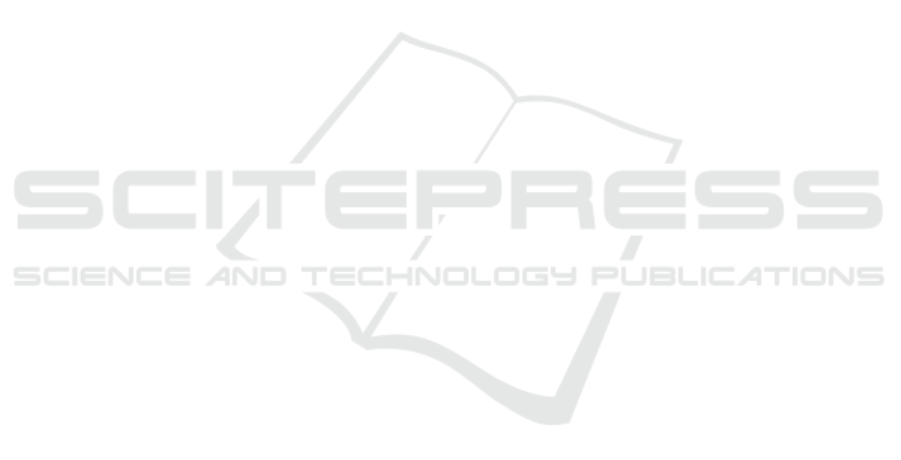
solution is applied to the RGB-D sequences in order
to preprocess sets of consecutive frames before apply-
ing the 3DMM fitting. Preliminary results have been
reported that show the reconstruction errors between
the 3D models derived after 3DMM fitting and the
corresponding ground truth scans. It clearly emerges
as the proposed framework provides superior results
with respect to a landmark-based solution.
As further step in the direction of proposing our
system for face rehabilitation purposes, we are col-
lecting a face dataset that includes RGB-D sequences
of the face captured by a Kinect camera, and the
corresponding high-resolution scans acquired with a
3dMD scanner.
REFERENCES
Amberg, B., Romdhani, S., and Vetter, T. (2007). Optimal
step nonrigid ICP algorithms for surface registration.
In IEEE Conf. on Computer Vision and Pattern Recog-
nition (CVPR), pages 1–8.
Anasosalu, P. K., Thomas, D., and Sugimoto, A. (2013).
Compact and accurate 3-D face modeling using an
rgb-d camera: Let’s open the door to 3-D video con-
ference. In IEEE Int. Conf. on Computer Vision Work-
shops, pages 67–74.
Bas, A., Smith, W. A., Bolkart, T., and Wuhrer, S. (2016).
Fitting a 3D morphable model to edges: A comparison
between hard and soft correspondences. In Asian Con-
ference on Computer Vision, pages 377–391. Springer.
Berretti, S., Pala, P., and Del Bimbo, A. (2014). Face recog-
nition by super-resolved 3D models from consumer
depth cameras. IEEE Trans. on Information Forensics
and Security, 9(9):1436–1449.
Blanz, V. and Vetter, T. (1999). A morphable model for the
synthesis of 3D faces. In ACM Conf. on Computer
Graphics and Interactive Techniques, pages 187–194.
Blanz, V. and Vetter, T. (2003). Face recognition based on
fitting a 3D morphable model. IEEE Trans. on Pattern
Analysis and Machine Intelligence, 25(9):1063–1074.
Bondi, E., Pala, P., Berretti, S., and Del Bimbo, A.
(2016). Reconstructing high-resolution face models
from kinect depth sequences. IEEE Trans. on Infor-
mation Forensics and Security, 11(12):2843–2853.
Booth, J., Roussos, A., Ververas, E., Antonakos, E.,
Ploumpis, S., Panagakis, Y., and Zafeiriou, S. (2018).
3D reconstruction of ’in-the-wild’ faces in images and
videos. IEEE Trans. on Pattern Analysis and Machine
Intelligence, 40(11):2638 – 2652.
Booth, J., Roussos, A., Zafeiriou, S., Ponniahand, A., and
Dunaway, D. (2016). A 3D morphable model learnt
from 10,000 faces. In IEEE Conf. on Computer Vision
and Pattern Recognition, pages 5543–5552.
