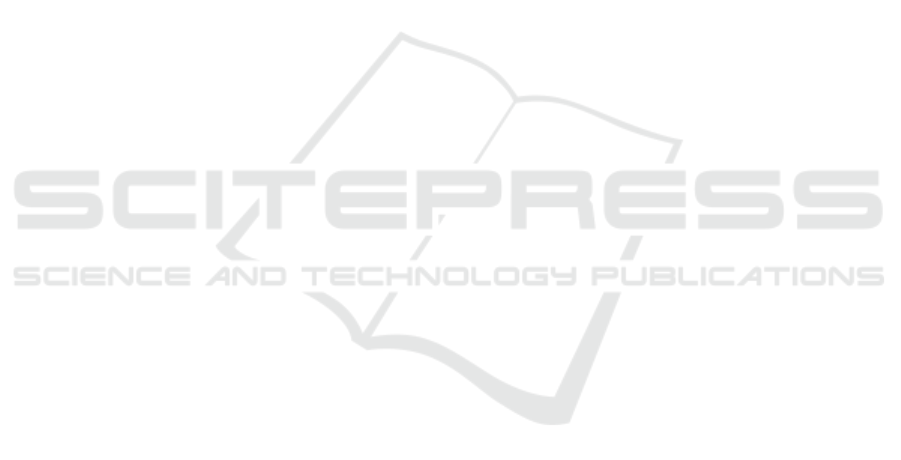
REFERENCES
Altman, N. S. (1992). An introduction to kernel and nearest-
neighbor nonparametric regression. The American
Statistician, 46(3):175–185.
Beeson, P. M., Higginson, K., and Rising, K. (2013).
Writing treatment for aphasia: A texting approach.
Journal of Speech, Language, and Hearing Research,
56(3):945–955.
Bentley, P., Ganesalingam, J., Jones, A. L. C., Mahady, K.,
Epton, S., Rinne, P., Sharma, P., Halse, O., Mehta, A.,
and Rueckert, D. (2014). Prediction of stroke throm-
bolysis outcome using ct brain machine learning. Neu-
roImage: Clinical, 4:635–640.
Berthier, M. L. (2005). Poststroke aphasia. Drugs & aging,
22(2):163–182.
BGSEGEV (2018). BGSegev STROKETOR - stroke detec-
tor. https://stroketor.segevlabs.org/.
Boulos, M. N. K., Brewer, A. C., Karimkhani, C., Buller,
D. B., and Dellavalle, R. P. (2014). Mobile medical
and health apps: state of the art, concerns, regula-
tory control and certification. Online journal of public
health informatics, 5(3):229.
Bunkheila, G. (retrieved Jan 2018). Signal processing
and machine learning techniques for sensor data an-
alytics. https://www.mathworks.com/videos/signal-
processing-and-machine-learning-techniques-for-
sensor-data-analytics-107549.html.
Centers for Disease Control and Prevention, USA, (2009).
Prevalence and most common causes of disability
among adults–united states, 2005. MMWR: Morbidity
and Mortality weekly report, 58(16):421–426.
Crockett, D. and Eliason, B. (2016). What is data mining in
healthcare. Insights: Health Catalyst.
de Bruin, J. S., Schuh, C., Seeling, W., Luger, E., Gall, M.,
H
¨
utterer, E., Kornek, G., Ludvik, B., Hoppichler, F.,
and Schindler, K. (2015). Assessing the feasibility
of a mobile health-supported clinical decision support
system for nutritional triage in oncology outpatients
using arden syntax. Artificial intelligence in medicine.
Demaerschalk, B. M., Vegunta, S., Vargas, B. B., Wu, Q.,
Channer, D. D., and Hentz, J. G. (2012). Reliability
of real-time video smartphone for assessing national
institutes of health stroke scale scores in acute stroke
patients. Stroke, 43(12):3271–3277.
Dobkin, B. H. and Dorsch, A. (2011). The promise of
mhealth: daily activity monitoring and outcome as-
sessments by wearable sensors. Neurorehabilitation
and neural repair, 25(9):788–798.
