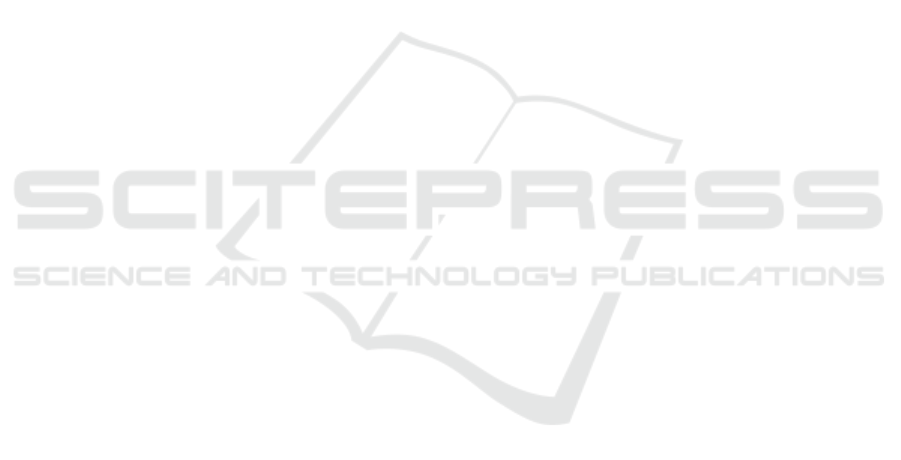
ing their users from the error-prone, manual process
required when a GIS tool is used to put into practice
the underlying methodologies—(Barra et al., 2017;
Tom
´
as et al., 2018). For instance, the ADAfinder
tool, for which former references existed, is at least
10 times faster than a regular GIS operator.
The tools are also flexible, being able to adapt
themselves to variations in the format of the input
shapefiles thanks to the use of the so-called ”read-map
files.”
Speed, flexibility and adequate GUI interfaces
make the toolset a very apt tool for research activ-
ities, where different scenarios—usually defined by
variations on the input parameters—may change the
results. On the other side, the availability of the
command-line versions of the tools makes possible to
integrate them in more production-oriented environ-
ments, thanks to the use of option files. Furthermore,
it is possible to use the toolset as a black box to in-
clude it in higher level software components, since
C++ classes have been included in the library imple-
menting the logic of the three applications. Last, but
not least, the tools, in their GUI versions, may be
integrated quite easily in either ArcGIS or Quantum
GIS—there is no need to modify the source code; this
may be done using the mechanisms provided by these
GIS tools. In this way, the applications become an
extra set of tools available in the regular work envi-
ronment of a GIS operator.
The toolset has undergone a very strict testing pro-
cess, using either real datasets and the comparison of
manual and automated results to check their validity
or synthetic ones when these were not available—that
is, for ADAclassifier and los2hv. In this case, how-
ever, the software is being actively tested nowadays,
and, although no results are available yet, the partial
outcomes are promising, thus confirming, at the time
being, the positive results of the formal validation pro-
cess.
ACKNOWLEDGEMENTS
This work has received funding from the Shift2Rail
Joint Undertaking under the European Union’s Hori-
zon 2020 research and innovation programme, with
grant agreement No 777630, project MOMIT, “Multi-
scale Observation and Monitoring of railway Infras-
tructure Threats”.
REFERENCES
Barra, A., Solari, L., B
´
ejar-Pizarro, M., Montserrat, O.,
Bianchini, S., Herrera, G., Crosetto, M., Sarro, R.,
Gonz
´
alez-Alonso, E., Mateos, R. M., Lig
¨
u
´
erzana, S.,
L
´
opez, C., and Moretti, S. (2017). A methodology to
detect and update Active Deformation Areas based on
Sentinel-1 SAR images. Remote Sensing, 9(10):1002.
MOMIT (2017). Multi-scale observation and monitor-
ing of railway infrastructure threats. Home | MOMIT
project consortium. http://www.momit-project.eu/.
Web page; accessed 4-December-2018.
Navarro, J. A. and Cuevas, M. (2018a). los2hv -
Compute horizontal and vertical components of the
movement. http://www.momit-project.eu/results-and-
publications. MOMIT project technical note. Online;
accessed 4-December-2018.
Navarro, J. A. and Cuevas, M. (2018b). ADAfinder - A
tool to automate the detection of Active Deformation
Areas. http://www.momit-project.eu/results-and-
publications. MOMIT project technical note. Online;
accessed 4-December-2018.
Navarro, J. A., Cuevas, M., Barra, A., and Crosetto, M.
(2018a). Detection of Active Deformation Areas
based on Sentinel-1 imagery: an efficient, fast and
flexible implementation. In Proceedings of 18th
International Scientific and Technical Conference
(RACURS 2018), Crete, Greece.
Navarro, J. A., Cuevas, M., and Crosetto, M. (2018b).
ADAclassifier - Classify ADAs automati-
cally. http://www.momit-project.eu/results-and-
publications. MOMIT project technical note. Online;
accessed 4-December-2018.
Navarro, J. A., Cuevas, M., and Crosetto, M.
(2018c). SW data processing components test
plan. http://www.momit-project.eu/results-and-
publications. MOMIT project deliverable. Online;
accessed 4-December-2018.
Navarro, J. A., Tom
´
as, R., Cuevas, M., and Crosetto,
M. (2018d). SW data processing components
test report. http://www.momit-project.eu/results-and-
publications. MOMIT project deliverable. Online; ac-
cessed 4-December-2018.
Notti, D., Herrera, G., Bianchini, S., Meisina, S., Garc
´
ıa-
Davalillo, J. C., and Zucca, F. (2014). A methodol-
ogy for improving landslide PSI data analysis. Inter-
national Journal of Remote Sensing, (35):2186–2214.
doi: 10.1080/01431161.2014.889864.
Tom
´
as, R., Cano, M., Pastor, J. L., and Riquelme, A. (2018).
Automatic classification of Active Deformation
Areas. http://www.momit-project.eu/results-and-
publications. MOMIT project technical note. Online;
accessed 4-December-2018.
GISTAM 2019 - 5th International Conference on Geographical Information Systems Theory, Applications and Management
174