEEE.
Deng, Z., Gijsenij, A., and Zhang, J. (2011). Source cam-
era identification using auto-white balance approxi-
mation. In Computer Vision (ICCV), 2011 IEEE In-
ternational Conference on, pages 57–64. IEEE.
Farinella, G. M., Giuffrida, M. V., Digiacomo, V., and Bat-
tiato, S. (2015). On blind source camera identifica-
tion. In International Conference on Advanced Con-
cepts for Intelligent Vision Systems, pages 464–473.
Springer.
Galdi, C., Nappi, M., and Dugelay, J.-L. (2016). Multi-
modal authentication on smartphones: Combining iris
and sensor recognition for a double check of user iden-
tity. Pattern Recognition Letters, 82:144–153.
Galdi, C., Nappi, M., Dugelay, J.-L., and Yu, Y. (2018).
Exploring new authentication protocols for sensitive
data protection on smartphones. IEEE Communica-
tions Magazine, 56(1):136–142.
Geradts, Z. J., Bijhold, J., Kieft, M., Kurosawa, K., Kuroki,
K., and Saitoh, N. (2001). Methods for identification
of images acquired with digital cameras. In Enabling
technologies for law enforcement and security, vol-
ume 4232, pages 505–513. International Society for
Optics and Photonics.
Gloe, T. and B
¨
ohme, R. (2010). The’dresden image
database’for benchmarking digital image forensics. In
Proceedings of the 2010 ACM Symposium on Applied
Computing, pages 1584–1590. ACM.
Gloe, T., Pfennig, S., and Kirchner, M. (2012). Unex-
pected artefacts in prnu-based camera identification:
a’dresden image database’case-study. In Proceedings
of the on Multimedia and security, pages 109–114.
ACM.
Lanh, T. V., Chong, K., Emmanuel, S., and Kankanhalli,
M. S. (2007). A survey on digital camera image foren-
sic methods. In 2007 IEEE International Conference
on Multimedia and Expo, pages 16–19.
Li, C.-T. (2009). Source camera identification using enah-
nced sensor pattern noise. In Image Processing
(ICIP), 2009 16th IEEE International Conference on,
pages 1509–1512. IEEE.
Lin, X. and Li, C.-T. (2016). Enhancing sensor pattern noise
via filtering distortion removal. IEEE Signal Process-
ing Letters, 23(3):381–385.
Lukas, J., Fridrich, J., and Goljan, M. (2006). Digital cam-
era identification from sensor pattern noise. IEEE
Transactions on Information Forensics and Security,
1(2):205–214.
Marra, F., Gragnaniello, D., and Verdoliva, L. (2018). On
the vulnerability of deep learning to adversarial at-
tacks for camera model identification. Signal Process-
ing: Image Communication, 65:240–248.
Marra, F., Poggi, G., Sansone, C., and Verdoliva, L. (2017).
A study of co-occurrence based local features for cam-
era model identification. Multimedia Tools and Appli-
cations, 76(4):4765–4781.
Redi, J. A., Taktak, W., and Dugelay, J.-L. (2011). Digital
image forensics: a booklet for beginners. Multimedia
Tools and Applications, 51(1):133–162.
Shullani, D., Fontani, M., Iuliani, M., Al Shaya, O., and
Piva, A. (2017). Vision: a video and image dataset for
source identification. EURASIP Journal on Informa-
tion Security, 2017(1):15.
Van, L. T., Emmanuel, S., and Kankanhalli, M. S. (2007).
Identifying source cell phone using chromatic aberra-
tion. In Multimedia and Expo, 2007 IEEE Interna-
tional Conference on, pages 883–886. IEEE.
SOCRatES: A Database of Realistic Data for SOurce Camera REcognition on Smartphones
655