Freund, Y., Schapire, R., and Abe, N. (1999). A short in-
troduction to boosting. Journal-Japanese Society For
Artificial Intelligence, 14(771-780):1612.
Frid, A., Safra, E. J., Hazan, H., Lokey, L. L., Hilu, D.,
Manevitz, L., Ramig, L. O., and Sapir, S. (2014).
Computational diagnosis of parkinson’s disease di-
rectly from natural speech using machine learning
techniques. In Software Science, Technology and En-
gineering (SWSTE), 2014 IEEE International Confer-
ence on, pages 50–53. IEEE.
Gonzalez, M. A., Hanna, N., Rodrigo, M. E., Satler, L. F.,
and Waksman, R. (2011). Reliability of prehospital
real-time cellular video phone in assessing the simpli-
fied national institutes of health stroke scale in patients
with acute stroke. Stroke, 42(6):1522–1527.
Guo, X., Yu, Q., Li, R., Alm, C. O., Calvelli, C., Shi, P., and
Haake, A. (2016). Intelligent medical image grouping
through interactive learning. International Journal of
Data Science and Analytics, 2(3-4):95–105.
Hazan, H., Hilu, D., Manevitz, L., Ramig, L. O., and Sapir,
S. (2012). Early diagnosis of parkinson’s disease via
machine learning on speech data. In Electrical &
Electronics Engineers in Israel (IEEEI), 2012 IEEE
27th Convention of, pages 1–4. IEEE.
Khosla, A., Cao, Y., Lin, C. C.-Y., Chiu, H.-K., Hu, J.,
and Lee, H. (2010). An integrated machine learn-
ing approach to stroke prediction. In Proceedings of
the 16th ACM SIGKDD international conference on
Knowledge discovery and data mining, pages 183–
192. ACM.
Kim, D.-K., Yoo, S. K., Park, I.-C., Choa, M., Bae,
K. Y., Kim, Y.-D., and Heo, J.-H. (2009). A mobile
telemedicine system for remote consultation in cases
of acute stroke. Journal of telemedicine and telecare,
15(2):102–107.
King, C. E. and Sarrafzadeh, M. (2017). A survey of
smartwatches in remote health monitoring. Journal
of Healthcare Informatics Research, pages 1–24.
Koh, H. C., Tan, G., et al. (2011). Data mining applica-
tions in healthcare. Journal of healthcare information
management, 19(2):65.
Krpic, A., Savanovic, A., and Cikajlo, I. (2013). Telere-
habilitation: remote multimedia-supported assistance
and mobile monitoring of balance training outcomes
can facilitate the clinical staff’s effort. International
Journal of Rehabilitation Research, 36(2):162–171.
Little, M. A., McSharry, P. E., Hunter, E. J., Spielman, J.,
Ramig, L. O., et al. (2009). Suitability of dysphonia
measurements for telemonitoring of parkinson’s dis-
ease. IEEE transactions on biomedical engineering,
56(4):1015–1022.
Mans, R., Schonenberg, H., Leonardi, G., Panzarasa, S.,
Cavallini, A., Quaglini, S., and van der AALST, W.
(2008). Process mining techniques: an application to
stroke care. Studies in health technology and infor-
matics, 136:573.
Micallef, N., Baillie, L., and Uzor, S. (2016). Time to ex-
ercise!: an aide-memoire stroke app for post-stroke
arm rehabilitation. In Proceedings of the 18th Inter-
national Conference on Human-Computer Interaction
with Mobile Devices and Services, pages 112–123.
ACM.
Mitchell, J. R., Sharma, P., Modi, J., Simpson, M., Thomas,
M., Hill, M. D., and Goyal, M. (2011). A smartphone
client-server teleradiology system for primary diagno-
sis of acute stroke. Journal of medical Internet re-
search, 13(2).
Nam, H., Cha, M.-J., Kim, Y., Kim, E., Park, E., Lee,
H., Nam, C., and Heo, J. (2012). Use of a hand-
held, computerized device as a decision support tool
Mobile Applications for Stroke: A Survey and a Speech Classification Approach
165