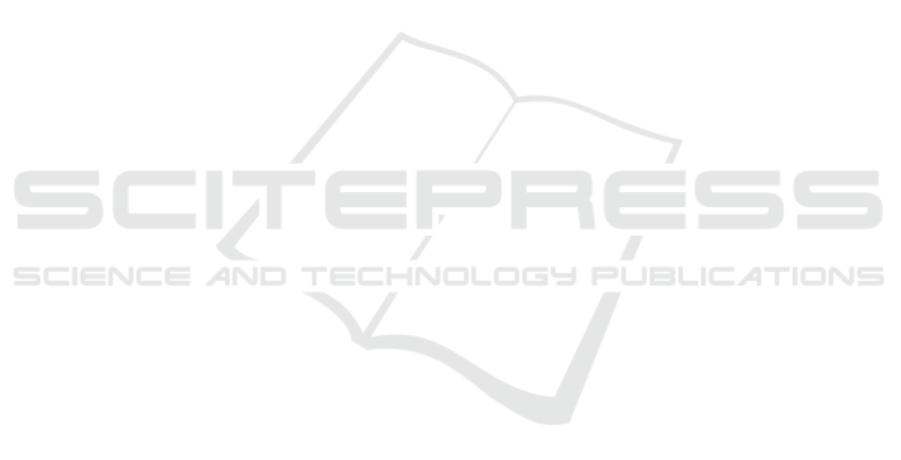
Dembczynski, K., Cheng, W., and H
¨
ullermeier, E. (2010).
Bayes optimal multilabel classification via probabilis-
tic classifier chains. In International Conference
on International Conference on Machine Learning,
pages 279–286.
Friedman, M. (1940). A comparison of alternative tests of
significance for the problem of m rankings. Annals of
Mathematical Statistics, 11(1):86–92.
Garcia, S. and Herrera, F. (2008). An extension on “sta-
tistical comparisons of classifiers over multiple data
sets” for all pairwise comparisons. Journal of Ma-
chine Learning Research, 9:2677–2694.
Gibaja, E. and Ventura, S. (2014). Multi-label learning: a
review of the state of the art and ongoing research.
Wiley Interdisciplinary Reviews: Data Mining and
Knowledge Discovery, 4(6):411–444.
Gibaja, E. and Ventura, S. (2015). A tutorial on multilabel
learning. ACM Computing Surveys, 47(3):52:1–52:38.
Goncalves, E., Plastino, A., and Freitas, A. A. (2015). Sim-
pler is better: a novel genetic algorithm to induce com-
pact multi-label chain classifiers. In Conference on
Genetic and Evolutionary Computation Conference,
pages 559–566.
Gonzalez-Lopez, J., Cano, A., and Ventura, S. (2017).
Large-scale multi-label ensemble learning on spark.
In IEEE Trustcom/BigDataSE/ICESS, pages 893–900.
Gonzalez-Lopez, J., Ventura, S., and Cano, A. (2018). Dis-
tributed nearest neighbor classification for large-scale
multi-label data on spark. Future Generation Com-
puter Systems, 87:66 – 82.
Hall, M., Frank, E., Holmes, G., Pfahringer, B., Reutemann,
P., and Witten, I. H. (2009). The weka data mining
software: An update. ACM SIGKDD Explorations
Newsletter, 11(1):10–18.
Hsu, D. J., Kakade, S. M., Langford, J., and Zhang, T.
(2009). Multi-label prediction via compressed sens-
ing. In International Conference on Neural Informa-
tion Processing Systems, pages 772–780.
Melki, G., Cano, A., Kecman, V., and Ventura, S. (2017).
Multi-target support vector regression via correlation
regressor chains. Information Sciences, 415-416:53–
69.
Moyano, J. M., Gibaja, E., and Ventura, S. (2017). An
evolutionary algorithm for optimizing the target or-
dering in ensemble of regressor chains. In 2017 IEEE
Congress on Evolutionary Computation (CEC), pages
2015–2021.
Moyano, J. M., Gibaja, E. L., Cios, K. J., and Ventura, S.
(2018). Review of ensembles of multi-label classi-
fiers: Models, experimental study and prospects. In-
formation Fusion, 44:33 – 45.
Nasierding, G. and Kouzani, A. (2010). Image to text trans-
lation by multi-label classification. In Advanced In-
telligent Computing Theories and Applications with
Aspects of Artificial Intelligence, volume 6216, pages
247–254.
Prabhu, Y. and Varma, M. (2014). Fastxml: A fast, accurate
and stable tree-classifier for extreme multi-label learn-
ing. In ACM SIGKDD International Conference on
Knowledge Discovery and Data Mining, pages 263–
272.
Read, J. (2008). A pruned problem transformation method
for multi-label classification. In Proceedings of the
NZ Computer Science Research Student Conference,
pages 143–150.
Read, J., Pfahringer, B., Holmes, G., and Frank, E. (2011).
Classifier chains for multi-label classification. Ma-
chine Learning, 85(3):335–359.
Roseberry, M. and Cano, A. (2018). Multi-label kNN
Classifier with Self Adjusting Memory for Drifting
Data Streams. In International Workshop on Learning
with Imbalanced Domains: Theory and Applications,
ECML-PKDD, pages 23–37.
Shaffer, J. P. (1986). Modified sequentially rejective multi-
ple test procedures. Journal of the American Statisti-
cal Association, 81(395):826–831.
Skryjomski, P., Krawczyk, B., and Cano, A. (2018). Speed-
ing up k-Nearest Neighbors Classifier for Large-Scale
Multi-Label Learning on GPUs. Neurocomputing, In
press.
Tang, L. and Liu, H. (2009). Scalable learning of collective
behavior based on sparse social dimensions. In ACM
Conference on Information and Knowledge Manage-
ment, pages 1107–1116.
Tsoumakas, G. and Katakis, I. (2007). Multi-label classi-
fication: An overview. International Journal of Data
Warehousing and Mining, 3(3):1–13.
Tsoumakas, G., Katakis, I., and Vlahavas, I. (2008). Effec-
tive and efficient multilabel classification in domains
with large number of labels. In Workshop on Mining
Multidimensional Data, ECML-PKDD.
Tsoumakas, G., Katakis, I., and Vlahavas, I. (2010). Data
Mining and Knowledge Discovery Handbook, Part
6, chapter Mining Multi-label Data, pages 667–685.
Springer.
Tsoumakas, G., Katakis, I., and Vlahavas, I. (2011a). Ran-
dom k-labelsets for multi-label classification. IEEE
Transactions on Knowledge and Data Engineering,
23(7):1079–1089.
Tsoumakas, G., Spyromitros-Xioufis, E., Vilcek, J., and
Vlahavas, I. (2011b). Mulan: A java library for
multi-label learning. Journal of Machine Learning
Research, 12:2411–2414.
Speeding Up Classifier Chains in Multi-label Classification
37