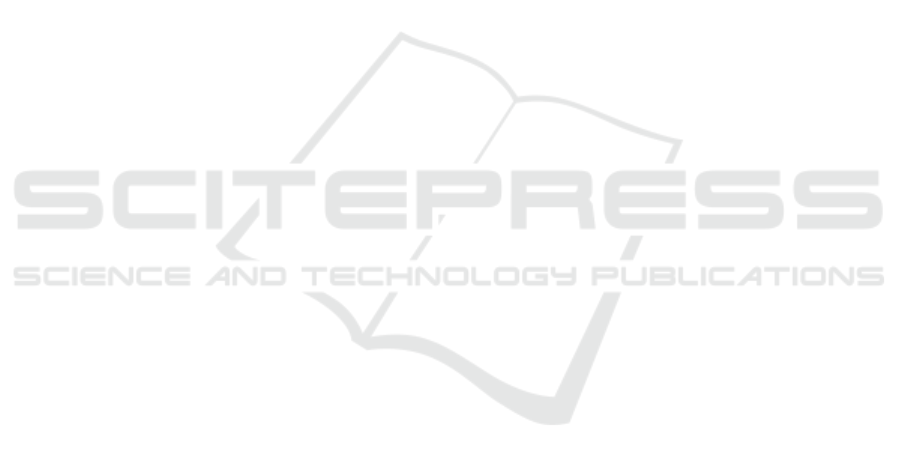
formance can also be limited due to moving objects
in the scene under low active illumination conditions.
Moving objects can require to reduce the integration
time in that case, but and as a consequence, the noise
level and σ will increase (Gay-Bellile et al., 2015),
limiting thus the maximum distance range.
The presence of light absorbent materials poses a
severe challenge for the ToF camera. Light absorbent
objects cannot be detected by this type of camera,
even at relatively short distances. This dependency on
the material’s reflectivity makes caregiver detection a
challenge when low reflective clothing are used. As a
countermeasure the active illumination power can be
increased at the cost of higher power consumption.
By contrast, STCs can detect light absorbent ob-
jects under ambient daylight conditions. However, re-
flective objects cause inaccurate depth measurements
by the STCs. This inaccuracy is most likely caused
by the lack of texture on the saturated reflective patch.
A similar behavior was observed for the non-textured
GP. Disparity computation errors occur due to the lack
of texture. If such errors originate from saturated re-
gions, the problem could probably be mitigated if a
high dynamic range imager were used. Where the
levels of GP texture are limited, increasing the size
of the pixel block used to compute pixel disparities
could help. We can expect this problem for indoor
scenarios, icy/wet conditions outdoors, or for clothing
with low levels of texture. However, GPs in outdoor
conditions normally provide good enough texture for
STCs.
Since STCs are able to operate in daylight con-
ditions without additional active illumination, a lot
of heat dissipation and power consumption can be
avoided. However, ToF cameras require active illu-
mination in all scenarios in order to maintain a high
level of SNR. As a consequence, ToF cameras have
much higher levels of heat dissipation that require a
careful thermal design. Embedding electronics into
the armrest of a PWC can be a challenge at higher
levels of heat dissipation. This is never the case when
STCs are used in daylight conditions.
6 CONCLUSIONS
In this paper, we reviewed challenges related to inte-
gration and the use of depth cameras on PWCs.
The main limitation of ToF cameras is the noise
level in combination with the active illumination
power. STCs, for their part, are limited by the level of
ambient illumination and by the texture of the objects
in order to successfully compute the pixel disparities.
Both STC and ToF camera technologies are suit-
able for e.g. obstacle or caregiver detection by the
PWC but we prefer STC because of the lower heat
dissipation and power savings in daylight conditions,
as well as the more robust detection of light absorbent
materials. The results from this study should be rele-
vant for any low-speed vehicle or autonomous robot.
As a future step, we plan to assess the dynamic
performance of both cameras under dynamic condi-
tions with moving objects in the scene. We believe
that the dynamic performances will be degraded un-
der low illumination levels and higher exposure or in-
tegration times.
REFERENCES
Beder, C., Bartczak, B., and Koch, R. (2007). A Compari-
son of PMD-Cameras and Stereo-Vision for the Task
of Surface Reconstruction using Patchlets. In Con-
ference on Computer Vision and Pattern Recognition
(CVPR), pages 1–8.
Cho, J., Choi, J., Kim, S.-J., Park, S., Shin, J., Kim, J. D.,
and Yoon, E. (2014). A 3-D Camera With Adaptable
Background Light Suppression Using Pixel-Binning
and Super-Resolution. IEEE Journal of Solid-State
Circuits, 49(10):2319–2332.
Choi, S., Park, J., Byun, J., and Yu, W. (2014). Robust
Ground Plane Detection from 3D Point Clouds. In
14th International Conference on Control, Automa-
tion and Systems (ICCAS), pages 1076–1081.
Dashpute, A., Anand, C., and Sarkar, M. (2018). Depth
Resolution Enhancement in Time-of-Flight Cameras
Using Polarization State of the Reflected Light. IEEE
Transactions on Instrumentation and Measurement,
PP(1):1–9.
Francis, S. L. X., Anavatti, S. G., Garratt, M., and Shim,
H. (2015). A ToF-Camera as a 3D Vision Sensor for
Autonomous Mobile Robotics. International Journal
of Advanced Robotic Systems, 12(11):156.
Gay-Bellile, V., Bartoli, A., Hamrouni, K., Sayd, P., Bour-
geois, S., and Belhedi, A. (2015). Noise modelling
in time-of-flight sensors with application to depth
noise removal and uncertainty estimation in three-
dimensional measurement. IET Computer Vision,
9(6):967–977.
Gonzalez, R. and Woods, R. (2010). Digital Image Process-
ing (Third Edition). Prentice-Hall Inc.
He, Y., Liang, B., Zou, Y., He, J., and Yang, J. (2017).
Depth Errors Analysis and Correction for Time-of-
Flight (ToF) Cameras. Sensors, 17(1):92.
Hussmann, S., Knoll, F., and Edeler, T. (2014). Modula-
tion method including noise model for minimizing the
wiggling error of tof cameras. IEEE Transactions on
Instrumentation and Measurement, 63(5):1127–1136.
Kazmi, W., Foix, S., Aleny
`
a, G., and Andersen, H. J.
(2014). Indoor and outdoor depth imaging of leaves
with time-of-flight and stereo vision sensors: Analy-
VEHITS 2019 - 5th International Conference on Vehicle Technology and Intelligent Transport Systems
84