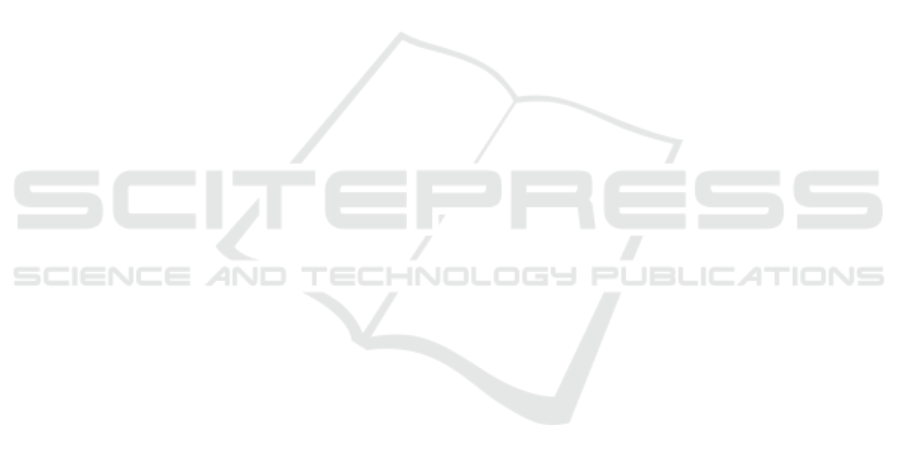
classification approaches are implemented and cross
tested on the discussed data classes. The results show
that there are significant differences in the accuracy
of the classification approaches in context of specific
data classes. The use of a flexible and dedicated clas-
sification of semantic concepts, like we presented in
this paper allows for better suggestions and improves
the semantic labeling process massively. To improve
our approach, we already started to evaluate the use
of a summarized representative, using a combination
of multiple classification approaches. In this con-
text, we envision the use of a hierarchical approach
for the classification, i.e., creating a global classi-
fier which contains a selection of specialized ones.
Creating specialized classifications would result in a
self optimized adaptation of the look up strategies of
a global method. However, at the moment, the re-
sults of our summarized representatives are only sim-
ilar to the ones achieved without the creation of sum-
marized representatives. Hence, further research is
needed. Beside summarizing representatives, another
direction of research includes the enhancement of the
approaches applied to the different classes, such as us-
ing further machine learning methods for dealing with
full texts. Another important point is the evaluation
of different classification approaches. Preliminary re-
sults of using machine learning-based approaches like
Autoencoders promise better classification results. In
addition, it must be examined to what extent the re-
sults of our approach can be generalized in larger real
applications. It will therefore be necessary to carry
out evaluations with a larger number of data sets with
similar yet different concepts and data attributes. In
these cases it might be helpful to additionally support
the classification by considering label-based sugges-
tions with methods like (Paulus et al., 2018). Finally,
the determination of the data classes is currently lim-
ited to the identified ones and is a manual process. An
extension would include the implementation of a fea-
ture that allows for any granularity in data classes and
an automated identification of those.
REFERENCES
Craswell, N. (2009). Mean Reciprocal Rank. In LIU, L.
and
¨
OZSU, M. T., editors, Encyclopedia of Database
Systems, page 1703. Springer US, Boston, MA.
Goel, A., Knoblock, C. A., and Lerman, K. (2011). Using
Conditional Random Fields to Exploit Token Struc-
ture and Labels for Accurate Semantic Annotation. In
Proceedings of the 25th National Conference on Arti-
ficial Intelligence (AAAI-11), San Francisco, CA.
Goel, A., Knoblock, C. A., and Lerman, K. (2012). Ex-
ploiting Structure within Data for Accurate Labeling
Using Conditional Random Fields. In Proceedings of
the 14th International Conference on Artificial Intelli-
gence (ICAI).
Groß, A., Pruski, C., and Rahm, E. (2016). Evolution
of biomedical ontologies and mappings: Overview
of recent approaches. Computational and structural
biotechnology journal, 14:333–340.
Li, X., Tu, Z., Jia, Q., Man, X., Wang, H., and Zhang,
X. (2017). Deep-level Quality Management Based
on Big Data Analytics with Case Study. In Pro-
ceedings 2017 Chinese Automation Congress (CAC),
pages 4921–4926, Piscataway, NJ. IEEE.
Paulus, A., Pomp, A., Poth, L., Lipp, J., and Meisen, T.
(2018). Gathering and Combining Semantic Concepts
from Multiple Knowledge Bases. In Proceedings of
the 20th International Conference on Enterprise In-
formation Systems, pages 69–80. SCITEPRESS - Sci-
ence and Technology Publications.
Pham, M., Alse, S., Knoblock, C. A., and Szekely, P.
(2016). Semantic Labeling: A Domain-Independent
Approach. In Groth, P., editor, The semantic web -
ISWC 2016, Lecture note in computer science, pages
446–462. Springer, Cham.
Pomp, A., Paulus, A., Jeschke, S., and Meisen, T. (2017).
ESKAPE: Information Platform for Enabling Seman-
tic Data Processing. In Proceedings of the 19th
International Conference on Enterprise Information.
SCITEPRESS - Science and Technology Publications.
Pomp, A., Paulus, A., Klischies, D., Schwier, C., and
Meisen, T. (2018). A Web-based UI to Enable Se-
mantic Modeling for Everyone. In SEMANTiCS 2018
14th International Conference on Semantic Systems.
Ramnandan, S. K., Mittal, A., Knoblock, C. A., and
Szekely, P. (2015). Assigning Semantic Labels to
Data Sources. In Gandon, F., Sabou, M., Sack, H.,
d’Amato, C., Cudr
´
e-Mauroux, P., and Zimmermann,
A., editors, The Semantic Web. Latest Advances and
New Domains: 12th European Semantic Web Confer-
ence, ESWC 2015, Portoroz, Slovenia, May 31 – June
4, 2015. Proceedings, pages 403–417. Springer Inter-
national Publishing, Cham.
RWTH Aachen University (2017). Digital Connected
Production. https://www.rwth-campus.com/
wp-content/uploads/2015/01/Broschuere-Cluster-
Productionstechnik-20170508-web.pdf.
Syed, Z., Finin, T., Mulwad, V., and Joshi, A. (2010). Ex-
ploiting a web of semantic data for interpreting tables.
In Proceedings of the Second Web Science Confer-
ence, volume 5.
Terrizzano, I. G., Schwarz, P. M., Roth, M., and Colino,
J. E. (2015). Data Wrangling: The Challenging Your-
ney from the Wild to the Lake. In CIDR.
Wang, J., Wang, H., Wang, Z., and Zhu, K. (2012). Un-
derstanding tables on the web. Conceptual Modeling,
pages 141–155.
ICEIS 2019 - 21st International Conference on Enterprise Information Systems
60