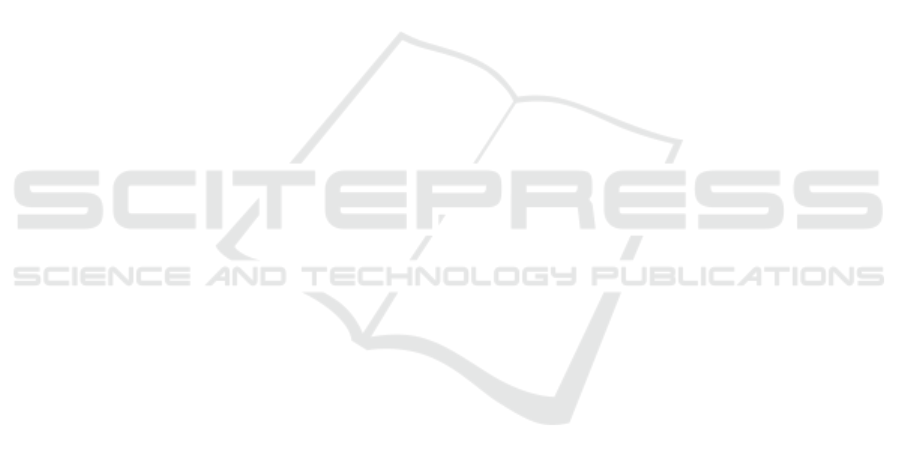
Gupta, A., Kuppili, P., Akella, A., and Barford, P. (2009).
An empirical study of malware evolution. In 2009
First International Communication Systems and Net-
works and Workshops, pages 1–10.
Hajmasan, G., Mondoc, A., and Cre, O. (2017). Dynamic
behavior evaluation for malware detection. In 2017
5th International Symposium on Digital Forensic and
Security (ISDFS), pages 1–6.
Hassen, M., Carvalho, M. M., and Chan, P. K. (2017). Mal-
ware classification using static analysis based features.
In 2017 IEEE Symposium Series on Computational
Intelligence (SSCI), pages 1–7.
Karam, C. and Kamluk, V. (2015). Blockchainware - de-
centralized malware on the blockchain. In Black Hat
ASIA.
Kilgallon, S., Rosa, L. D. L., and Cavazos, J. (2017). Im-
proving the effectiveness and efficiency of dynamic
malware analysis with machine learning. In 2017 Re-
silience Week (RWS), pages 30–36.
Kirat, Jiyong, and Stoecklin (2018). Deeplocker concealing
targeted attacks with ai locksmithing.
Kolosnjaji, B., Demontis, A., Biggio, B., Maiorca, D., Gi-
acinto, G., Eckert, C., and Roli, F. (2018). Adversarial
malware binaries: Evading deep learning for malware
detection in executables. CoRR, abs/1803.04173.
Lim, H., Yamaguchi, Y., Shimada, H., and Takakura, H.
(2015). Malware classification method based on se-
quence of traffic flow. In 2015 International Con-
ference on Information Systems Security and Privacy
(ICISSP), pages 1–8.
M. Chess, D. and R. White, S. (2000). An undetectable
computer virus. In Proceedings of Virus Bulletin Con-
ference.
Magnusardottir, A. (2018). Fileless ransomware: How it
works & how to stop it? White Paper.
Martinelli, F., Mercaldo, F., Saracino, A., and Visaggio,
C. A. (2016). I find your behavior disturbing: Static
and dynamic app behavioral analysis for detection of
android malware. In 2016 14th Annual Conference on
Privacy, Security and Trust (PST), pages 129–136.
Miller, C. and Valasek, C. (2015). Remote exploitation of
an unaltered passenger vehicle. White Paper.
Moubarak, J., Chamoun, M., and Filiol, E. (2018). Devel-
oping a k-ary malware using blockchain. In NOMS
2018 - 2018 IEEE/IFIP Network Operations and
Management Symposium, pages 1–4.
