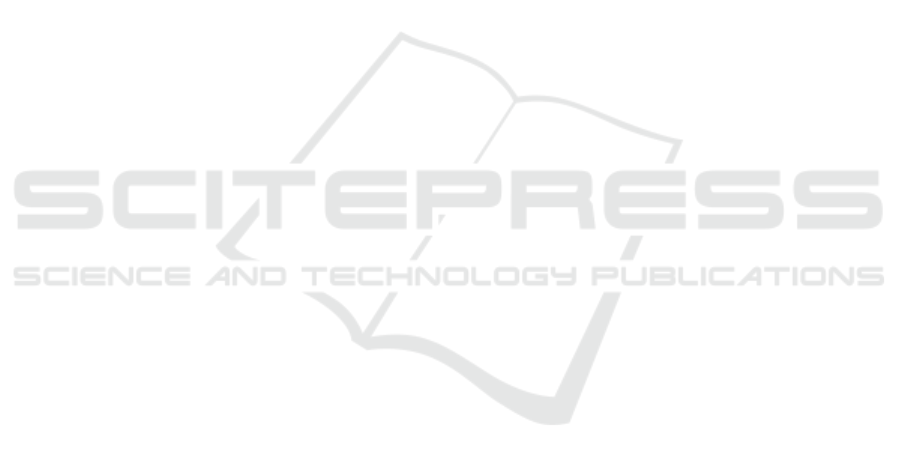
cially remarkable. Although the εDGA has potential
to find the solution superior to the skilled engineer in
terms of the best score, the its score averagely almost
equivalent and at worst inferior in the
pl03
problems.
6 CONCLUSION
We addressed the segment assignment in shield tun-
neling as a constrained combinatorial optimization
problem. This paper proposed the εICPSO and de-
monstrated its effectiveness to segment assignment
problems. The experimental results showed its poten-
tial to reduce construction costs as compared with the
conventional method. In all the test problems, the pro-
posed method outperformed all the comparative met-
hods. In the future, we will make more experiments
using three-dimensional simulator for more accurate
evaluation of the proposed method.
REFERENCES
Bonyadi, M., Li, X., and Michalewicz, Z. (2013). A hy-
brid particle swarm with velocity mutation for con-
straint optimization problems. In Proceedings of the
15th annual conference on Genetic and evolutionary
computation, pages 1–8. ACM.
Eberhart, R. C. and Shi, Y. (2000). Comparing inertia weig-
hts and constriction factors in particle swarm optimi-
zation. In
Evolutionary Computation, 2000. Procee-
dings of the 2000 Congress on, volume 1, pages 84–
88. IEEE.
Engelbrecht, A. P. (2014). Fitness function evaluations: A
fair stopping condition? In Swarm Intelligence (SIS),
2014 IEEE Symposium on , pages 1–8. IEEE.
Glover, F. W. and Kochenberger, G. A. (2006). Handbook
of metaheuristics, volume 57. Springer Science & Bu-
siness Media.
Goldberg, D. (1989). Genetic algorithms in search, optimi-
zation and machine learning. Addison-Wesley, Rea-
ding, MA.
Goldberg, D. E. and Deb, K. (1991). A comparative analysis
of selection schemes used in genetic algorithms. In
Foundations of genetic algorithms, volume 1, pages
69–93. Elsevier.
Hasanipanah, M., Noorian-Bidgoli, M., Armaghani, D. J.,
and Khamesi, H. (2016). Feasibility of pso-ann model
for predicting surface settlement caused by tunneling.
Engineering with Computers, 32(4):705–715.
Hassanien, A. E. and Emary, E. (2018). Swarm intelligence:
principles, advances, and applications . CRC Press.
Ihara, K., Kato, S., Nakaya, T., and Ogi, T. (2018). Con-
strained GA based segment assignment in shield tun-
neling to minimize the amount of excavated soil. In
2018 IEEE 7th Global Conference on Consumer Elec-
tronics (GCCE), pages 229–230. IEEE.
Japan Society of Civil Engineers (2007). Standard speci-
fications for tunneling-2006: shield tunnels. Tunnel
Engineering Committee.
Kennedy, J. (2011). Particle swarm optimization. In Ency-
clopedia of machine learning, pages 760–766. Sprin-
ger.
Kennedy, J. and Eberhart, R. (1995). Particle swarm optimi-
zation. In IEEE International Conference on Neural
Networks, pages 1942–1948. IEEE.
Koyama, Y. (2003). Present status and technology of shield
tunneling method in japan. Tunnelling and Under-
ground Space Technology, 18(2-3):145–159.
Larra
˜
naga, P. and Lozano, J. A. (2002). Estimation of distri-
bution algorithms: A new tool for evolutionary com-
putation, volume 2. Springer Science & Business Me-
dia.
Maidl, B., Herrenknecht, M., Maidl, U., and Wehrmeyer, G.
(2013). Mechanised shield tunnelling. John Wiley &
Sons.
Miller, B. L., Goldberg, D. E., et al. (1995). Genetic algo-
rithms, tournament selection, and the effects of noise.
Complex systems, 9(3):193–212.
Pampara, G., Franken, N., and Engelbrecht, A. P. (2005).
Combining particle swarm optimisation with angle
modulation to solve binary problems. In Evolutionary
Computation, 2005. The 2005 IEEE Congress on, vo-
lume 1, pages 89–96. IEEE.
Soares, J., Ghazvini, M. A. F., Silva, M., and Vale,
Z. (2016). Multi-dimensional signaling method for
population-based metaheuristics: Solving the large-
scale scheduling problem in smart grids. Swarm and
Evolutionary Computation, 29:13–32.
Storn, R. and Price, K. (1997). Differential evolution–a
simple and efficient heuristic for global optimization
over continuous spaces. Journal of global optimiza-
tion, 11(4):341–359.
Strasser, S., Goodman, R., Sheppard, J., and Butcher, S.
(2016). A new discrete particle swarm optimization
algorithm. In Proceedings of the Genetic and Evo-
lutionary Computation Conference (GECCO), pages
53–60. ACM.
Suwansawat, S. and Einstein, H. H. (2006). Artificial neural
networks for predicting the maximum surface settle-
ment caused by epb shield tunneling. Tunnelling and
underground space technology, 21(2):133–150.
Suzuki, A. (2016). 2016 annual report of NILIM: Pro-
ductivity improvement in infrastrucure development
process using i-construction. http://www.nilim.go.jp/
english/annual/annual2016/ar2016e.html. Accessed:
2018-10-23.
Syswerda, G. (1989). Uniform crossover in genetic al-
gorithms. In Proceedings of the third international
conference on Genetic algorithms, pages 2–9. Morgan
Kaufmann Publishers.
Takahama, T. and Sakai, S. (2005). Constrained optimiza-
tion by ε constrained particle swarm optimizer with
ε-level control. In Soft computing as transdisciplinary
science and technology, pages 1019–1029. Springer.
Takahama, T. and Sakai, S. (2006). Constrained optimi-
zation by the ε constrained differential evolution with
A PSO based Approach to Assign Segments for Reducing Excavated Soil in Shield Tunneling
335