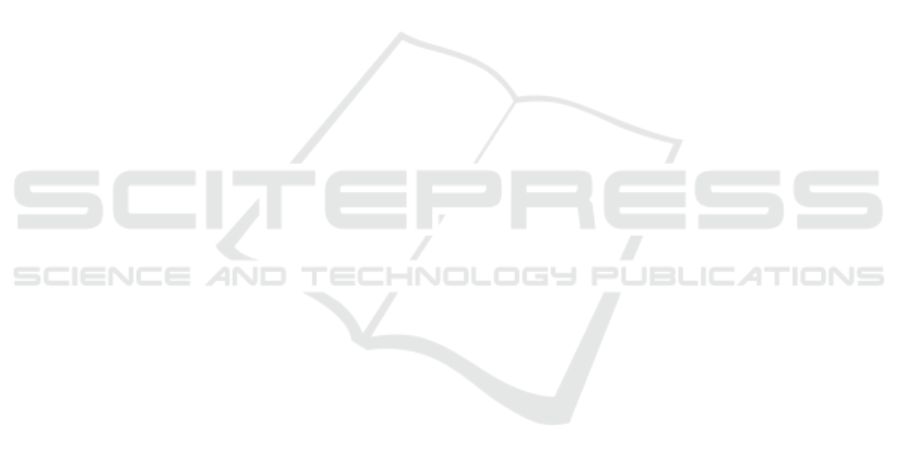
ACKNOWLEDGEMENTS
This paper is based on results obtained from a
project commissioned by the New Energy and Indus-
trial Technology Development Organization (NEDO).
CFD calculations were performed in collaboration
with Siemens Healthcare within a collaboration
agreement with the Jikei University.
REFERENCES
Amini, L., Azarpazhouh, R., Farzadfar, M., Mousavi, S.,
Jazaieri, F., Khorvash, F., Norouzi, R., and Toghianfar,
N. (2013). Prediction and control of stroke by data
mining. Int J Prev Med., 4(Suppl 2):S245–249.
Bentley, P., Ganesalingam, J., Jones, A., Mahady, K., Ep-
ton, S., Rinne, P., Sharma, P., Halse, O., Mehta, A.,
and Rueckert, D. (2014). Prediction of stroke throm-
bolysis outcome using ct brain machine learning. Neu-
roimage Clin., 30(4):635–640.
Chawla, N., Bowyer, K., Hall, L., and Kegelmeyer, W.
(2002). Smote: Synthetic minority over-sampling
technique. Journal of Artificial Intelligence Research,
16:321–357.
Chung, B. and Cebral, J. (2015). Cfd for evaluation and
treatment planning of aneurysms: review of proposed
clinical uses and their challenges. Ann Biomed Eng.,
43(1):122–138.
Hitman, G., Colhoun, H., Newman, C., Szarek, M., Bet-
teridge, D., Durrington, P., Fuller, J., Livingstone, S.,
Neil, H., and Investigators., C. (2007). Stroke predic-
tion and stroke prevention with atorvastatin in the col-
laborative atorvastatin diabetes study (cards). Diabet
Med., 24(12):1313–1321.
Letham, B., Rudin, C., McCormick, T., and Madigan, D.
(2015). Stroke prediction and stroke prevention with
atorvastatin in the collaborative atorvastatin diabetes
study (cards). Annals of Applied Statistics, 9(3):1350–
1371.
Lumley, T., Kronmal, R., Cushman, M., Manolio, T., and
Goldstein, S. (2002). A stroke prediction score in the
elderly: validation and web-based application. J Clin
Epidemiol, 55(2):129–136.
Manolio, T., Kronmal, R., Burke, G., O’Leary, D., and
Price, T. (1996). Short-term predictors of incident
stroke in older adults. Stroke, 27(9):1479–1486.
Morino, T., Tanoue, T., Tateshima, S., Vinuela, F., and Tan-
ishita, K. (2010). Intra-aneurysmal blood flow based
on patient-specific ct angiogram. Experiments in Flu-
ids, 49(2):485–496.
Nogueira, R., Bor-Seng-Shu, E., Saeed, N., Teixeira, M.,
Panerai, R., and Robinson, T. (2016). Meta-analysis of
vascular imaging features to predict outcome follow-
ing intravenous rtpa for acute ischemic stroke. Fron-
tiers in Neurology, 7(77):1–8.
Qian, Y., Takao, H., Umezu, M., and Murayama, Y. (2011).
Risk analysis of unruptured aneurysms using compu-
tational fluid dynamics technology: preliminary re-
sults. AJNR Am J Neuroradiol., 32(10):1948–1955.
Shojima, M., Oshima, M., Takagi, K., Torii, R., Hayakawa,
M., Katada, K., Morita, A., and Kirino, T. (2004).
Magnitude and role of wall shear stress on cere-
bral aneurysm: computational fluid dynamic study
of 20 middle cerebral artery aneurysms. Stroke,
35(11):2500–2505.
Takao, H., Murayama, Y., Abe, T., Ishibashi, T., Yuki, I.,
Otsuka, S., Suzuki, T., Masuda, S., Mohamed, A.,
Sen, I., Yamamoto, M., and Abe, T. (2012). Cfd re-
veals hemodynamic differences between unruptured
and ruptured intracranial aneurysms during observa-
tion. Stroke, 43(2):A2731.
Wang, T., Massaro, J., Levy, D., Vasan, R., Wolf, P.,
D’Agostino, R., Larson, M., Kannel, W., and Ben-
jamin, E. (2003). A risk score for predicting stroke
or death in individuals with new-onset atrial fibrilla-
tion in the community: the framingham heart study.
JAMA, 290(8):1049–1056.
Xiang, J., Siddiqui, A., and Meng, H. (2014). The effect of
inlet waveforms on computational hemodynamics of
patient-specific intracranial aneurysms. J Biomech.,
47(16):3882–3890.
Classification Model for Cerebral Aneurysm Rupture Prediction using Medical and Blood-flow-simulation Data
899