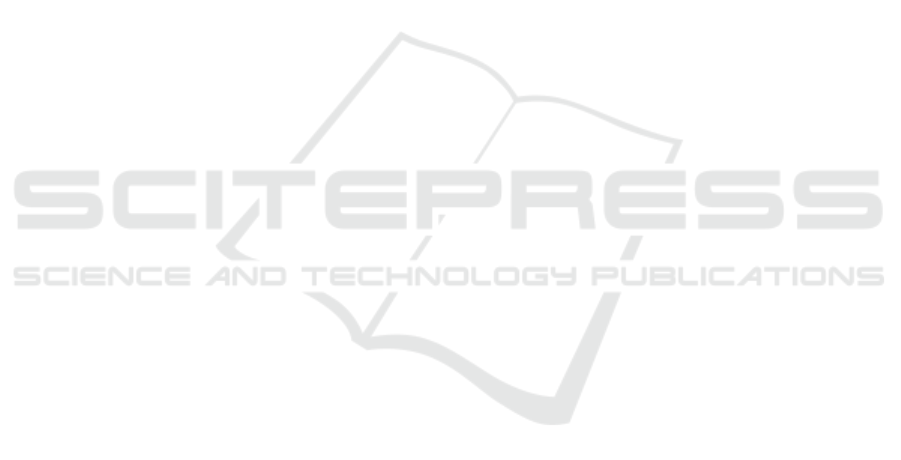
tion courses with the learning analytics multitrail ap-
proach. J. UCS, 21(1):23–47.
Clements, M. K. (1980). Analyzing children’s errors on
written mathematical tasks. Educational studies in
mathematics, 11(1):1–21.
Cooper, H. (1989). Synthesis of research on homework.
Educational leadership, 47(3):85–91.
Corrin, L. and de Barba, P. (2014). Exploring students’
interpretation of feedback delivered through learning
analytics dashboards. In Proceedings of the ascilite
2014 conference, pages 629–633.
Cripps, A. (1996). Using artificial neural nets to predict
academic performance. In Proceedings of the 1996
ACM Symposium on Applied Computing, pages 33–
37. ACM.
Dejaeger, K., Goethals, F., Giangreco, A., Mola, L., and
Baesens, B. (2012). Gaining insight into student
satisfaction using comprehensible data mining tech-
niques. European Journal of Operational Research,
218(2):548–562.
Dekker, G., Pechenizkiy, M., and Vleeshouwers, J. (2009).
Predicting students drop out: A case study. In Educa-
tional Data Mining 2009.
Giesbers, B., Rienties, B., Tempelaar, D., and Gijselaers,
W. (2013). Investigating the relations between motiva-
tion, tool use, participation, and performance in an e-
learning course using web-videoconferencing. Com-
puters in Human Behavior, 29(1):285–292.
Guo, W. W. (2010). Incorporating statistical and neural net-
work approaches for student course satisfaction anal-
ysis and prediction. Expert Systems with Applications,
37(4):3358–3365.
Guruler, H., Istanbullu, A., and Karahasan, M. (2010).
A new student performance analysing system using
knowledge discovery in higher educational databases.
Computers & Education, 55(1):247–254.
Halawa, S., Greene, D., and Mitchell, J. (2014). Dropout
prediction in MOOCs using learner activity features.
eLearning Papers, 37.
Hattie, J. (2015). The applicability of visible learning to
higher education. Scholarship of Teaching and Learn-
ing in Psychology, 1(1):79.
Hattie, J. and Timperley, H. (2007). The power of feedback.
Review of educational research, 77(1):81–112.
Heller, N. and Bry, F. (20-22 June 2018). Predicting learn-
ers’ behaviours to get it wrong. In Methodologies and
Intelligent Systems for Technology Enhanced Learn-
ing, 8th International Conference, Advances in Intelli-
gent Systems and Computing, pages 12–19. Springer.
Jovanovi
´
c, J., Ga
ˇ
sevi
´
c, D., Dawson, S., Pardo, A., and Mir-
riahi, N. (2017). Learning analytics to unveil learn-
ing strategies in a flipped classroom. The Internet and
Higher Education, 33(4):74–85.
Kerly, A., Ellis, R., and Bull, S. (2008). Calmsystem: a con-
versational agent for learner modelling. In Applica-
tions and Innovations in Intelligent Systems XV, pages
89–102. Springer.
Kizilcec, R. F., Piech, C., and Schneider, E. (2013). Decon-
structing disengagement: analyzing learner subpopu-
lations in massive open online courses. In Proceed-
ings of the third international conference on learning
analytics and knowledge, pages 170–179. ACM.
Lykourentzou, I., Giannoukos, I., Nikolopoulos, V., Mpar-
dis, G., and Loumos, V. (2009). Dropout prediction
in e-learning courses through the combination of ma-
chine learning techniques. Computers and Education,
53(3):950–965.
Merceron, A. and Yacef, K. (2008). Interestingness mea-
sures for association rules in educational data. In Ed-
ucational Data Mining 2008.
Oladokun, V., Adebanjo, A., and Charles-Owaba, O.
(2008). Predicting students’ academic performance
using artificial neural network: A case study of an en-
gineering course. The Pacific Journal of Science and
Technology, 9(1):72–79.
Onah, D. F., Sinclair, J., and Boyatt, R. (2014). Dropout
rates of massive open online courses: behavioural pat-
terns. EDULEARN14 proceedings, pages 5825–5834.
Onji, K. (2009). Procrastination, prompts, and prefer-
ences. Journal of Behavioral Economics and Finance,
2:111–113.
Pardos, Z. A., Baker, R. S., San Pedro, M. O., Gowda,
S. M., and Gowda, S. M. (2013). Affective states
and state tests: Investigating how affect throughout the
school year predicts end of year learning outcomes. In
Proceedings of the Third International Conference on
Learning Analytics and Knowledge, pages 117–124.
ACM.
Park, Y. and Jo, I.-H. (2015). Development of the learn-
ing analytics dashboard to support students’ learning
performance. J. UCS, 21(1):110–133.
Radatz, H. (1979). Error analysis in mathematics educa-
tion. Journal for Research in Mathematics Education,
pages 163–172.
Schumacher, C. and Ifenthaler, D. (2018). Features students
really expect from learning analytics. Computers in
human behavior, 78:397–407.
Siemens, G., editor (2010). 1st international conference on
learning analytics and knowledge 2011.
Tan, M. and Shao, P. (2015). Prediction of student dropout
in e-learning program through the use of machine
learning method. iJet, 10(1):11–17.
Tempelaar, D. T., Rienties, B., and Giesbers, B. (2015).
In search for the most informative data for feedback
generation: Learning analytics in a data-rich context.
Computers in Human Behavior, 47:157–167.
Trautwein, U. and K
¨
oller, O. (2003). The relationship be-
tween homework and achievement—still much of a
mystery. Educational psychology review, 15(2):115–
145.
Verbert, K., Duval, E., Klerkx, J., Govaerts, S., and Santos,
J. L. (2013). Learning analytics dashboard applica-
tions. American Behavioral Scientist, 57(10):1500–
1509.
Ye, C. and Biswas, G. (2014). Early prediction of student
dropout and performance in moocs using higher gran-
ularity temporal information. Journal of Learning An-
alytics, 1(3):169–172.
Zimmerman, B. J. and Kitsantas, A. (2005). Homework
practices and academic achievement: The mediat-
ing role of self-efficacy and perceived responsibil-
ity beliefs. Contemporary Educational Psychology,
30(4):397–417.
Nudging by Predicting: A Case Study
243