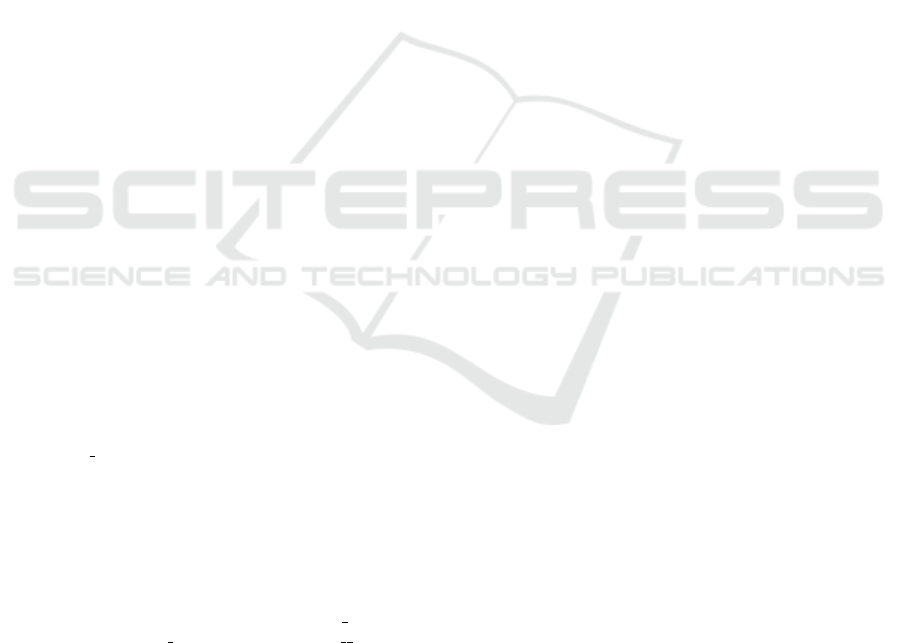
for action to help transform the conditional perceived
privacy into a fully perceived one.
ACKNOWLEDGEMENTS
This work has been funded by the Federal Ministry of
Transport and Digital Infrastructure (BMVI) within
the funding guideline ”Automated and Connected
Driving” under the grant number 16AVF2134B.
Many thanks go to Adam Michalik for research assis-
tance and the participants for sharing their opinions
on this matter. Also we like to thank the reviewer for
their feedback on this work.
REFERENCES
Bishop, R. (2000). Intelligent vehicle applications world-
wide. IEEE Intelligent Systems and Their Applica-
tions, 15(1):78–81.
Bonnefon, J.-F., Shariff, A., and Rahwan, I. (2016). The
Social dilemma of autonomous vehicles. Science,
352(6293):1573–1576.
Brell, T., Philipsen, R., and Ziefle, M. (2018). scary! risk
perceptions in autonomous driving: the influence of
experience on perceived benefits and barriers. Risk
analysis.
Breuer, J. J., Faulhaber, A., Frank, P., and Gleissner, S.
(2007). Real World Safety Benefits of Brake Assis-
tance Systems. In 20th International Technical Con-
ference on the Enhanced Safety of Vehicles (ESV).
Destatis (2018a). 17% of the population with academic de-
gree. https://www.destatis.de/EN/FactsFigures/
SocietyState/EducationResearchCulture/
EducationalLevel/AcademicDegree.html.
Destatis (2018b). Accidents and casualties cov-
ered. https://www.destatis.de/EN/FactsFigures/
EconomicSectors/TransportTraffic/TrafficAccidents/
Tables /RoadTrafficAccidents.html.
Destatis (2018c). Causes of accidents. https://www.
destatis.de/EN/FactsFigures/EconomicSectors/
TransportTraffic/TrafficAccidents/Tables/
DriverMistakes.html.
Destatis (2018d). Unfallentwicklung auf deutschen
Straßen [Accident development on German roads].
https://www.destatis.de/DE/PresseService/Presse/
Pressekonferenzen/2018/verkehrsunfaelle 2017/
Pressebroschuere unfallentwicklung.pdf? blob=
publicationFile.
