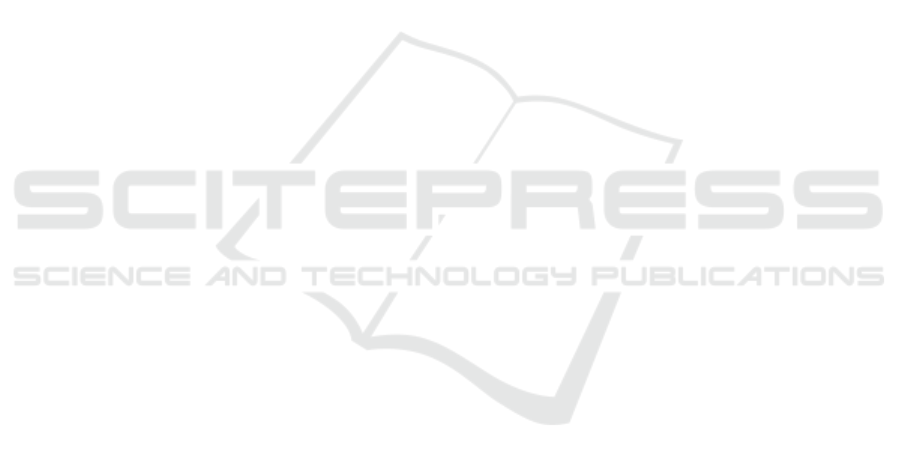
garding solution quality. In future work, it may also
be promising to further scrutinize the impact of the
communication topology as design parameter.
REFERENCES
Aggarwal, S. and Goswami, P. (2014). Implementation of
dejong function by various selection method and ana-
lyze their performance. IJRCCT, 3(6).
Ahrari, A. and Shariat-Panahi, M. (2015). An improved
evolution strategy with adaptive population size. Op-
timization, 64(12):2567–2586.
Anders, G., Hinrichs, C., Siefert, F., Behrmann, P., Reif,
W., and Sonnenschein, M. (2012). On the Influence
of Inter-Agent Variation on Multi-Agent Algorithms
Solving a Dynamic Task Allocation Problem under
Uncertainty. In Sixth IEEE International Conference
on Self-Adaptive and Self-Organizing Systems (SASO
2012), pages 29–38, Lyon, France. IEEE Computer
Society. (Best Paper Award).
B
¨
ack, T., Fogel, D. B., and Michalewicz, Z., editors (1997).
Handbook of Evolutionary Computation. IOP Pub-
lishing Ltd., Bristol, UK, UK, 1st edition.
Bremer, J. and Lehnhoff, S. (2016). A decentralized
PSO with decoder for scheduling distributed electric-
ity generation. In Squillero, G. and Burelli, P., editors,
Applications of Evolutionary Computation: 19th Eu-
ropean Conference EvoApplications (1), volume 9597
of Lecture Notes in Computer Science, pages 427–
442, Porto, Portugal. Springer.
Bremer, J. and Lehnhoff, S. (2017a). An agent-based ap-
proach to decentralized global optimization: Adapt-
ing cohda to coordinate descent. In van den Herik,
J., Rocha, A., and Filipe, J., editors, ICAART 2017
- Proceedings of the 9th International Conference on
Agents and Artificial Intelligence, volume 1, pages
129–136, Porto, Portugal. SciTePress, Science and
Technology Publications, Lda.
Bremer, J. and Lehnhoff, S. (2017b). Decentralized coali-
tion formation with agent-based combinatorial heuris-
tics. ADCAIJ: Advances in Distributed Computing
and Artificial Intelligence Journal, 6(3).
Bremer, J. and Lehnhoff, S. (2017c). Hybrid Multi-
ensemble Scheduling, pages 342–358. Springer Inter-
national Publishing, Cham.
