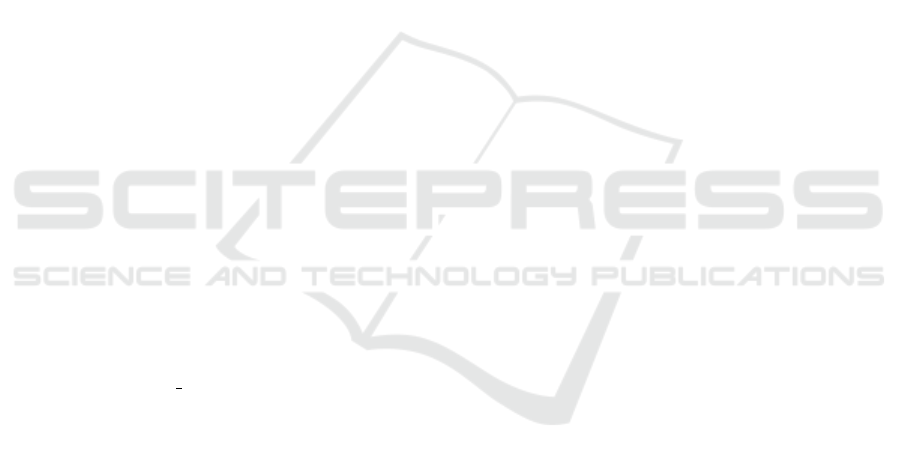
the semantic weights of relations in the real world.
This conversion step allows the Two-Stage-MR-BPR
to learn ranking edges based on their weights and to
learn to distinguish observed edges from unobserved
ones. Experiments on four real-world datasets sho-
wed that Two-Stage-MR-BPR outperformed the ori-
ginal MR-BPR and other state-of-art models in the
task of multi-label network classification and docu-
ment classification. In future work, we are planning to
explore and integrate several possible approaches to
further expand the capacity of the BPR models. Two
of those possible approaches for expansion are lear-
ning nonlinear latent representation and using non-
uniform samplers (Rendle and Freudenthaler, 2014).
ACKNOWLEDGEMENTS
The authors gratefully acknowledge the fun-
ding of their work through the
¨
UberDax project
(https://www.ismll.uni-hildesheim.de/projekte/
UberDax.html) which is sponsored by the Bundesmi-
nisterium fr Bildung und Forschung (BMBF) under
the grant agreement no. 01IS17053.
REFERENCES
Ahmed, C., ElKorany, A., and Bahgat, R. (2016). A super-
vised learning approach to link prediction in twitter.
Social Network Analysis and Mining, 6(1):24.
Breitkreutz, B.-J., Stark, C., Reguly, T., Boucher, L.,
Breitkreutz, A., Livstone, M., Oughtred, R., Lackner,
D. H., B
¨
ahler, J., Wood, V., et al. (2007). The bio-
grid interaction database: 2008 update. Nucleic acids
research, 36(suppl 1):D637–D640.
Cai, H., Zheng, V. W., and Chang, K. (2018). A compre-
hensive survey of graph embedding: problems, techni-
ques and applications. IEEE Transactions on Know-
ledge and Data Engineering.
Grover, A. and Leskovec, J. (2016). node2vec: Scalable
feature learning for networks. In Proceedings of the
22nd ACM SIGKDD international conference on Kno-
wledge discovery and data mining, pages 855–864.
ACM.
Jamali, M. and Ester, M. (2010). A matrix factorization
technique with trust propagation for recommendation
in social networks. In Proceedings of the fourth ACM
conference on Recommender systems, pages 135–142.
ACM.
Krohn-Grimberghe, A., Drumond, L., Freudenthaler, C.,
and Schmidt-Thieme, L. (2012). Multi-relational ma-
trix factorization using bayesian personalized ranking
for social network data. In Proceedings of the fifth
ACM international conference on Web search and
data mining, pages 173–182. ACM.
Lerche, L. and Jannach, D. (2014). Using graded implicit
feedback for bayesian personalized ranking. In Pro-
ceedings of the 8th ACM Conference on Recommen-
der systems, pages 353–356. ACM.
Liben-Nowell, D. and Kleinberg, J. (2007). The link-
prediction problem for social networks. journal of the
Association for Information Science and Technology,
58(7):1019–1031.
Mikolov, T., Chen, K., Corrado, G., and Dean, J. (2013).
Efficient estimation of word representations in vector
space. arXiv preprint arXiv:1301.3781.
Perozzi, B., Al-Rfou, R., and Skiena, S. (2014). Deepwalk:
Online learning of social representations. In Procee-
dings of the 20th ACM SIGKDD international confe-
rence on Knowledge discovery and data mining, pages
701–710. ACM.
Rendle, S. and Freudenthaler, C. (2014). Improving pair-
wise learning for item recommendation from implicit
feedback. In Proceedings of the 7th ACM internatio-
nal conference on Web search and data mining, pages
273–282. ACM.
Rendle, S., Freudenthaler, C., Gantner, Z., and Schmidt-
Thieme, L. (2009). Bpr: Bayesian personalized ran-
king from implicit feedback. In Proceedings of the
twenty-fifth conference on uncertainty in artificial in-
telligence, pages 452–461. AUAI Press.
Sen, P., Namata, G., Bilgic, M., Getoor, L., Galligher, B.,
and Eliassi-Rad, T. (2008). Collective classification in
network data. AI magazine, 29(3):93.
Singh, A. P. and Gordon, G. J. (2008). Relational learning
via collective matrix factorization. In Proceedings of
the 14th ACM SIGKDD international conference on
Knowledge discovery and data mining, pages 650–
658. ACM.
Symeonidis, P., Tiakas, E., and Manolopoulos, Y. (2010).
Transitive node similarity for link prediction in social
networks with positive and negative links. In Procee-
dings of the fourth ACM conference on Recommender
systems, pages 183–190. ACM.
Tang, J., Qu, M., Wang, M., Zhang, M., Yan, J., and Mei,
Q. (2015). Line: Large-scale information network
embedding. In Proceedings of the 24th International
Conference on World Wide Web, pages 1067–1077.
International World Wide Web Conferences Steering
Committee.
Tang, L. and Liu, H. (2009a). Relational learning via latent
social dimensions. In Proceedings of the 15th ACM
SIGKDD international conference on Knowledge dis-
covery and data mining, pages 817–826. ACM.
Tang, L. and Liu, H. (2009b). Scalable learning of col-
lective behavior based on sparse social dimensions. In
Proceedings of the 18th ACM conference on Informa-
tion and knowledge management, pages 1107–1116.
ACM.
Thomas, N. K. and Welling, M. (2016). Semi-supervised
classification with graph convolutional networks.
arxiv preprint. arXiv preprint arXiv:1609.02907, 103.
Tu, C., Zhang, W., Liu, Z., and Sun, M. (2016). Max-margin
deepwalk: Discriminative learning of network repre-
sentation. In IJCAI, pages 3889–3895.
Yang, C., Liu, Z., Zhao, D., Sun, M., and Chang, E. Y.
(2015). Network representation learning with rich text
information. In IJCAI, pages 2111–2117.
Zafarani, R. and Liu, H. (2009). Social computing data re-
pository at asu.
ICAART 2019 - 11th International Conference on Agents and Artificial Intelligence
366