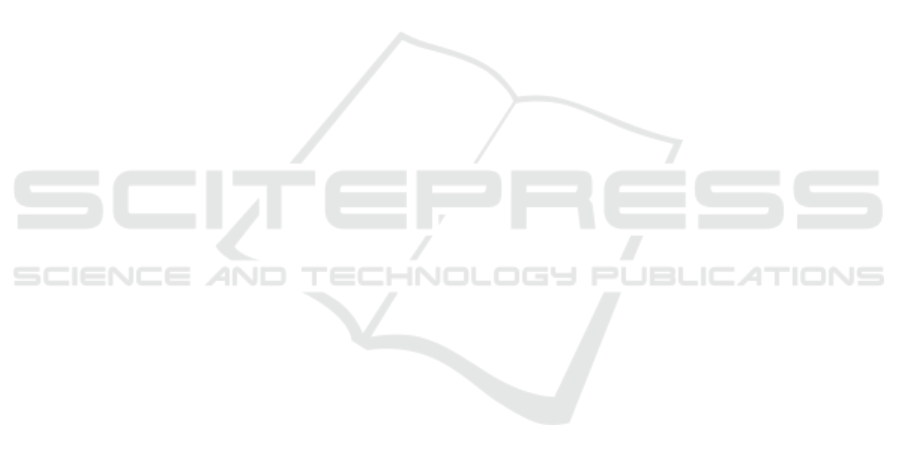
This includes strategies such as fixed price strategies,
pre-announced increasing prices (price commitment
strategy), or reimbursements for decreasing prices
(price-matching strategy).
The second stream seeks to mitigate strategic
waiting behavior by limiting the product availability
in order to address a customer’s concern of not being
able to purchase the product in the future (cf. run-
out). Similarly, sellers can also counteract strategic
consumer behavior by strategically announcing (or
hiding) partial inventory information to highlight the
product’s scarcity.
As typically observed in practice, in our model,
we allow for free price adjustments. On average, how-
ever, this leads to comparatively stable price paths.
These reference prices can be estimated by strate-
gic customers, cf. Wu et al. (2015). Further mod-
els focusing on reference price effects are studied by
Popescu, Wu (2007), Wu, Wu (2015), or Chenavaz,
Paraschiv (2018).
Finally, the key question is how (i) reference
prices, (ii) consumer’s price anticipations, and (iii) a
firm’s pricing strategy affect each other. Further, the
mutual dependencies will have to be determined by
buyers and sellers based on their partially observable
asymmetric (market) data. In addition, the complex
interplay of their mutual beliefs is further complicated
when multiple seller compete for the same market, cf.,
e.g., Levin et al. (2009), Liu, Zhang (2013).
1.2 Contribution
In the literature, for simplicity, mostly so-called my-
opic customers are considered. They simply arrive
and decide; they do not return to check prices again
and they do not anticipate future prices.
In our model, we consider the following two
sources of strategic customer behavior. First, we al-
low that customers return with a certain probability
in case they refuse to buy, i.e., if their willingness-
to-pay (WTP) does not exceed the current offer price.
To reflect planning uncertainty over time, we model a
customer’s future WTP as a random variable. Second,
we allow a certain share of customers to anticipate fu-
ture offer prices (based on the current offer price and
predetermined reference prices) as well as their indi-
vidual future WTP in order to check whether there is
an incentive to delay their purchase decision - even if
their current WTP exceeds the offer price. This allows
customers to optimize their consumer surplus.
We study a finite horizon model with limited ini-
tial inventory (i.e., products cannot be reproduced or
reordered). While in the literature demand is often
assumed to be of a special highly stylized functional
form, we allow for fairly general demand definitions.
In our model, demand is characterized by randomized
evolutions of individual WTP, which are not observ-
able for the seller. To this end, demand is allowed to
generally depend on time, the current offer price, and
reference prices.
The main contributions of this paper are the
following: We (i) present a demand model which
is based on individual reservation prices, reference
prices, and expected consumer surpluses, (ii) we com-
pute optimized feedback pricing strategies, (iii) we
study the impact of different strategic behaviors com-
pared to myopic settings, and (iv) we propose a Hid-
den Markov version of our model.
This paper is organized as follows. In Section 2,
we describe our model setup and define strategic cus-
tomer behaviors. In Section 3, we present our solution
approach and illustrate its results using different nu-
merical examples. In Section 4, we study a relaxed
version of our model with partially observable states.
Conclusions are summarized in the final Section 5.
2 MODEL DESCRIPTION
We consider the situation in which a firm wants to sell
a finite number of goods (e.g., airline tickets, event
tickets, accommodation services) over a certain time
frame. We assume a monopoly situation. Further, a
certain ratio of customers acts strategically, i.e., they
(i) repeatedly track prices and wait for acceptable of-
fers and (ii) they are forward-looking, i.e., they com-
pare their current consumer surplus with expected fu-
ture consumer surpluses.
We assume that the time horizon T is finite. We
assume that products cannot be reproduced or re-
ordered. If a sale takes place, shipping costs c have
to be paid, c ≥ 0. A sale of one item at price a, a ≥ 0,
leads to a profit of a −c. Discounting is also included
in the model. For the length of one period, we use the
discount factor δ, 0 ≤ δ ≤ 1.
2.1 Individual Buying Behavior
We consider a discrete time model with T periods.
We assume that consumers have individual (random)
reservation prices denoted by R
t
for periods (t,t + 1),
t = 0,1,...,T − 1. The reservation prices particularly
account for a customer’s planning uncertainty to be
able to benefit from the product (e.g., an airline ticket
or an event ticket in time T ). In this context, the av-
erage planning uncertainty typically decreases over
time as the time horizon T gets closer.
ICORES 2019 - 8th International Conference on Operations Research and Enterprise Systems
180