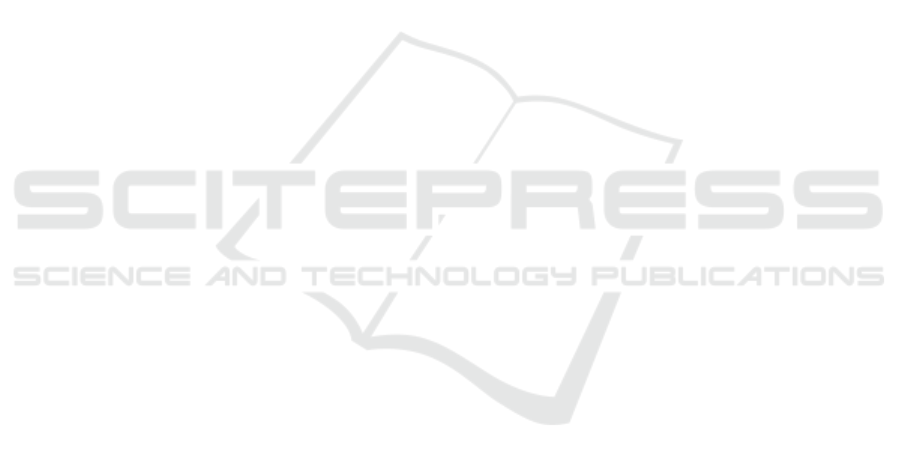
word, students must learn the word’s specific
character(s), associate the character(s) with its
meaning, and recall the word’s sound(s) in order to
communicate with the word. Connections between
character, meaning, and sound must be bi-directional
for successful use of the word in writing, reading, and
conversation. In contrast, learners of phonetic
European languages usually only need to construct
two-way mental connections to be able to write, read,
and converse. Native speakers of traditionally
phonetic languages struggle when learning Mandarin
because it is not possible to “spell” Chinese
characters and, often, there is no obvious association
between a character and its sound (i.e., it is not
possible to “sound it out”). Even native Chinese
speakers may blank when called upon to write the
character for a relatively common word due to this
lack of intuitive connections.
Warschauer and Healey (1998) argued that the
development of information technology provided
foreign language instructors and learners with new
possibilities for practicing language acquisition. For
instance, the popular language-learning app Duolingo
(2017) offers gamified, self-paced courses for native
English speakers to learn 27 languages (NPR/TED
Staff, 2014). As users progress through each lesson in
their course, the app uses their responses to develop
and verify translations of websites and articles on the
Internet. However, Duolingo did not offer a course in
Mandarin until 2017 (Hagiwara, 2017), likely due to
the complexities involved in teaching and learning
Chinese as a second language. The app ChineseSkill
follows a gamified format similar to that of Duolingo,
but focuses strictly on Mandarin (ChineseSkill Co.,
Ltd, 2017). Applications like these broaden the reach
of the Chinese language to learners who may have
otherwise been intimidated by the time and
commitment it requires to gain fluency.
Research has also shown that reading and writing
Chinese characters are two separate information
acquisition processes with different influencing
factors (Jiang, 2007). The use of Pinyin, a
Romanization system for Mandarin, helps to link
these processes by transforming characters into
phonetic words. Through Pinyin, applications like
Duolingo and ChineseSkill, as well as other digital
resources, can allow learners to read or submit the
phonetic versions of Chinese characters. Zhu et al.,
(2009) indicated that as a digital input method, Pinyin
can strengthen character recognition through the
consolidation of pronunciation capabilities. While
tablets and other touch devices may allow learners to
draw characters, Pinyin bridges the availability of
Chinese learning acquisition to broader digital
environments. Learners must still memorize
characters for the sake of recognition in reading, and
in order to connect the character and its Pinyin
equivalent, but producing characters and applying the
proper stroke order is no longer a necessity.
Despite an understanding that Chinese language
acquisition requires characters, meanings, sounds,
and often Pinyin, little research has been done on the
difficulties inherent to each specific component of the
process. Even less work has focused on how Chinese
language acquisition has adapted to the digital world.
As a Chinese language instructor at a major
institution in New England, the first author observed
that students typically begin word memorization by
practicing connections between sound and meaning,
considering the character/meaning connection as a
secondary task. Her observation was supported by
literature on Chinese language acquisition. Tan and
Perfetti (1999) suggested that phonology is an
obligatory component of word identification in
Chinese reading. Perfetti and Liu (2006) then
supported this idea, stating that phonology is
automatically activated in reading words, regardless
of whether activation occurs before or after the
moment of lexical access and regardless of whether it
is instrumental in retrieving the word’s meaning.
Essentially, Chinese characters activate
pronunciation, even when the reader’s goal is to
determine the character’s meaning.
Based on past research and considering the six
connections required for Chinese word acquisition, it
is possible to speculate that connections between
meaning and character are more difficult because
sound must also be accessed, even if unintentionally.
It becomes difficult to discern if and how these
components of word acquisition can be teased apart,
and whether providing particular types of connections
more frequently than others has the potential to
produce more robust learning.
As such, we conducted the present study as a
randomized controlled trial with three conditions to
compare the consequences of removing each bi-
directional connection pair involved in Chinese word
acquisition: sound focused practice, meaning focused
practice, and character focused practice. The present
study sought to answer the following research
questions:
1. Does student performance, as measured at post-
test, differ significantly when a learning
assignment for novel words lacks bi-directional
sound-meaning, meaning-character, or
character-sound connection pairs?
CSEDU 2019 - 11th International Conference on Computer Supported Education
322