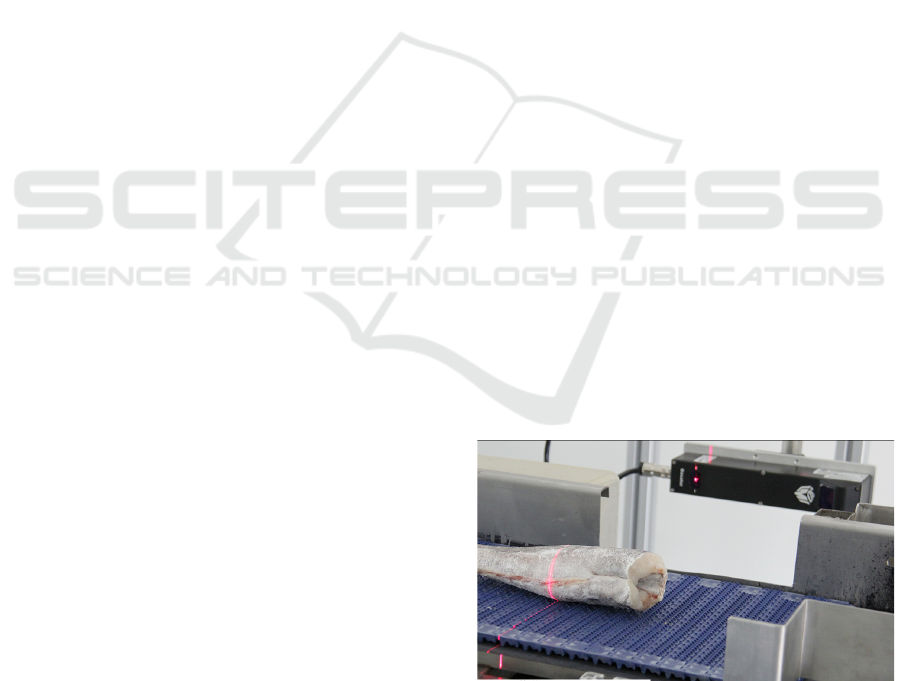
mension while at the same time inspects it for "de-
fects" (e.g. if it is the "head bone" present and visi-
ble?).
2 PREVIOUS WORK
The computer vision technologies applied to the in-
dustry cover a great variety of solutions for image
acquisition like: visible/near-infrared, computed to-
mography, X-ray (Kelkar et al., 2015), magnetic res-
onance imaging, laser scanner (Kelkar et al., 2011) in
2D and 3D space (Mathiassen et al., 2011). The es-
timation of weight using computer vision techniques
implies the determination of density. Magnetic res-
onance imaging, computed tomography, laser scan-
ners,and even X-ray are imaging modalities that have
been frequently employed for this kind of task.
An overview of some of the AOI-based developed
solutions for "food inspection", in specific, focused
to compute (estimate) parameters, such as the weight
and density are presented to follow.
In (Viazzi et al., 2015), computer vision tech-
niques are used to estimate the weight of a complete
fish. The study uses regression techniques to find the
best model that estimates weight using features ex-
tracted from images. The computer vision algorithm
includes four steps. First, detect the region that en-
closes the fish. Second, the fish is segmented using
the "Otsu adaptive thresholding" algorithm. Next, it
is removed the tail fin using a shape analysis method.
Finally, some features like height, length, and area are
extracted with and without including the tail fin. As
conclusions, authors state both for: 1) the area pa-
rameter is sufficient and more robust to estimate the
weight of a fish; and 2) the tail fin negatively influ-
ences the weight estimation. Another approach tries
to establish a relation between the projected area of
sushi shrimp, captured using an RGB camera, and its
corresponding weight (Poonnoy and Chum-in, 2012).
In (Mortensen et al., 2016) for the use case of
broiler chickens weight estimation, it is described a
system composed of a weighing platform (used to
give a reference weight) and a RGB-D sensor (Mi-
crosoft kinect), where authors developed an algorithm
including the following steps: (1) segment the broil-
ers using watershed and region growing techniques
in a previously filtered gray-scale image (combining
a Gaussian filter and morphological operators); (2)
the extraction of features like projected area, width,
perimeter, radius eccentricity, volume and others from
each segmented broiler; and (3) weight prediction of
each broiler based on the extracted features using ar-
tificial neural networks. A convex hull and numeri-
cal integration are used to obtain an approximated 3D
model of the broilers.
In (Adamczak et al., 2018) it is used a white light
(3D scanning) technology to determine the weight of
the chicken breasts.
Eggs volume estimation based on computer vi-
sion techniques was explored by (Soltani et al., 2015)
with the objective to extract the eggs major and minor
diameters. Two methods for estimating the volume
were tested: 1) a Mathematical model based on Pap-
pus theorem; and 2) Artificial Neural Networks. As
result, it was observed that the developed mathemati-
cal model achieved better results..
3 AUTOMATIC VISION SYSTEM
In order to support the development of the proposed
method, we built an experimental prototype (a stand
alone machine) comprising: 1) a conveyor belt that
moves at a speed of 0.5 m/s, which can transport
fishes of different sizes and volumes; 2) a precision
scale; 3) two "Entry-Level 3D Laser Line Profile Sen-
sors" - Gocators 2150 (Technologies, 2018) in an op-
posite layout placed at a distance of one meter (Fig-
ure 1). The Gocator 2150 is a 3D smart sensor / cam-
era, an all-in-one solution that lets factories to im-
prove efficiency in product validation; and 4) an in-
dustrial PC.
With this, each fish is weighted using the scale
and its total weight (T
w
) is recorded. Then, the fish
is scanned using the Gocators sensors, resulting two
line profiles (corresponding to both sides of the fish;
top and bottom). The computed profiles are then inte-
grated generating a precise 3D fish model using a fine
tuning developed computer vision algorithm.
Figure 1: Hardware prototype of the acquisition module,
including sensors that emits and capture lines of laser that
are projected in the fish body over the conveyor belt.
Taking as input the generated 3D model, we estimate
the individual weight of a voxel (V
w
) dividing the total
weigh of the fish (T
W
) by the total number of the com-
ICPRAM 2019 - 8th International Conference on Pattern Recognition Applications and Methods
708