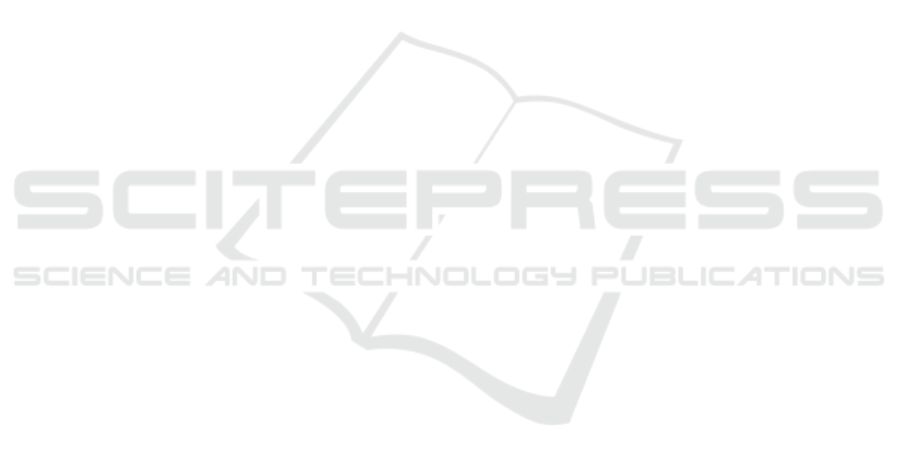
Our study develops an analytical model to deter-
mine an optimal policy level in a Cournot oligopoly
structure for both C&T and RPS. We extend the
model of Tanaka and Chen (2012) by considering the
optimal policy level (i.e., the rate of emission cap
and the rate of RPS). All sectors may then strategi-
cally determine output quantities to maximize prof-
its. We then apply the model to analyze a relation-
ship between market equilibria and energy and envi-
ronmental policy as C&T and RPS. Thus, the analyt-
ical model is used to generate contestable hypothe-
ses, while the numerical experiment gives us a more
meaningful and intuitive interpretation of the results.
We highlight the effect on electricity prices, social
welfare, and CO
2
emissions under C&T and RPS.
The following findings are provided in this pa-
per. Under C&T, the electricity price influences re-
newable energy generation. Electricity prices are high
because of low levels of non-renewable energy gener-
ation. The social surplus of C&T’s electricity market
becomes relatively large. C&T directly controls CO
2
emissions. Under RPS, renewable energy generation
decreases along with non-renewable energy genera-
tion. Electricity prices are low due to the high level of
renewable energy generation. RPS does not control
CO
2
emissions comparatively because non-renewable
energy generation also increases when renewable en-
ergy generation increases.
The remaining part of this paper is organized as
follows: Section 2 introduces one single-level model
and two bi-level models. After laying out our numer-
ical data in Section 3, we use the proposed model to
conduct experiments. Section 4 contains concluding
remarks along with suggestions for future research.
2 MODEL
In this study, we assume that there are one renewable
energy sector and two non-renewable energy sectors
possessing thermal power in the electricity industry.
We adopt a complementary approach to the mod-
els’ interaction between a deregulated electricity in-
dustry and a policymaker by assuming that the poli-
cymaker’s objective is to maximize social welfare in-
clusive of damages. In order to explore the variation
of outcomes, we allow for the following market set-
tings:
Benchmark Setting (BM). This benchmark
setting has a policymaker operating all power
plants in order to maximize social welfare,
considering damage from emissions. This
setup results in a single-level program.
C&T. The C&T is a market-based scheme for
reducing the emission of CO
2
effectively in a
region. Policymaker imposes upper-limit of
the emissions, that is, “cap” on power pro-
ducers. The power producers then trade a
difference between the upper-limit and actual
emissions in the market of the emission per-
mit. If actual emission for a power producer
is fewer (more) than upper-limit, the producer
might be able to sell (buy) the emission per-
mit. Energy sectors’ decisions at the lower
level are made by price-taking renewable and
non-renewable energy sectors that take the
rate of emission cap as given and maximize
their profits inclusive of emission revenues
or costs, the shadow price of the emission
cap constraint. At the upper level, the policy
maker decides the emission cap percentage in
order maximize social welfare constrained by
the lower level.
RPS. The RPS scheme is one for encourag-
ing power producers to supply a certain min-
imum share of their electricity from renew-
able energy sources. In countries or regions
where the RPS scheme is introduced there is
usually secondary market for REC. If the pro-
ducers can not meet the RPS target, in order
to meet the target they might be able to pur-
chase the certificates in the REC market. En-
ergy sectors’ decisions at the lower level are
made by renewable energy and non-renewable
energy sectors that take the RPS percentage
target as given and maximize their profits in-
clusive of REC revenues or costs determined
by the shadow price of the RPS constraint.
Each sector is dominant and behaves in Cournot
fashion (i.e., it is able to influence the electricity
price). Since we will examine three settings, we de-
note ·
∗
, ·
†
, and ·
⋄
as the optimal values for the decision
variables under BM, C&T, and RPS, respectively.
2.1 Notation
The parameters, and variables adopted in this study
are:
Parameters.
a: Intercept of the linear inverse demand function
(U.S. Dollars/MWh)
b: Slope of the linear inverse demand function
(U.S. Dollars/MWh
2
)
c
1
: Cost of non-renewable energy production 1
(U.S. Dollars/MWh)
ICORES 2019 - 8th International Conference on Operations Research and Enterprise Systems
430