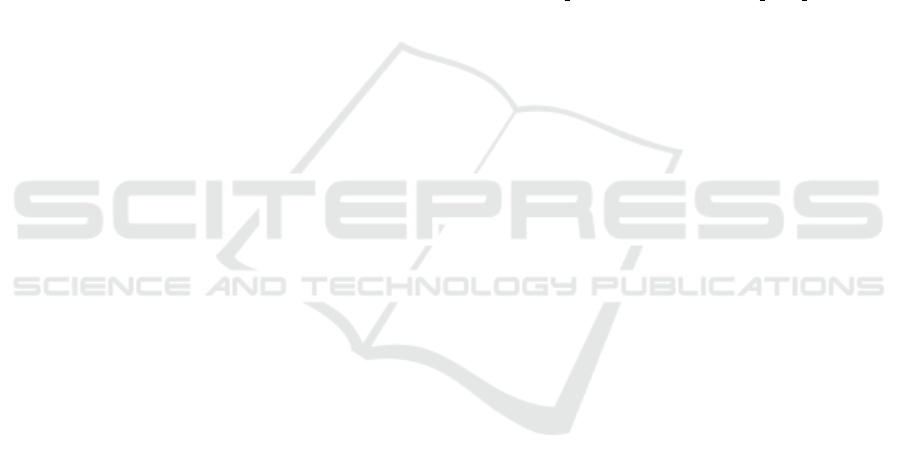
6 CONCLUSIONS
We have discussed several approaches to reduce re-
turns in the context of garment e-commerce. A few
promising approaches could not be followed up be-
cause they proved too costly to implement, such as
adding garment size tags only after delivery and sub-
sequent measurement to effectively remove manufac-
turing tolerances. One simple approach – providing
more detailed product-specific measurement tables –
is currently in evaluation. We shortly mentioned the
usefulness of qualitative size information and pre-
sented some preliminary results.
In the main part of our paper, we describe a
method to identify and remove highly predictive fea-
tures from large, mostly undocumented datasets to
improve the quality and stability of trained models
while also preventing overfitting. We demonstrate the
usefulness of this method by describing a rule set of
only eleven rules that predicts returns at good preci-
sion and recall on a large real-life dataset. To achieve
this, it was also necessary to modify the chosen learn-
ing algorithm RIPPER in a minor way to ensure it
always characterizes returns rather than non-returns.
The described rules show some intriguing patterns
which are currently investigated by our commercial
partner and some may prove to be generally useful.
In the future we hope to follow up on reconstruct-
ing precise body size from ordering information – ob-
serving that we already obtained reasonably precise
manufacturing size information – and finish our pre-
liminary investigations towards a final result.
ACKNOWLEDGEMENTS
This project was funded by the Austrian Research
Promotion Agency (FFG) and by the Austrian Fed-
eral Ministry for Transport, Innovation and Technol-
ogy (BMVIT) as project Think!First (859099)
REFERENCES
Cohen, W. (1995). Fast effective rule induction. In Pro-
ceedings of the Twelfth International Conference on
Machine Learning, San Francisco, CA, 1995, pages
115–123. Morgan Kaufmann.
Colsen, E. (2013). Using human and machine processing
in recommendation systems. In Human Computation
and Crowdsourcing: Works in Progress and Demon-
stration Abstracts, number 13-01 in CR.
Ghaffari, S. (2011). Will it fit? consumer decision mak-
ing in online shopping environments. Master’s the-
sis, School of Industrial Design, Georgia Institute for
Technology, USA.
Hagemann, H. (2015). Umweltrelevante Produktinfor-
mationen im E-Commerce: Chancen f
¨
ur nachhalti-
gen Konsum. Umweltbundesamt, D-06844 Dessau-
Roßlau, Germany.
Halbach, J., St
¨
uber, E., and Piepke, M. (2015). Nach-
haltigkeit im Online-Handel - Die Rolle von Aus-
gestaltung und Kommunikation. IFH Institut f
¨
u Han-
delsforschung GmbH.
Hofacker, L. and Langenberg, C. (2015). E-Commerce-
Markt
¨
Osterreich/Schweiz 2015. EHI Retail In-
stitute. https://www.ehi.org/deStudien/e-commerce-
markt-oesterreichschweiz-2015.
Knabl, W., K
¨
ob, M., Meszaros, G., Prenger, C., Rischanek,
U., Segal, D., and Weigl, A. (2015). retail - Magazin
f
¨
ur den
¨
osterreichischen Handel. Offizielles Medium
des Handelsverbandes, volume 04. Handelsverband.
https://www.handelsverband.at/fileadmin/content/
images publikationen/retail/Retail 2015 04.pdf.
Kristensen, K., Borum, N., Christensen, L., et al. (2013).
Towards a next generation universally accessible
online shopping-for-apparel system. In Human-
Computer Interaction: Users and Cotexts of Use, Vol-
ume 8006 of the series Lecture Notes in Computer Sci-
ence, pp. 418-427. Springer.
Lengauer, E., Koll, O., Kreuzer, M., Herry, M., and Sed-
lacek, N. (2015). eComTraf - Auswirkungen von E-
Commerce auf das Gesamtverkehrssystem. Austrian
Federal Ministry for Transport, Innovation and Tech-
nology (BMVIT). Study funded within the search pro-
gram Mobility for the Future (Mobilit
¨
at der Zukunft,
MdZ) by the Austrian Federal Ministry for Transport,
Innovation and Technology (BMVIT).
Seewald, A. (2007). Improving the effectiveness of mail-
ings by building a response model for inactive cus-
tomers. Technical Report 2007-01, Seewald So-
lutions, L
¨
archenstraße 1, A-4616 Weißkirchen a.d.
Traun, Austria.
Singh, K. (2015). Reducing Customer Returns in an Online
Marketplace. Dept. of Fashion Technology, National
Institute of Fashion Technology, Mumbai, India.
Toktay, L. (2003). Forecasting product returns. In Guide Jr.,
D. and Van Wassenhove, L., editor, Business Apects
of Closed-Loop Supply Chains, International Man-
agement Series, volume 2. Carnegie Bosch Institute.
Wernbacher, T., Pfeiffer, A., Denk, N., Platzer, M., Berger,
M., Seewald, A., Winter, T., and Miller, I. (2017).
Minimizing returns through gamification, persuasive
design principles & machine learning. In Poster pre-
sentation at the 11th European Conference on Games
Based Learning (ECGBL 2017), Oct. 5-6 2017, Graz,
Austria.
Willemsen, R., Abraham, J., and van Welle, R. (2016).
Global B2C E-commerce Report 2016. Ecommerce
Foundation, Raddhuisstraat 22, NL-1060 Amsterdam,
The Netherlands.
ICAART 2019 - 11th International Conference on Agents and Artificial Intelligence
808