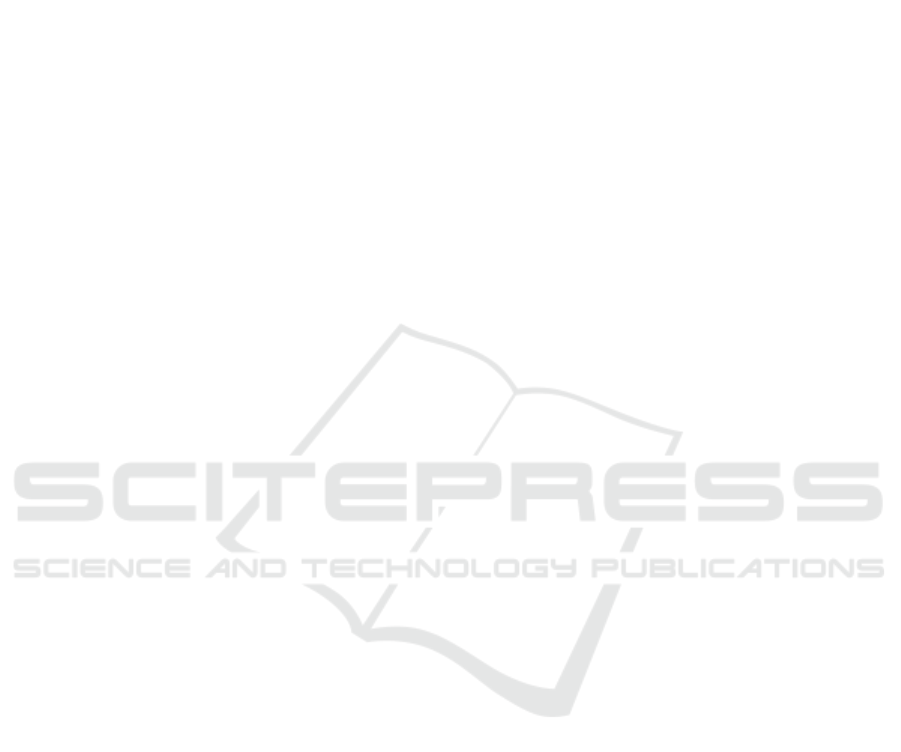
Predicting Group Convergence in Egocentric Videos
Jyoti Nigam and Renu M. Rameshan
Indian Institute of Technology, Mandi, Himachal Pradesh, India
Keywords:
Ego-vision, Group Convergence, Gaze Mask, Strongly Connected Graph.
Abstract:
In this work, our aim is to find the group dynamics in social gathering videos that are captured from the first-
person perspective. The complexity of the task is high as only one sensor (wearable camera) is present to sense
all the N agents. An additional complexity arises as the first-person who is part of the group is not visible in the
video. In particular, we are interested in identifying the group (with certain number of agents) convergence.
We generate a dataset named EgoConvergingGroup to evaluate our proposed method. The proposed method
predicts the group convergence in 90 to 250 number of frames, which is much ahead of the actual convergence.
1 INTRODUCTION AND
RELATED WORK
Over the past few years wearable cameras have be-
come very common and analysis of egocentric videos
has become a well recognized research area. We fo-
cus on egocentric videos involving social interactions.
Our aim is to analyze and predict group formation in
such videos. Activities like people converging to form
a group, diverging from a group, standing together,
etc (Bhargava and Chaudhuri, 2014) are classified as
social interactions. Recognizing such group interac-
tions in a social gathering where first-person is also
a part of that group is a challenging and interesting
problem which also has various applications.
A prime application is in mining the interesting
moments and group activities from the videos cap-
tured in the entire day. The information about the
different types of interactions in a social gathering
can aid in various other applications like: (i) find-
ing connections among the agents, (ii) identifying
who is meeting whom and recognition of isolated per-
son, and (iii) finding center of attraction in the group
along with interacting individuals. The above men-
tioned applications have a significant role in surveil-
lance, safety and social behavior analysis (Bhargava
and Chaudhuri, 2014).
In (Alletto et al., 2015), the authors detected sta-
tionary (already formed) groups in ego-vision scenar-
ios. People in the scene are tracked through out the
video and their head pose and 3D locations are esti-
mated. In (Bhargava and Chaudhuri, 2014), the group
dynamics is captured by a single surveillance cam-
era which is fixed at a certain height and sees all the
agents throughout which can give complete trajectory
information of the group.
In egocentric videos due to the absence of a third-
person static camera we cannot get the global infor-
mation i.e. all agents are not being captured from the
camera as the first-person is not visible in the videos.
In the proposed work, we consider a social gathering
scenario in which the agents are moving towards each
other to form a group. We are particularly interested
in finding the criterion for group convergence.
To handle the above mentioned challenges, we
adopted the technique from (Lin et al., 2004), which
addresses the problem of predicting group conver-
gence of mobile autonomous agents. Their solution
is based on local distributed control strategy where
each agent has a sensor which senses the neighboring
agents to form the interaction graph. These graphs are
analyzed to predict the group convergence.
We solve the problem of predicting group conver-
gence in egocentric videos where we have only one
sensor that is the first-person camera. We extract all
the information needed for creating the graph from
the video assuming all agents are visible throughout
the video. The single sensor (camera) makes this
problem challenging.
The contributions of the proposed work are
• Group dynamics of agents in egocentric videos
are analyzed with only partial information about
the group. The meaning of partial information is
that out of N agents only N − 1 agents are seen
throughout the video.
• We show that the gaze information of an agent can
Nigam, J. and Rameshan, R.
Predicting Group Convergence in Egocentric Videos.
DOI: 10.5220/0007556207730777
In Proceedings of the 8th International Conference on Pattern Recognition Applications and Methods (ICPRAM 2019), pages 773-777
ISBN: 978-989-758-351-3
Copyright
c
2019 by SCITEPRESS – Science and Technology Publications, Lda. All rights reserved
773