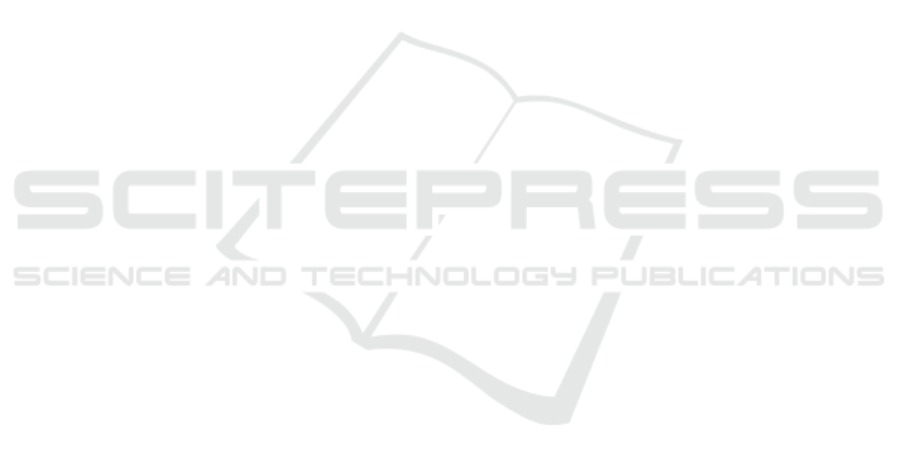
frontier: insights from hundreds of use cases. Techni-
cal Report April, McKinsey Global Institute.
Colder, B. (2011). Emulation as an integrating principle for
cognition. Frontiers in Human Neuroscience, 5:Arti-
cle 54.
Da Lio, M., Plebe, A., Bortoluzzi, D., Rosati Papini, G. P.,
and Don
`
a, R. (2018). Autonomous vehicle architec-
ture inspired by the neurocognition of human driv-
ing. In International Conference on Vehicle Tech-
nology and Intelligent Transport Systems, pages 507–
513. Scitepress.
Damasio, A. (1989). Time-locked multiregional retroacti-
vation: A systems-level proposal for the neural sub-
strates of recall and recognition. Cognition, 33:25–62.
Glorot, X. and Bengio, Y. (2010). Understanding the dif-
ficulty of training deep feedforward neural networks.
In International Conference on Artificial Intelligence
and Statistics, pages 249–256.
Grillner, S. and Wall
´
en, P. (2004). Innate versus learned
movements – a false dichotomy. Progress in Brain
Research, 143:1–12.
Grush, R. (2004). The emulation theory of representation:
Motor control, imagery, and perception. Behavioral
and Brain Science, 27:377–442.
Hazelwood, K., Bird, S., Brooks, D., Chintala, S., Diril, U.,
Dzhulgakov, D., Fawzy, M., Jia, B., Jia, Y., Kalro, A.,
Law, J., Lee, K., Lu, J., Noordhuis, P., Smelyanskiy,
M., Xiong, L., and Wang, X. (2018). Applied machine
learning at Facebook: A datacenter infrastructure per-
spective. In IEEE International Symposium on High
Performance Computer Architecture (HPCA), pages
620–629.
Hesslow, G. (2012). The current status of the simulation
theory of cognition. Brain, 1428:71–79.
Hinton, G. E. and Salakhutdinov, R. R. (2006). Reducing
the dimensionality of data with neural networks. Sci-
ence, 28:504–507.
Jeannerod, M. (2001). Neural simulation of action: A uni-
fying mechanism for motor cognition. NeuroImage,
14:S103–S109.
Jones, W., Alasoo, K., Fishman, D., and Parts, L. (2017).
Computational biology: deep learning. Emerging Top-
ics in Life Sciences, 1:136–161.
Kingma, D. P. and Ba, J. (2014). Adam: A method for
stochastic optimization. In Proceedings of Interna-
tional Conference on Learning Representations.
Kosslyn, S. M. (1994). Image and Brain: the Resolution of
the Imagery Debate. MIT Press, Cambridge (MA).
