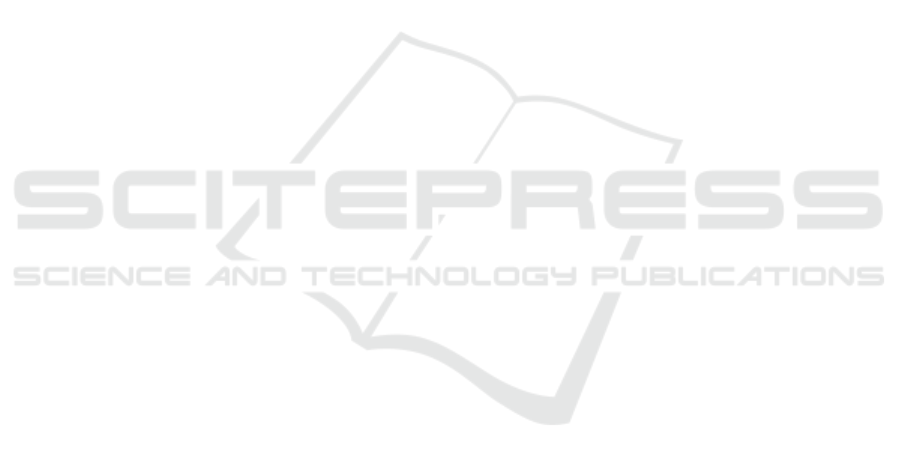
With the increasing growth of ubiquitous and per-
vasive technologies directed to internal environments,
it is evident the need of the applications to know the
location of the mobile devices to better adapt to sit-
uations, offering better services. Identifying that a
user is in a certain environment, because it is port-
ing a smartphone, creates several new possibilities
for applications. The WALDO provides parameters
to identify the location of mobile devices, with low
location errors, making it suitable for many types of
applications. The precision tests were performed us-
ing two distinct datasets, aiming to obtain non-biased
results. Although this is an opportunistic approach,
using variable signal technology, the test results were
satisfactory.
As future work, tests will be performed regard-
ing the influence caused by the use of heterogeneous
devices during the estimation of positioning. In addi-
tion, it is intended to implement a system that obtains
the RSS and MAC information through the APs, in
a passive tracking approach. In this way, it is possi-
ble to estimate the location of both sides of the com-
munication, seeking to improve the precision in the
estimates. In addition, the use of other information
sources along with Wi-Fi will be tested.
ACKNOWLEDGEMENTS
The authors would like to thank Penguim Formula
for partial supporting/funding of this research and
UFSM/FATEC through project number 041250 -
9.07.0025 (100548).
REFERENCES
Ahmed, T., Calders, T., and Pedersen, T. B. T. (2016). An-
alytics on indoor moving objects with applications in
airport baggage tracking.
Chen, L.-H., Wu, E. H.-K., Jin, M.-H., and Chen, G.-H.
(2014). Homogeneous features utilization to address
the device heterogeneity problem in fingerprint local-
ization. IEEE Sensors Journal, 14(4):998–1005.
Fukuzaki, Y., Mochizuki, M., Murao, K., and Nishio, N.
(2015). Statistical analysis of actual number of pedes-
trians for wi-fi packet-based pedestrian flow sensing.
In Adjunct Proceedings of the 2015 ACM Interna-
tional Joint Conference on Pervasive and Ubiquitous
Computing and Proceedings of the 2015 ACM Inter-
national Symposium on Wearable Computers, pages
1519–1526. ACM.
Ge, X. and Qu, Z. (2016). Optimization wifi indoor posi-
tioning knn algorithm location-based fingerprint. In
Software Engineering and Service Science (ICSESS),
2016 7th IEEE International Conference on, pages
135–137. IEEE.
Group, I. . W. et al. (1999). Part11: Wireless lan medium
access control (mac) and physical layer (phy) specifi-
cations. ANSI/IEEE Std. 802.11.
Hossain, A. M. and Soh, W.-S. (2015). A survey of
calibration-free indoor positioning systems. Com-
puter Communications, 66:1–13.
Kanan, R. and Elhassan, O. (2016). A combined batteryless
radio and wifi indoor positioning for hospital nursing.
Kannan, B., Meneguzzi, F., Dias, M. B., and Sycara, K.
(2013). Predictive indoor navigation using commer-
cial smart-phones. In Proceedings of the 28th Annual
ACM Symposium on Applied Computing, pages 519–
525. ACM.
Lane, N. D., Chon, Y., Zhou, L., Zhang, Y., Li, F., Kim,
D., Ding, G., Zhao, F., and Cha, H. (2013). Pig-
gyback crowdsensing (pcs): energy efficient crowd-
sourcing of mobile sensor data by exploiting smart-
phone app opportunities. In Proceedings of the 11th
ACM Conference on Embedded Networked Sensor
Systems, page 7. ACM.
Laoudias, C., Pich
´
e, R., and Panayiotou, C. (2013). De-
vice self-calibration in location systems using signal
strength histograms. 7:165–181.
Lymberopoulos, D., Liu, J., Yang, X., Choudhury, R. R.,
Handziski, V., and Sen, S. (2015). A realistic evalua-
tion and comparison of indoor location technologies:
Experiences and lessons learned. In Proceedings of
the 14th international conference on information pro-
cessing in sensor networks, pages 178–189. ACM.
Mainetti, L., Patrono, L., and Sergi, I. (2014). A survey on
indoor positioning systems. In Software, Telecommu-
nications and Computer Networks (SoftCOM), 2014
22nd International Conference on, pages 111–120.
IEEE.
Oosterlinck, D., Benoit, D. F., Baecke, P., and Van de
Weghe, N. (2017). Bluetooth tracking of humans in
an indoor environment: An application to shopping
mall visits. Applied Geography, 78:55–65.
Paul, A. S. and Wan, E. A. (2009). Rssi-based indoor
localization and tracking using sigma-point kalman
smoothers. IEEE Journal of Selected Topics in Sig-
nal Processing, 3(5):860–873.
Potort
`
ı, F., Crivello, A., Girolami, M., Traficante, E., and
Barsocchi, P. (2016). Wi-fi probes as digital crumbs
for crowd localisation. pages 1–8.
Rai, A., Chintalapudi, K. K., Padmanabhan, V. N., and Sen,
R. (2012). Zee: Zero-effort crowdsourcing for indoor
localization. In Proceedings of the 18th annual in-
ternational conference on Mobile computing and net-
working, pages 293–304. ACM.
Yang, C. and Shao, H.-R. (2015). Wifi-based indoor posi-
tioning. IEEE Communications Magazine, 53(3):150–
157.
Yang, S., Dessai, P., Verma, M., and Gerla, M. (2013). Free-
loc: Calibration-free crowdsourced indoor localiza-
tion. In INFOCOM, 2013 Proceedings IEEE, pages
2481–2489. IEEE.
ICEIS 2019 - 21st International Conference on Enterprise Information Systems
578