Bremer, J. and Sonnenschein, M. (2014). Parallel tempering
for constrained many criteria optimization in dynamic
virtual power plants. In Computational Intelligence
Applications in Smart Grid (CIASG), 2014 IEEE Sym-
posium on, pages 1–8.
Brent, R. (1973). Algorithms for Minimization Without
Derivatives. Dover Books on Mathematics. Dover
Publications.
Brent, R. P. (1971). An algorithm with guaranteed conver-
gence for finding a zero of a function. Comput. J.,
14(4):422–425.
Colorni, A., Dorigo, M., Maniezzo, V., et al. (1991). Dis-
tributed optimization by ant colonies. In Proceedings
of the first European conference on artificial life, vol-
ume 142, pages 134–142. Paris, France.
Dekker, T. (1969). Finding a zero by means of successive
linear interpolation. Constructive aspects of the fun-
damental theorem of algebra, pages 37–51.
Dorigo, M. and St
¨
utzle, T. (2004). Ant Colony Optimiza-
tion. Bradford Company, Scituate, MA, USA.
Gano, S. E., Kim, H., and Brown II, D. E. (2006). Compar-
ison of three surrogate modeling techniques: Datas-
cape, kriging, and second order regression. In Pro-
ceedings of the 11th AIAA/ISSMO Multidisciplinary
Analysis and Optimization Conference, AIAA-2006-
7048, Portsmouth, Virginia.
Hansen, E. (1980). Global optimization using interval anal-
ysis – the multi-dimensional case. Numer. Math.,
34(3):247–270.
Hansen, N. (2006). The CMA evolution strategy: a compar-
ing review. In Lozano, J., Larranaga, P., Inza, I., and
Bengoetxea, E., editors, Towards a new evolutionary
computation. Advances on estimation of distribution
algorithms, pages 75–102. Springer.
Hansen, N. (2011). The CMA Evolution Strategy: A Tuto-
rial. Technical report.
Hansen, N. and Ostermeier, A. (2001). Completely deran-
domized self-adaptation in evolution strategies. Evol.
Comput., 9(2):159–195.
Hansen, P., Jaumard, B., and Lu, S.-H. (1992). Global op-
timization of univariate lipschitz functions ii: New al-
gorithms and computational comparison. Math. Pro-
gram., 55(3):273–292.
Hinrichs, C. and Sonnenschein, M. (2014). The Effects
of Variation on Solving a Combinatorial Optimiza-
tion Problem in Collaborative Multi-Agent Systems.
In Mller, J. P., Weyrich, M., and Bazzan, A. L., edi-
tors, Multiagent System Technologies, volume 8732 of
Lecture Notes in Computer Science, pages 170–187.
Springer International Publishing.
Hinrichs, C., Sonnenschein, M., and Lehnhoff, S. (2013).
Evaluation of a Self-Organizing Heuristic for Inter-
dependent Distributed Search Spaces. In Filipe, J.
and Fred, A. L. N., editors, International Conference
on Agents and Artificial Intelligence (ICAART 2013),
volume Volume 1 – Agents, pages 25–34. SciTePress.
Hinrichs, C., Vogel, U., and Sonnenschein, M. (2011). Ap-
proaching decentralized demand side management via
self-organizing agents. Workshop.
Horst, R. and Pardalos, P. M., editors (1995). Handbook of
Global Optimization. Kluwer Academic Publishers,
Dordrecht, Netherlands.
Jamil, M. and Yang, X. (2013). A literature survey of
benchmark functions for global optimization prob-
lems. CoRR, abs/1308.4008.
Kennedy, J. and Eberhart, R. (1995). Particle swarm op-
timization. In Neural Networks, 1995. Proceedings.,
IEEE International Conference on, volume 4, pages
1942–1948 vol.4. IEEE.
Kramer, O. (2010). A review of constraint-handling tech-
niques for evolution strategies. Appl. Comp. Intell.
Soft Comput., 2010:1–19.
ICAART 2019 - 11th International Conference on Agents and Artificial Intelligence
78