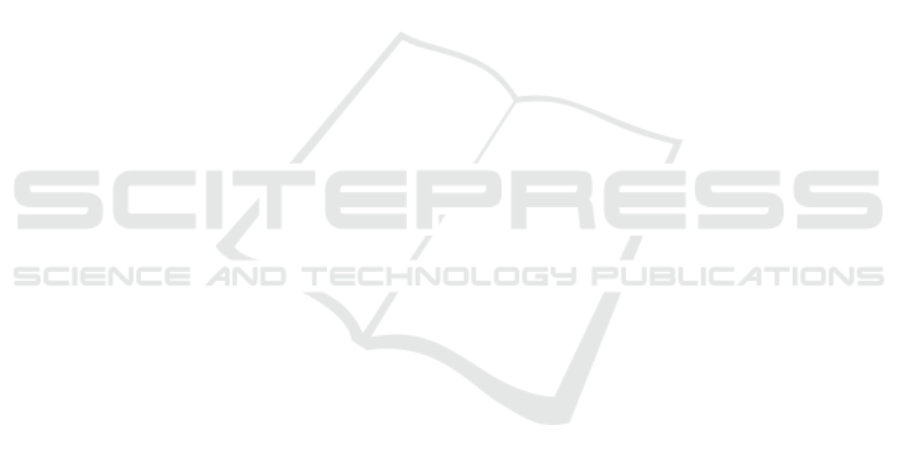
Discussion starts with stressing that the inspected
small number of epochs |T| respects that the
exploration-exploitation balance is vital in this case.
Otherwise, even a rare adding of random deviations
from exploitive actions, whose non-optimal character
with respect to the exploitation has negligible influ-
ence, guarantees convergence of learning and thus the
policy optimality. This distinguishes our experiments
from usual tests, e.g. (Ouyang et al., 2017), and makes
them relevant.
The experiments dealt with structurally same
static DM. The numerical choice of their parame-
ters was based on the following, qualitatively obvious
fact. The need for exploration (within the considered
short-horizon scenario) depends on the mutual rela-
tion of the prior probability p(θ|V
0
), see Table 4, and
the parameter θ
simulated
of the simulated environment
model determining transition probability, see Tables
2, 3. The influence of this relation is enhanced or at-
tenuated by the considered reward r.
The first experiment, reflected in Figure 1, in
which the DP policy is the best one warns that explo-
ration need not be always helpful. Notably, FPD and
Boltzmann’s machine with sufficiently small λ can be
arbitrarily close to its best behaviour. Due to the lack
of exploration significance no other conclusions con-
cerning the quality of the tested policies can be made.
But it calls for an improvement of λ-tuning, which
should converge to zero if the exploration is superflu-
ous.
The second experiment, reflected in Figure 2, is
more informative. The policy based on the newly
proposed relation of FPD with MDP and an adaptive
choice of λ (FPDExpAdaptive) brings the highest im-
provement (about 2%). A similar performance can be
reached for a fixed but properly chosen λ (FPDExp).
The adaptive FPD is worse (FPDAdaptive) but still
outperforms the remaining competitors. The similar-
ity of the results for the λ-dependent FPD and Boltz-
mann’s machine supports the conjecture that the per-
formance of Boltzmann’s machine can be improved
by adapting λ. This may be important in its other ap-
plications.
4 CONCLUDING REMARKS
The paper has arisen from inspecting the conjecture
that the certainty-equivalent version of non-traditional
fully probabilistic design (FPD) of decision policies
properly balances exploitation with exploration. The
achieved results support it. Moreover the paper: (a)
established a better relation of FPD to the wide-spread
Markov decision processes; (b) proposed an adaptive
tuning of the involved parameter, which can be used
in the closely-related simulated annealing and Boltz-
mann’s machine; (c) provided a sample of extensive
experiments, which confirmed that standard explo-
ration techniques are outperformed by the FPD-based
policies.
The future work will concern: (i) an algorithmic
recognition of cases in which exploration is unnec-
essary; (ii) inspection of a tuning mechanism based
on extremum-seeking control; (iii) an efficient im-
plementation of λ-tuning; (iv) application of the pro-
posed ideas to continuous-valued MDP; (v) real-life
problems, especially those in which a short, but non-
unit, decision horizon is vital as in environmental de-
cision making (Springborn, 2014).
REFERENCES
˚
Astr
¨
om, K. (1970). Introduction to Stochastic Control. Aca-
demic Press, NY.
Auer, P., Cesa-Bianchi, N., and Fischer, P. (2002). Finite-
time analysis of the multiarmed bandit problem. Ma-
chine Learning, 47(2-3):235–256.
Barndorff-Nielsen, O. (1978). Information and Exponential
Families in Statistical Theory. Wiley, NY.
Bellman, R. (1961). Adaptive Control Processes. Princeton
U. Press, NJ.
Berger, J. (1985). Statistical Decision Theory and Bayesian
Analysis. Springer, NY.
Bernardo, J. (1979). Expected information as expected util-
ity. The An. of Stat., 7(3):686–690.
Bertsekas, D. (2001). Dynamic Programming and Optimal
Control. Athena Scientific, US.
Cover, T. and Thomas, J. (1991). Elements of Information
Theory. Wiley. 2nd edition.
ˇ
Crepin
ˇ
sek, M., Liu, S., and Mernik, M. (2013). Exploration
and exploitation in evolutionary algorithms: A survey.
ACM Computing Survey, 45(3):37–44.
Duff, M. O. (2002). Optimal Learning; Computational
Procedures for Bayes-Adaptive Markov Decision Pro-
cesses. PhD thesis, University of Massachusetts
Amherst.
Feldbaum, A. (1960,61). Theory of dual control. Autom.
Remote Control, 21,22(9,2).
G
´
omez, A. G. V. and Kappen, H. (2012). Dynamic policy
programming. The J. of Machine Learning Research,
30:3207–3245.
Guan, P., Raginsky, M., and Willett, R. (2012). On-
line Markov decision processes with Kullback-Leibler
control cost. In American Control Conference, pages
1388–1393. IEEE.
Kappen, H. (2005). Linear theory for control of non-
linear stochastic systems. Physical review letters,
95(20):200201.
ICAART 2019 - 11th International Conference on Agents and Artificial Intelligence
862