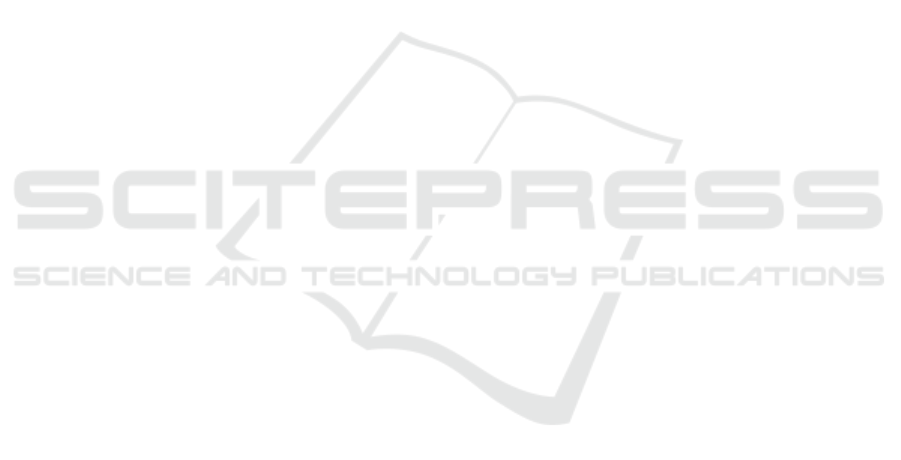
lies on having successful data mining models, and a
description of these is left for future work, as it is a
separate module of the system. Using data mining to
analyze the data collected in the app brings the sys-
tem even further towards better disease management.
In the future, we will offer research and analysis of
data from other diseases, such as cancer that our team
has begun to study, and adapt the system accordingly.
We have begun studying various data mining meth-
ods (Richardson et al., 2019) in order to select the
most appropriate models from our CDSS and will re-
port on progress in future work. Future studies will
also involve testing the system with both patient and
caregiver subjects.
REFERENCES
Artikis, A., Bamidis, P. D., Billis, A., Bratsas, C.,
Frantzidis, C., Karkaletsis, V., Klados, M., Konstan-
tinidis, E., Konstantopoulos, S., Kosmopoulos, D.,
et al. (2012). Supporting tele-health and ai-based
clinical decision making with sensor data fusion and
semantic interpretation: The usefil case study. In
International workshop on artificial intelligence and
NetMedicine, page 21.
BGSEGEV (2018). BGSegev STROKETOR - stroke detec-
tor. https://stroketor.segevlabs.org/.
Boulos, M. N. K., Brewer, A. C., Karimkhani, C., Buller,
D. B., and Dellavalle, R. P. (2014). Mobile medical
and health apps: state of the art, concerns, regula-
tory control and certification. Online journal of public
health informatics, 5(3):229.
Bourouis, A., Feham, M., Hossain, M. A., and Zhang, L.
(2014). An intelligent mobile based decision support
system for retinal disease diagnosis. Decision Support
Systems, 59:341–350.
Castaneda, C., Nalley, K., Mannion, C., Bhattacharyya, P.,
Blake, P., Pecora, A., Goy, A., and Suh, K. S. (2015).
Clinical decision support systems for improving di-
agnostic accuracy and achieving precision medicine.
Journal of clinical bioinformatics, 5(1):4.
Criqui, M. H. and Aboyans, V. (2015). Epidemiology
of peripheral artery disease. Circulation research,
116(9):1509–1526.
Demaerschalk, B. M., Vegunta, S., Vargas, B. B., Wu, Q.,
Channer, D. D., and Hentz, J. G. (2012). Reliability
of real-time video smartphone for assessing national
institutes of health stroke scale scores in acute stroke
patients. Stroke, 43(12):3271–3277.
Dobkin, B. H. and Dorsch, A. (2011). The promise of
mhealth: daily activity monitoring and outcome as-
sessments by wearable sensors. Neurorehabilitation
and neural repair, 25(9):788–798.
El-Sappagh, S. H. and El-Masri, S. (2014). A distributed
clinical decision support system architecture. Journal
of King Saud University-Computer and Information
Sciences, 26(1):69–78.
Kawamoto, K., Houlihan, C. A., Balas, E. A., and Lobach,
D. F. (2005). Improving clinical practice using clin-
ical decision support systems: a systematic review
of trials to identify features critical to success. Bmj,
330(7494):765.
Micallef, N., Baillie, L., and Uzor, S. (2016). Time to ex-
ercise!: an aide-memoire stroke app for post-stroke
arm rehabilitation. In Proceedings of the 18th Inter-
national Conference on Human-Computer Interaction
with Mobile Devices and Services, pages 112–123.
ACM.
Mitchell, J. R., Sharma, P., Modi, J., Simpson, M., Thomas,
M., Hill, M. D., and Goyal, M. (2011). A smartphone
client-server teleradiology system for primary diagno-
sis of acute stroke. Journal of medical Internet re-
search, 13(2).
Nam, H. S., Heo, J., Kim, J., Kim, Y. D., Song, T. J., Park,
E., and Heo, J. H. (2014). Development of smartphone
application that aids stroke screening and identifying
nearby acute stroke care hospitals. Yonsei medical
journal, 55(1):25–29.
Ozdalga, E., Ozdalga, A., and Ahuja, N. (2012). The smart-
phone in medicine: a review of current and potential
use among physicians and students. Journal of medi-
cal Internet research, 14(5).
Peleg, M., Shahar, Y., and Quaglini, S. (2014). Making
healthcare more accessible, better, faster, and cheaper:
the mobiguide project. European Journal of ePrac-
tice: Issue on Mobile eHealth, 20:5–20.
Peleg, M., Shahar, Y., Quaglini, S., Broens, T., Budasu,
R., Fung, N., Fux, A., Garc
´
ıa-S
´
aez, G., Goldstein,
A., Gonz
´
alez-Ferrer, A., et al. (2017). Assessment of
a personalized and distributed patient guidance sys-
tem. International journal of medical informatics,
101:108–130.
Richardson, A., Shani Ben Ari, and Sinai, M., Atsmon, A.,
Conley, E. S., Gat, Y., and Segev, G. (2019). Mobile
applications for stroke: A survey and a speech classi-
fication approach. In ICT4AWE, page to appear.
Seo, W.-K., Kang, J., Jeon, M., Lee, K., Lee, S., Kim, J. H.,
Oh, K., and Koh, S.-B. (2015). Feasibility of using
a mobile application for the monitoring and manage-
ment of stroke-associated risk factors. Journal of Clin-
ical Neurology, 11(2):142–148.
Shibl, R., Lawley, M., and Debuse, J. (2013). Factors influ-
encing decision support system acceptance. Decision
Support Systems, 54(2):953–961.
Terry, K. (2015). Number of health apps soars but use does
not always follow. Medscape Medical News.
Weymann, N., H
¨
arter, M., and Dirmaier, J. (2016). Informa-
tion and decision support needs in patients with type
2 diabetes. Health informatics journal, 22(1):46–59.
Zhang, M. W., Yeo, L. L., and Ho, R. C. (2015). Harness-
ing smartphone technologies for stroke care, rehabili-
tation and beyond. BMJ innovations, 1(4):145–150.
A Clinical Decision Support System based on an Unobtrusive Mobile App
173