Eichberger, A., Markovic, G., Magosi, Z., Rogic, B., Lex,
C., and Samiee, S. (2017). A Car2X sensor model for
virtual development of automated driving. Interna-
tional Journal of Advanced Robotic Systems, 14(5):1–
11.
Farmer, C. M. (2004). Effect of electronic stability control
on automobile crash risk. Traffic Injury Prevention,
5(4):317–325.
Haeuslschmid, R. and Buelow, M. V. (2017). Supporting
Trust in Autonomous Driving. In IUI 2017, pages
319–329.
Jung, J. C. and “Alison” Park, S. B. (2017). Case study:
Volkswagen’s diesel emissions scandal. Thunderbird
International Business Review, 59(1):127–137.
K
¨
onig, M. and Neumayr, L. (2017). Users’ resistance to-
wards radical innovations: The case of the self-driving
car. Transportation Research Part F, 44:42–52.
Kusano, K. D. and Gabler, H. C. (2012). Safety bene-
fits of forward collision warning, brake assist, and
autonomous braking systems in rear-end collisions.
IEEE Transactions on Intelligent Transportation Sys-
tems, 13(4):1546–1555.
Liu, J. F., Su, Y. F., Ko, M. K., and Yu, P. N. (2008).
Development of a vision-based driver assistance sys-
tem with lane departure warning and forward colli-
sion warning functions. In Techniques and Applica-
tions, 2008. DICTA’08. Digital Image, pages 480–
485. IEEE.
Neyer, F., Felber, J., and Gebhardt, C. (2016). Kurzskala
zur erfassung von technikbereitschaft (technology
commitment). In Zusammenstellung sozialwis-
senschaftlicher Items und Skalen.
Picone, M., Busanelli, S., Amoretti, M., Zanichelli, F., and
Ferrari, G. (2015). Advanced Technologies for Intel-
ligent Transportation Systems. Springer International
Publishing AG.
SAE (2016). Surface vehicle recommended practice - tax-
onomy and definitions for terms related to driving au-
tomation systems for on-road motor vehicles. J3016.
Scherping, R., St
¨
ahlin, U., and Bertram, T. (2013). Vehi-
cle2x data preprocessing for safety functions. In 5th
International Symposium on Wireless Vehicular Com-
munications (WiVeC). IEEE.
Schmidt, T., Philipsen, R., Themann, P., and Ziefle, M.
(2016a). Public perception of V2X-technology - Eval-
uation of general advantages, disadvantages and rea-
sons for data sharing with connected vehicles. In IEEE
Intelligent Vehicles Symposium (IV) Gothenburg, Swe-
den, June 19-22, pages 1344–1349.
Schmidt, T., Philipsen, R., and Ziefle, M. (2016b). Share
to protect. In International Conference on Human
Aspects of Information Security, Privacy, and Trust,
pages 152–163. Springer.
Schmidt, T., Philipsen, R., and Ziefle, M. (2016c). User
Diverse Privacy Requirements for V2X-Technology:
Quantitative Research on Context-Based Privacy As-
pects. In Proceedings of the International Conference
on Vehicle Technology and Intelligent Transport Sys-
tems (VEHITS 2016), pages 60–67.
Sharpe, N. F. (2017). Volkswagen’s bad decisions & harm-
ful emissions: How poor process corrupted codetermi-
nation in germany’s dual board structure. Mich. Bus.
& Entrepreneurial L. Rev., 7:49.
Trommer, S., Jarass, J., and Kolarova, V. (2015). Early
adopters of electric vehicles in germany unveiled.
World Electric Vehicle Journal, 7(4):722–732.
Vahidi, A. and Eskandarian, A. (2003). Research Ad-
vances in Intelligent Collision Avoidance and Adap-
VEHITS 2019 - 5th International Conference on Vehicle Technology and Intelligent Transport Systems
358