Chui, H. and Rangarajan, A. (2000). A feature registration
framework using mixture models. In IEEE Workshop
on Mathematical Methods in Biomedical Image Anal-
ysis, pages 190–197.
Cleveland, W. S. (1979). Robust locally weighted regres-
sion and smoothing scatterplots. Journal of the Amer-
ican Statistical Association, 74(368):829–836.
Ferrari, C., Lisanti, G., Berretti, S., and Del Bimbo, A.
(2015). Dictionary learning based 3D morphable
model construction for face recognition with varying
expression and pose. In Int. Conf. on 3D Vision.
Ferrari, C., Lisanti, G., Berretti, S., and Del Bimbo,
A. (2017). A dictionary learning-based 3D mor-
phable shape model. IEEE Trans. on Multimedia,
19(12):2666–2679.
Hernandez, M., Choi, J., and Medioni, G. (2012). Laser
scan quality 3-D face modeling using a low-cost depth
camera. In European Signal Processing Conf. (EU-
SIPCO), pages 1995–1999. IEEE.
Izadi, S., Kim, D., Hilliges, O., Molyneaux, D., Newcombe,
R., Kohli, P., Shotton, J., Hodges, S., Freeman, D.,
Davison, A., and Fitzgibbon, A. (2011). Kinectfusion:
Real-time 3D reconstruction and interaction using a
moving depth camera. In ACM Symposium on User
Interface Software and Technology, pages 559–568.
Kazemi, V., Keskin, C., Taylor, J., Kohli, P., and Izadi, S.
(2014). Real-time face reconstruction from a single
depth image. In IEEE Int. Conf. on 3D Vision, vol-
ume 1, pages 369–376.
Mairal, J., Bach, F., Ponce, J., and Sapiro, G. (2009). Online
dictionary learning for sparse coding. In Int. Conf. on
Machine Learning, pages 689–696.
Myronenko, A. and Song, X. (2010). Point set registration:
Coherent point drift. IEEE Trans. on Pattern Analysis
and Machine Intelligence, 32(12):2262–2275.
Newcombe, R. A., Izadi, S., Hilliges, O., Molyneaux, D.,
Kim, D., Davison, A. J., Kohi, P., Shotton, J., Hodges,
S., and Fitzgibbon, A. (2011). Kinectfusion: Real-
time dense surface mapping and tracking. In IEEE
Int. Symposium on Mixed and Augmented Reality.
Patel, A. and Smith, W. A. P. (2009). 3D morphable face
models revisited. In IEEE Conf. on Computer Vision
and Pattern Recognition, pages 1327–1334.
Paysan, P., Knothe, R., Amberg, B., Romdhani, S., and
Vetter, T. (2009). A 3D face model for pose and il-
lumination invariant face recognition. In IEEE Int.
Conf. on Advanced Video and Signal Based Surveil-
lance (AVSS), pages 296–301.
Phillips, P. J., Flynn, P. J., Scruggs, T., Bowyer, K. W.,
Chang, J., Hoffman, K., Marques, J., Min, J., and
Worek, W. (2005). Overview of the face recognition
grand challenge. In IEEE Work. on Face Recognition
Grand Challenge Experiments, pages 947–954.
Yin, L., Wei, X., Sun, Y., Wang, J., and Rosato, M. J.
(2006). A 3D facial expression database for facial be-
havior research. In IEEE Int. Conf. on Automatic Face
and Gesture Recognition, pages 211–216.
Zollh
¨
ofer, M., Martinek, M., Greiner, G., Stamminger, M.,
and S
¨
ußmuth, J. (2011). Automatic reconstruction of
personalized avatars from 3D face scans. Computer
Animation and Virtual Worlds, 22(2-3):195–202.
Zollh
¨
ofer, M., Nießner, M., Izadi, S., Rehmann, C., Zach,
C., Fisher, M., Wu, C., Fitzgibbon, A., Loop, C.,
Theobalt, C., and Stamminger, M. (2014). Real-
time non-rigid reconstruction using an RGB-D cam-
era. ACM Trans. on Graphics, 33(4):156:1–156:12.
3D Face Reconstruction from RGB-D Data by Morphable Model to Point Cloud Dense Fitting
735