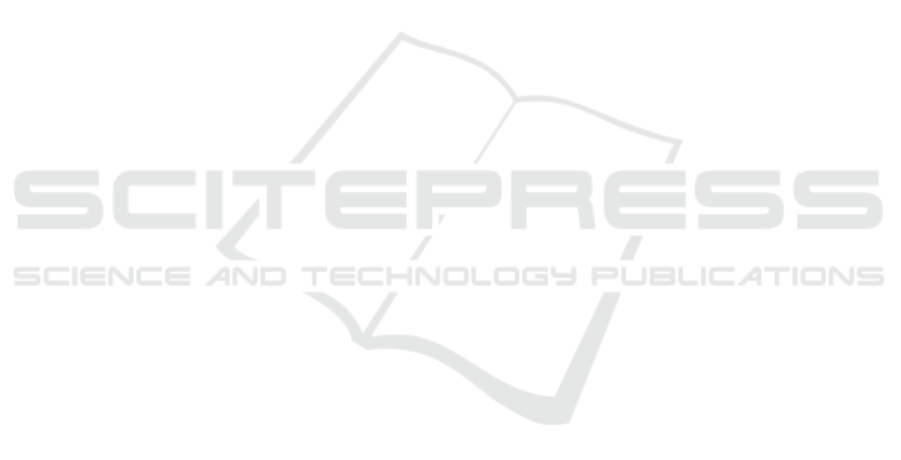
tifact/ minor cuts and scrapes etc. While, the results
for major knuckle and finger nail are much more con-
sistent. One most important point to mention is that
our network has just been trained over 500 random
images and performs much better than other existing
state-of-the-art ROI extraction algorithm.
5 CONCLUSION AND FUTURE
SCOPE
In this paper, we have proposed a end-to-end network
for extracting ROIs from any finger knuckle image.
To best of our knowledge, this is the first holistic
architecture proposed so far, segmenting three ROIs
namely major finger knuckle, minor finger knuckle
and finger nail in the image. We have explored the
possibility to extract a complete information from
a finger dorsal region so that it can enumerate the
recognition performance of state-of-art FKI recog-
nition systems. This method provides rotation and
translation error free results and capable of localizing
regions in case of challenging finger kuckle images.
The proposed holistic ROI segmentation network has
been trained with around 500 images and produces
very satisfactory and consistent results. Experimen-
tal results on two FKI data-sets show that our method
outperforms state-of-the-art finger knuckle segmenta-
tion approaches in terms of segmentation accuracy.
This work on holistic finger knuckle segmentation,
opens up the vast opportunities in the field of multi-
biometric authentication systems. In future work, we
will try to compute the recognition performance over
these extracted ROI’s.
REFERENCES
(2006-13). Polyu contact-less finger knuckle im-
age database. http://www4.comp.polyu.edu.hk/∼csa
jaykr/fn1.htm.
(2009). Finger-knuckle-print polyu. https://www4.comp.
polyu.edu.hk/∼biometrics/FKP.htm.
Bera, A., Bhattacharjee, D., and Nasipuri, M. (2014). Hand
biometrics in digital forensics. In Computational In-
telligence in Digital Forensics: Forensic Investigation
and Applications, pages 145–163. Springer.
Chlaoua, R., Meraoumia, A., Aiadi, K. E., and Korichi, M.
(2018). Deep learning for finger-knuckle-print iden-
tification system based on pcanet and svm classifier.
Evolving Systems, pages 1–12.
Girshick, R., Donahue, J., Darrell, T., and Malik, J. (2014).
Rich feature hierarchies for accurate object detection
and semantic segmentation. pages 580–587.
He, K., Zhang, X., Ren, S., and Sun, J. (2016). Deep resid-
ual learning for image recognition. pages 770–778.
Jain, A. K., Ross, A., and Prabhakar, S. (2004). An in-
troduction to biometric recognition. IEEE Transac-
tions on Circuits and Systems for Video Technology,
14(1):4–20.
Jaswal, G., Kaul, A., and Nath, R. (2016). Knuckle print
biometrics and fusion schemes–overview, challenges,
and solutions. ACM Computing Surveys (CSUR),
49(2):34.
Jaswal, G., Nigam, A., and Nath, R. (2017a). Deepknuckle:
revealing the human identity. Multimedia Tools and
Applications, pages 1–30.
Jaswal, G., Nigam, A., and Nath, R. (2017b). Finger
knuckle image based personal authentication using
deepmatching. pages 1–8.
Kumar, A. (2012). Can we use minor finger knuckle images
to identify humans? In Biometrics: Theory, Applica-
tions and Systems (BTAS), 2012 IEEE Fifth Interna-
tional Conference on, pages 55–60. IEEE.
Kumar, A. (2014). Importance of being unique from fin-
ger dorsal patterns: Exploring minor finger knuckle
patterns in verifying human identities. IEEE
Transactions on Information Forensics and Security,
9(8):1288–1298.
Kumar, A. and Kwong, C. (2013). Towards contactless,
low-cost and accurate 3d fingerprint identification.
pages 3438–3443.
Kumar, A. and Prathyusha, K. V. (2009). Personal au-
thentication using hand vein triangulation and knuckle
shape. IEEE Transactions on Image processing,
18(9):2127–2136.
Kumar, A. and Ravikanth, C. (2009). Personal authentica-
tion using finger knuckle surface. IEEE Transactions
on Information Forensics and Security, 4(1):98–110.
Kumar, A. and Xu, Z. (2016). Personal identification us-
ing minor knuckle patterns from palm dorsal surface.
IEEE Transactions on Information Forensics and Se-
curity, 11(10):2338–2348.
Nigam, A., Tiwari, K., and Gupta, P. (2016). Multiple
texture information fusion for finger-knuckle-print au-
thentication system. Neurocomputing, 188:190–205.
Zhai, Y., Cao, H., Cao, L., Ma, H., Gan, J., Zeng, J., Pi-
uri, V., Scotti, F., Deng, W., Zhi, Y., et al. (2018).
A novel finger-knuckle-print recognition based on
batch-normalized cnn. In Chinese Conference on Bio-
metric Recognition, pages 11–21. Springer.
Zhang, L., Zhang, L., and Zhang, D. (2009). Finger-
knuckle-print: a new biometric identifier. In 16th
IEEE International Conference on Image Processing,
pages 1981–1984. IEEE.
Zhang, L., Zhang, L., Zhang, D., and Zhu, H. (2010). On-
line finger-knuckle-print verification for personal au-
thentication. Pattern recognition, 43(7):2560–2571.
HFDSegNet: Holistic and Generalized Finger Dorsal ROI Segmentation Network
793