Krizhevsky, A. and Hinton, G. E. (2011). Using very deep
autoencoders for content-based image retrieval. In
European Symposium on Artificial Neural Networks,
Computational Intelligence and Machine Learning,
pages 489–494.
Kulkarni, T. D., Whitney, W. F., Kohli, P., and Tenenbaum,
J. B. (2015). Deep convolutional inverse graphics net-
work. In Advances in Neural Information Processing
Systems, pages 2539–2547.
Larochelle, H., Bengio, Y., Louradour, J., and Lamblin, P.
(2009). Exploring strategies for training deep neu-
ral networks. Journal of Machine Learning Research,
1:1–40.
Li, J., Cheng, H., Guo, H., and Qiu, S. (2018). Survey on
artificial intelligence for vehicles. Automotive Innova-
tion, 1:2–14.
Liu, D. and Todorov, E. (2007). Evidence for the flexible
sensorimotor strategies predicted by optimal feedback
control. Journal of Neuroscience, 27:9354–9368.
Liu, W., Wang, Z., Liu, X., Zeng, N., Liu, Y., and Alsaadi,
F. E. (2017). A survey of deep neural network ar-
chitectures and their applications. Neurocomputing,
234:11–26.
Mahon, B. Z. and Caramazza, A. (2011). What drives the
organization of object knowledge in the brain? the dis-
tributed domain-specific hypothesis. Trends in Cogni-
tive Sciences, 15:97–103.
Martin, A. (2007). The representation of object concepts in
the brain. Annual Review of Psychology, 58:25–45.
Mayer, N., Ilg, E., H
¨
ausser, P., Fischer, P., Cremers, D.,
Dosovitskiy, A., and Brox, T. (2016). A large dataset
to train convolutional networks for disparity, optical
flow, and scene flow estimation. In Proc. of IEEE In-
ternational Conference on Computer Vision and Pat-
tern Recognition, pages 4040–4048.
Meyer, K. and Damasio, A. (2009). Convergence and di-
vergence in a neural architecture for recognition and
memory. Trends in Neuroscience, 32:376–382.
Moulton, S. T. and Kosslyn, S. M. (2009). Imagining
predictions: mental imagery as mental emulation.
Philosophical transactions of the Royal Society B,
364:1273–1280.
NHTSA (2017). Fatality Analysis Reporting System
(FARS).
Olier, J. S., Barakova, E., Regazzoni, C., and Rauterberg,
M. (2017). Re-framing the characteristics of concepts
and their relation to learning and cognition in artificial
agents. Cognitive Systems Research, 44:50–68.
Ras, G., van Gerven, M., and Haselager, P. (2018). Explana-
tion methods in deep learning. In Escalante, H. J., Es-
calera, S., Guyon, I., Bar
´
o, X., G
¨
uc¸l
¨
ut
¨
urk, Y., G
¨
uc¸l
¨
u,
U., and van Gerven, M., editors, Explainable and In-
terpretable Models in Computer Vision and Machine
Learning. Springer-Verlag, Berlin.
Samek, W., Wiegand, T., and M
¨
uller, K. (2017). Explain-
able artificial intelligence: Understanding, visualiz-
ing and interpreting deep learning models. CoRR,
abs/1708.08296.
Schmidhuber, J. (2015). Deep learning in neural networks:
An overview. Neural Networks, 61:85–117.
Schwarting, W., Alonso-Mora, J., and Rus, D. (2018). Plan-
ning and decision-making for autonomous vehicles.
Annual Review of Control, Robotics, and Autonomous
Systems, 1:8.1–8.24.
Seger, C. A. and Miller, E. K. (2010). Category learning
in the brain. Annual Review of Neuroscience, 33:203–
219.
Sudre, C. H., Li, W., Vercauteren, T., Ourselin, S., and Car-
doso, M. J. (2017). Generalised dice overlap as a deep
learning loss function for highly unbalanced segmen-
tations. In Cardoso, J., Arbel, T., Carneiro, G., Syeda-
VEHITS 2019 - 5th International Conference on Vehicle Technology and Intelligent Transport Systems
50