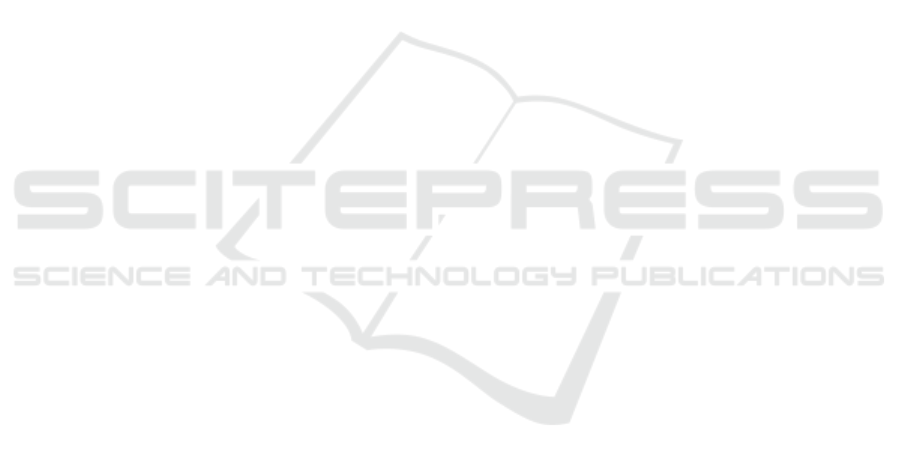
6 CONCLUSIONS
The considered neural network consists of the
oscillating mobile neurons that connect and
disconnect with respect to their entropies and the
entropy of the ensemble. The states of the neurons
are defined on the basis of the well-known Hodgkin-
Huxley model that defines the oscillations of the
neurons’ activity.
Such definition allows calculation of the entropy
of the neuron and the neuron’s ensemble using the
Klimontovich method that is widely used in
statistical physics.
The suggested approach contrasts with the
traditional methods, where the connections between
the neurons are governed by the external learning
procedures, and specifies the neurons’ connections
on the basis of the neurons’ internal properties.
Numerical simulations confirm feasibility of the
suggested model and demonstrate the required
properties of the entropy of separate neurons and of
the neurons’ ensembles. In particular, it was shown
that the entropy of the single neuron periodically
obtains the values greater than the values of the
entropy of this neuron acting in pair with the other
neuron. Following the suggested model, connection
and disconnection of the neurons is governed by this
inequality.
The suggested mechanism of assembling /
disassembling is equal to motion of the neurons
toward the other neurons or away from them,
respectively, and the information about the neurons’
entropies is transmitted via the glia.
REFERENCES
Apolloni, B., Bassis, S., Valerio, L., 2011. Training a
network of mobile neurons. In Proc. Int. Joint Conf.
on Neural Networks, San Jose, CA, 1683-1691.
Fausett, L., 1994. Fundamentals of Neural Networks:
Architectures, Algorithms and Applications. Upper
Saddle River, NJ, Prentice Hall.
Fregnac, Y., 2003. Hebbian cell assembles. In
Encyclopaedia of Cognitive Science, Nature
Publishing Group, 320-329.
Gerstner, W., Kistler, W., 2002. Mathematical
formulations of Hebbian learning. Biological
Cybernetics, 87, 404-415.
Haken, H., 2008. Brain Dynamics. An Introduction to
Models and Simulations. Springer-Verlag,
Berlin/Heidelberg, 2
nd
edition.
Hebb, D. O., 1949. The Organization of Behaviour: A
Neuropsycological Theory. Wiley & Sons, New York.
Izhikevich, E., 2003. Simple model of spiking neurons.
IEEE Trans. Neural Networks, 14, 1569-1572.
Izhikevich, E., 2007. Dynamical Systems in
Neuroschience: The Geometry of Excitability and
Bursting. The MIT Press, Cambridge, MA, London,
UK.
Kagan, E., Ben-Gal, I., 2015. Search and Foraging.
Individual Motion and Swarm Dynamics. CRC Press /
Chapman & Hall / Taylor & Francis, Boca Raton, FL,
Kagan, E., Rybalov, A., Ziv, H., 2016. Uninorm-based
neural network and its application for control of
mobile robots. In Proc. IEEE Int. Conf. Science of
Electrical Engineering, Eilat, Israel.
Kawahara, T., 1980. Coupled van der Pol oscillators – a
model of excitatory and inhibitory neural interactions.
Biological Cybernetics, 39(1), 37-43.
Klimontovich, Yu., 1987. Entropy evolution in self-
organization processes: H-theorem and S-theorem.
Physica, 142A, 390-404.
Klimontovich, Yu., 1991. Turbulent Motion and the
Structure of Chaos. Springer Science + Business
Media, Dordrecht.
Kuzmina, M., Manykin, E., Grichuk, E., 2014. Oscillatory
Neural Networks. De Gruyter, Berlin/Boston.
Leppanen, T., 2004 Computational Studies of Pattern
Formation in Turing Systems. Helsinki University of
Technology, Laboratory of Computational
Engineering, Report B40, Helsinki.
Mikhailov, A. 1990. Foundations of Synergetics I.
Distributed Active Systems. Springer-Verlag, Berlin,
2
nd
edition.
Rand, R., Holmes, P., 1980. Bifurcation of periodic
motions in two weakly coupled van der Pol oscillators.
Int. J. Non-Linear Mechanics, 15, 387–399.
Russell, S., Norvig, P., 2010. Artificial Intelligence. A
Modern Approach. Pearson Education, Upper Saddle
River, NJ, 3
rd
edition.
Sterratt, D., Graham, B., Gillies, A., Willshaw, D., 2011.
Principles of Computational Modelling in
Neuroscience. Cambridge University Press,
Cambridge, MA.
Turing, A., 1952. The chemical basis of morphogenesis.
Phil. Trans. Royal Soc. London, B, Biological
Sciences, 237(641), 37-72.
Vedenov, A., Ejov, A., Levchenko, E., 1987. Nonlinear
dynamical systems with memory and the functions of
neouron ensembles. In Gaponov-Grekhov A.,
Rabinovich, I (eds.) Nonlinear waves: Structures and
Bifurcations, Nauka, Moscow, 53-67 (in Russian).
Zaslavsky, G., 2007. Physics of Chaos in Hamiltonian
Systems. Imperial College Press, London, 2
nd
edition.
ICAART 2019 - 11th International Conference on Agents and Artificial Intelligence
266