Naeem, H., Guo, B., and Naeem, M. R. (2018). A light-
weight malware static visual analysis for iot infras-
tructure. In 2018 International Conference on Artifi-
cial Intelligence and Big Data (ICAIBD), pages 240–
244.
Narayanan, A., Yang, L., Chen, L., and Jinliang, L.
(2016). Adaptive and scalable android malware de-
tection through online learning. In 2016 International
Joint Conference on Neural Networks (IJCNN), pages
2484–2491.
OSahn, D., Kural, O. E., Akleylek, S., and Kilic¸, E. (2018).
New results on permission based static analysis for an-
droid malware. In 2018 6th International Symposium
on Digital Forensic and Security (ISDFS), pages 1–4.
Paola, A. D., Gaglio, S., Re, G. L., and Morana, M. (2018).
A hybrid system for malware detection on big data. In
IEEE INFOCOM 2018 - IEEE Conference on Com-
puter Communications Workshops (INFOCOM WK-
SHPS), pages 45–50.
Ponemon Institute, Research Report (2017). The 2017 state
of endpoint security risk.
Prokofiev, A. O., Smirnova, Y. S., and Surov, V. A. (2018).
A method to detect internet of things botnets. In
2018 IEEE Conference of Russian Young Researchers
in Electrical and Electronic Engineering (EIConRus),
pages 105–108.
Raff, E., Barker, J., Sylvester, J., Brandon, R., Catanzaro,
B., and Nicholas, C. K. (2017). Malware detection by
eating a whole exe. CoRR, abs/1710.09435.
Rigaki, M. and Garcia, S. (2018). Bringing a gan to a knife-
fight: Adapting malware communication to avoid de-
tection. In 2018 IEEE Security and Privacy Work-
shops (SPW), pages 70–75.
Selcuk, A. A., Orhan, F., and Batur, B. (2017). Undecidable
problems in malware analysis. In 2017 12th Inter-
national Conference for Internet Technology and Se-
cured Transactions (ICITST), pages 494–497.
Shirataki, S. and Yamaguchi, S. (2017). A study on in-
terpretability of decision of machine learning. In
2017 IEEE International Conference on Big Data (Big
Data), pages 4830–4831.
Soliman, S. W., Sobh, M. A., and Bahaa-Eldin, A. M.
(2017). Taxonomy of malware analysis in the iot. In
2017 12th International Conference on Computer En-
gineering and Systems (ICCES), pages 519–529.
Su, J., Vasconcellos, V. D., Prasad, S., Daniele, S., Feng,
Y., and Sakurai, K. (2018). Lightweight classifica-
tion of iot malware based on image recognition. In
2018 IEEE 42nd Annual Computer Software and Ap-
plications Conference (COMPSAC), volume 01, pages
664–669.
Su, M.-Y. and Fung, K.-T. (2016). Detection of android
malware by static analysis on permissions and sensi-
tive functions. In 2016 Eighth International Confer-
ence on Ubiquitous and Future Networks (ICUFN),
pages 873–875.
Yang, W., Kong, D., Xie, T., and Gunter, C. A. (2017). Mal-
ware detection in adversarial settings: Exploiting fea-
ture evolutions and confusions in android apps. In AC-
SAC.
Yeo, M., Koo, Y., Yoon, Y., Hwang, T., Ryu, J., Song,
J., and Park, C. (2018). Flow-based malware de-
tection using convolutional neural network. In 2018
International Conference on Information Networking
(ICOIN), pages 910–913.
Zhang-Kennedy, L., Assal, H., Rocheleau, J., Mohamed, R.,
Baig, K., and Chiasson, S. (2018). The aftermath of a
crypto-ransomware attack at a large academic institu-
tion. In Proceedings of the 27th USENIX Conference
on Security Symposium, SEC’18, pages 1061–1078,
Berkeley, CA, USA. USENIX Association.
The Curious Case of Machine Learning in Malware Detection
535