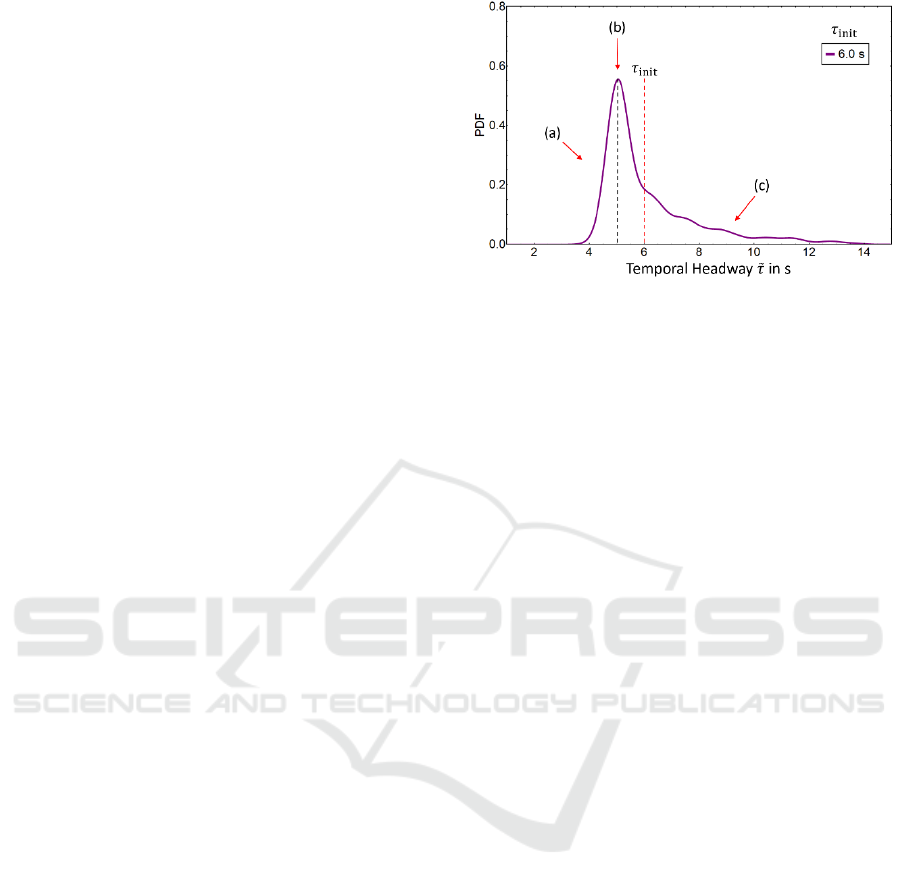
increase. Due to the slight model fluctuations, sharp
peaks are resulting (figure 2), i.e. the temporal
headway is not changing significantly within the
range from
to
. This behaviour does not seem
to be realistic at all. In Table 1 mean, variance and
skewness are listed to enable a quantitative point of
view.
Compared to other works, where often only
averaged empirical data over many cars is shown, our
dataset consists of single vehicle information. The
measurement took place on a bright day without any
precipitation in April 2018 on a straight road in
Duisburg, Germany exhibiting a speed limit of 50
km/h. Only vehicle speeds in flowing traffic were
taken into account. In order to make sure that the
driving behaviour of individual cars was not affected
by the measurement, the cameras were placed hidden.
Two road markings with a distance of 20 meters in
between served as an aid to determine speeds of
passing vehicles. Due to the associated averaging
process of the vehicle speeds within a range of 20
meters, an error of ±2 km/h should be taken into
account. This corresponds to the bin width of the
histogram shown in figure 3.
In order to obtain a more realistic behaviour
within the framework of a microscopic simulation, a
randomised free flow parameter
was chosen for
different initial temporal headways
(figure 4).
When comparing the different distributions of , it is
noticeable that they are getting wider (increasing
variance) with increasing
(table 2). However, the
skewness is continuously decreasing. This is due to
the safe space gap
of the Kerner-Klenov model,
which represents the lower limit of the gap between
two consecutive vehicles. If the gap is already small,
there are many more possibilities for an increase. The
bigger it becomes, the more balanced options there
are for the underlying agent leading to a more
symmetric shape of the related distribution. The mean
value of the temporal headway stays very close to
the initial value
.
It is interesting to see, that the results of
subsection 2.1 and subsection 2.3 differ not only in
variance, but also in their skewness (compare table 1
to table 2). Whereas a negative skewness is obtained
for a constant
, the skewness becomes positive
for a free flow parameter following a normal
distribution. A comparision of the mean values
shows, that both are systematically smaller than the
underlying initial value
.
For the following qualitative discussion, we now
turn to figure 5 showing a single distribution of from
figure 4 (
= 6 s). It seems that the mode of the
Figure 5: Qualitative discussion on the PDF for
following a normal distribution. As an example, the
distribution for the simulation of
= 6 s has been chosen
(see figure 4), which is representative of all. Compared to
the PDF’s heavy tail towards larger temp. headways (c),
the distribution has a steep slope towards shorter (a). The
mode (b, black dashed line) is systematically smaller than
the underlying initial temp. headway
(red dashed line).
distribution is systematically smaller than the
underlying initial temporal headway
, i.e. most of
the cars within the analysed ensemble tend to close
the gap to their preceding vehicle. The distribution
has got a steep slope on its left-hand side. It looks as
if there is a lower limit relating to short temporal
headways for a given
, whereas the heavy tail’s
range towards larger temporal headways cannot be
determined clearly.
4 CONCLUSIONS
We found out that the typical probability density
function (PDF) describing the temporal headway
development do not have a symmetrical shape. A
heavy tail behaviour towards larger temporal
headways occurs, if the free flow parameter
of
the underlying Kerner-Klenov model follows a
normal distribution. This shape seems to be
qualitatively independent of the initial temporal
headway
. Providing this information to an
automated vehicle helps to find the most efficient
driving strategy for merging into the ongoing traffic.
We would like to compare our results to real
traffic temporal headway distributions. With regard to
the described scenario, we are developing a stationary
infrared sensor system including multiple units to
detect a large number of passing vehicles. Using the
generated data helps us to adjust the model’s
underlying functions and parameters in order to
describe real traffic more reliable. Furthermore, this
research is going to be shared within the “MEC-
ICAART 2019 - 11th International Conference on Agents and Artificial Intelligence
242