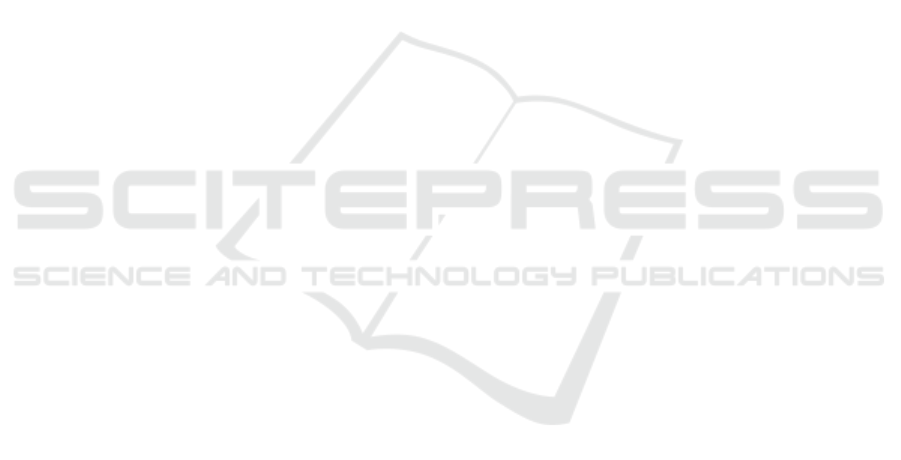
but when related information is missing or little, their
performance will drop.
Jin et al. (2016) improved news verification by
mining conflicting viewpoints in microblogs.
Long et al. (2017) proved that speaker profiles
such as party affiliation, speaker title, location and
credit history provided valuable information to vali-
date the credibility of news articles.
Farajtabar et al. (2017) proposed a multi-stage in-
tervention framework that tackled fake news in social
networks by combining reinforcement learning with a
point process network activity model.
Volkova et al. (2017) found social interaction fea-
tures were more informative for finer-grained sepa-
ration between four types of suspicious news (satire,
hoaxes, clickbait and propaganda) compared to syn-
tax and grammar features.
Tacchini et al. (2017) classified Facebook posts as
hoaxes or non-hoaxes with high accuracy on the basis
of the users who liked them.
6.4 Hybrid Approaches
Hybrid approaches combine the advantage of linguis-
tic models and network models, which intuitively out-
perform either of them.
Ruchansky et al. (2017) proposed a model that
combined the text of an article, the user response it
receives, and the source users promoting it for a more
accurate and automated prediction.
As far as we know, no other hybrid approaches are
available and fact-checking is absent from all existing
models. We also survey on commercial fake news de-
tectors and find that the majority of them take only
linguistic features into consideration.
7 CONCLUSION
In this paper, we evaluate a fake news detector Fake-
box on adversarial attacks, including fact-distortion,
subject-object exchange and cause confounding at-
tacks. Experiments show that our attack subverts the
model significantly. We believe that similar models
based solely on linguistic characteristics will perform
much less effectively in the real world and are espe-
cially vulnerable to tampering attacks. This kind of
attack is much more subtle, since it doesn’t change
the overall writing style of news articles and thus has
the potential to evade similarity detection. We argue
that multi-source fact comparing and checking must
be integrated into fake news detection models to truly
detect misinformation.
At the same time we find false positive rate rises
when it comes to either under-written real articles
or certain topics around which there is supposed to
be more fake news. The potential of misclassifying
under-written yet real news will hurt amateur news
writers’ enthusiasm. Thus we further suggest using
fact-checking as a helpful supplement so as to smooth
the negative effect of false positive judges.
One possible way to collect fact about news events
is to use a crowdsourced knowledge graph, which is
dynamically updated by local and well-informed peo-
ple. The timely information collected can then be
used to compare to that extracted from news articles
and help generate a label of veracity.
Our future work includes building a visualized in-
terface for news knowledge graph crowdsourcing, so
as to make work as easy as possible for non-experts
and stop fact-tampering fake news on early stage. We
also want to look at the issue of fake news propaga-
tion from a different angle, i.e., putting it in a social
context and examining human factors in order to bet-
ter understand the problem.
REFERENCES
Bourgonje, P., Schneider, J. M., and Rehm, G. (2017). From
clickbait to fake news detection: An approach based
on detecting the stance of headlines to articles. In pro-
ceedings of the 2017 EMNLP.
Chatzimilioudis, G., Konstantinidis, A., Laoudias, C., and
ZeinalipourYazti, D. (2012). Crowdsourcing with
smartphones. In IEEE Internet Comp., 36-44.
Chen, Y., Conroy, N. J., and Rubin, V. L. (2015). Mis-
leading online content: Recognizing clickbait as false
news. In Proceedings of the 2017 EMNLP.
Conroy, N. J., Rubin, V. L., and Chen, Y. (2015). Automatic
deception detection: methods for finding fake news.
In Proceedings of the 78th ASIS&T Annual Meeting.
Dumitrache, A., Aroyo, L., and Welty, C. (2018). Crowd-
sourcing ground truth for medical relation extraction.
In ACM Transactions on Interactive Intelligent Sys-
tems 8, 2.
Edell, A. (2018). I trained fake news detection ai with
>95% accuracy, and almost went crazy.
Farajtabar, M., Yang, J., Ye, X., Xu, H., Trivedi, R., Khalil,
E., Li, S., Song, L., and Zha, H. (2017). Fake news
mitigation via point process based intervention. In
arXiv:1703.07823 [cs.LG].
Granik, M. and Mesyura, V. (2017). Fake news detection
using naive bayes classifier. In IEEE First Ukraine
Conference on Electrical and Computer Engineering
(UKRCON).
Horne, B. D. and Adali, S. (2017). This just in: Fake news
packs a lot in title, uses simpler, repetitive content in
text body, more similar to satire than real news. In 2nd
Fake News Detection via NLP is Vulnerable to Adversarial Attacks
799