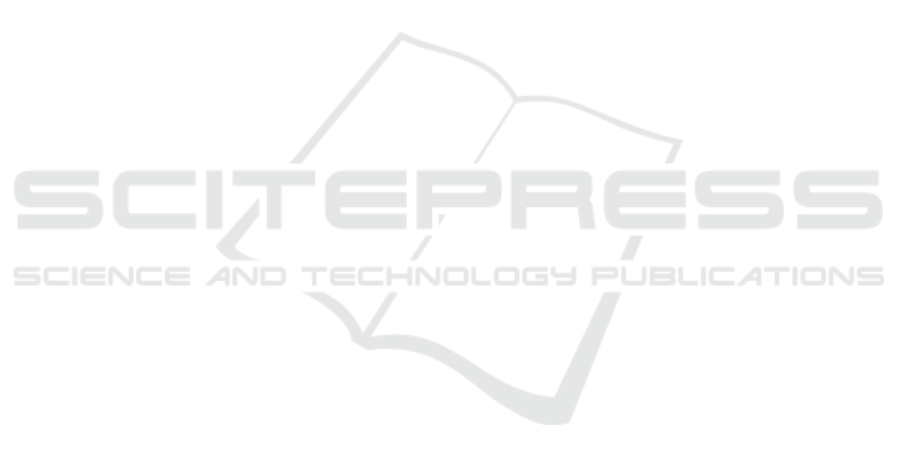
With the development of Internet of Things
technology, patients will have more complete data,
and the accuracy rate will increase. On the other
hand, this SVM classifier was only used to judge the
screening effect of each method. As mentioned in
Chapter 2, the system classification module used
weighted algorithm. Therefore, the classification
effect of the whole system will be more accurate.
5 CONCLUSION
In this paper, we designed a risk factor screening
module using different screening methods based on
the proposed context-aware system for hypertension.
After comparison and improvement, we selected the
RFSS method combined by random forest and
stability selection in four methods. We gradually
filtered 32 parameters in context information
obtained from DA module to 13 hypertension risk
factors, and performed by SVM classification
algorithm. Accuracy, sensitivity and specificity have
been improved. This method can improve the
screening rate and ensure the accuracy of this
system. Therefore, the context-aware system of
hypertension will improve performance by using this
screening method. The output of the system can
assist doctors and patients to have a comprehensive
understanding of their blood pressure condition
according to risk factors.
In the future research, we will increase the
dataset and data parameters with the improvement of
the performance of portable devices. On this basis,
we will extend this work by applying DL
technologies such as CNN, in order to see whether
the accuracy can be increased. In addition, statistical
hypothesis testing will be added to experimental
verification and the results will be compared with
clinical practice. Finally, the automated hypertension
risk assessment approach will be improved based on
future study. The diagnosis of doctors will be more
accurate and comprehensive.
ACKNOWLEDGEMENTS
This work was supported by National Natural
Science Foundation of China (No.61471064), and
National Science and Technology Major Project of
China (No.2017ZX03001022).
REFERENCES
Lim S. S., Vos T, Flaxman AD, Danaei G, Shibuya K,
Adair-Rohani H. et al., 2012. A comparative risk
assessment of burden of disease and injury attributable
to 67 risk factors and risk factor clusters in 21 regions,
1990–2010: a systematic analysis for the Global
Burden of Disease Study 2010. The Lancet,
380(9859), pp.2224-2260.
Chen, W., R.Gao, L.Liu, 2014. China Cardiovascular
Disease Report 2013. Chinese Circulation Journal,
29(07), pp.487-491.
Carey R. M, Whelton P K, 2018. Prevention, Detection,
Evaluation, and Management of High Blood Pressure
in Adults: Synopsis of the 2017 American College of
Cardiology/American Heart Association Hypertension
Guideline. Annals of Internal Medicine.
Whitworth J. A, Chalmers J., 2004. World health
organisation-international society of hypertension
(WHO/ISH) hypertension guidelines. Clinical and
Experimental Hypertension, 26, pp. 747-752.
Guixia Kang, Meikui Zhang, 2010. Recent Advances of
Wireless eHealth (WeHealth) in Terms of ’Sensing
China’. Proceedings of the 5th International
Conference on Cooperation and Promotion of
Information Resources in Science and Technology,
pp.517-519.
Sandi, G., I. G. B. B. Nugraha and S. H. Supangkat, 2013.
Mobile health monitoring and consultation to support
hypertension treatment. International Conference on
ICT for Smart Society, pp. 1-5.
Hong-Tao, Y. U., J. L. Wang and L. I. LiLua, 2007.
Research on the risk factors of hypertension and
OSAHS in the snore patients with different living
habits. Journal of Clinical Pulmonary Medicine.
Kunes, J. and Zicha, J., 2009. The interaction of genetic
and environmental factors in the ethology of
hypertension. Physiological Research, 58, pp. S33.
Ding C., Peng H., 2005. Feature selection based on mutual
information criteria of max-dependency, max-
relevance, and min-redundancy. IEEE Transactions on
Pattern Analysis and Machine Intelligence, 27(8), pp.
1226-1238.
Kohavi R., John G. H., 1997. Wrappers for feature subset
selection. Artificial Intelligence, 97(1-2), pp.273-324.
Hu Z. Y, Bao Y. K., 2015. Hybrid filter-wrapper feature
selection for short-term load forecasting. Engineering
Applications of Artificial Intelligence, 40, pp.17-27.
Koenigstein N., Paqued U., 2013. Xbox movies
recommendations: Variational Bayes matrix
factorization with embedded feature selection.
Proceedings of the 7th ACM conference on
Recommender Systems.
Madan, A. K., H. Dureja S. Gupta et al., 2008.
Topological models for prediction of pharmacokinetic
parameters of cephalosporins using random forest,
decision tree and moving average analysis. Sci Pharm,
76, pp.377–394.
Breiman, L., 2001. Random forest. Machine Learning, 45,
pp. 5–32.
A Risk Factors Screening Method in the Context-aware System of Hypertension
55