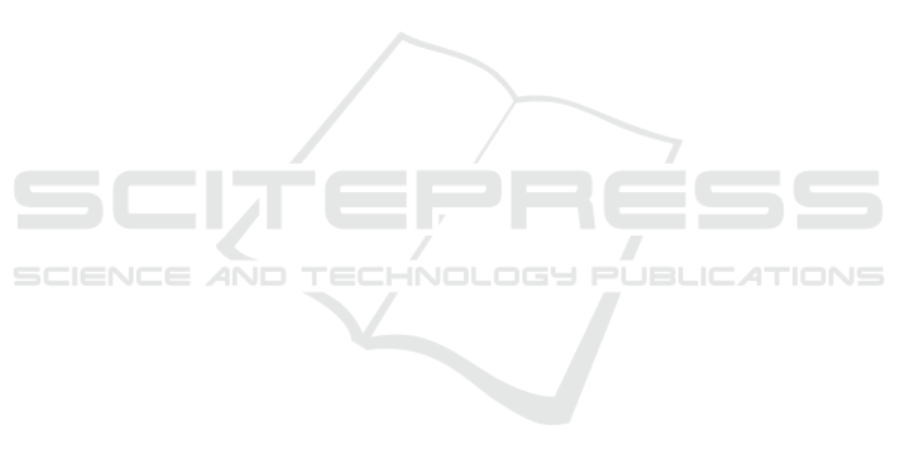
account for the majority of variances explained. In
sum, these results are useful for characterizing indi-
viduals’ motivational profiles especially, when lim-
ited access to subjects is assumed, and in cases where
subjects might be motivated to answer dishonestly to
direct questions. While the primary application of
these results is the CIRA method of risk analysis,
other domains could benefit from predicting inacces-
sible subject’s motivational profiles, especially where
decisions are characterized by trade-offs between var-
ious objectives and have great potential impact (e.g.
intelligence analysis, operations research, etc.). Fu-
ture work may expand the analysis to include other re-
gions of the world (e.g. USA, Eastern-cultures) to in-
vestigate whether the predictability of value profiles is
affected by deeper cultural differences. Finally, these
findings provide a solid benchmarking baseline for
other future work, which will investigate other classes
of observable features for inferring motivational pro-
files. More specifically, observables that represent the
outcome of a conscious decision process (e.g. own-
ership of items, style, etc.) will be analyzed in terms
of their capability to provide insight into the decision-
maker’s value structure.
ACKNOWLEDGEMENTS
The authors would like to thank the anonymous re-
viewers for their constructive comments and sugges-
tions for improving the paper.
This work was partially supported by the project
IoTSec – Security in IoT for Smart Grids, with
number 248113/O70 part of the IKTPLUSS program
funded by the Norwegian Research Council.
REFERENCES
Anderson, R. and Moore, T. (2009). Information security:
where computer science, economics and psychology
meet. Philosophical Transactions of the Royal Soci-
ety of London A: Mathematical, Physical and Engi-
neering Sciences, 367(1898):2717–2727.
Arora, J. (2017). Corporate governance: a farce at volkswa-
gen? The CASE Journal, 13(6):685–703.
Breiman, L. (2001). Random forests. Machine learning,
45(1):5–32.
Coos, A. (2018). Eu vs us: How do their data protection
regulations square off?
Dembe, A. E. and Boden, L. I. (2000). Moral hazard:
a question of morality? New Solutions: A Jour-
nal of Environmental and Occupational Health Policy,
10(3):257–279.
European Union (2016). Regulation (EU) 2016/679 of the
European Parliament and of the Council of 27 April
2016 on the protection of natural persons with re-
gard to the processing of personal data and on the
free movement of such data, and repealing Directive
95/46/EC (General Data Protection Regulation). Offi-
cial Journal of the European Union, L119:1–88.
Eurostat, N. (2008). Rev. 2–statistical classification of eco-
nomic activities in the european community. Office
for Official Publications of the European Communi-
ties, Luxemburg.
Forbes, D. L. (2011). Toward a unified model of human
motivation. Review of general psychology, 15(2):85.
Franklin, Z. (2014). Justice for revenge porn victims: Le-
gal theories to overcome claims of civil immunity by
operators of revenge porn websites. California Law
Review, pages 1303–1335.
Goldberg, L. R., Sweeney, D., Merenda, P. F., and
Hughes Jr, J. E. (1998). Demographic variables and
personality: The effects of gender, age, education, and
ethnic/racial status on self-descriptions of personal-
ity attributes. Personality and Individual differences,
24(3):393–403.
Grace-Martin, K. (2008). Assessing the fit of regression
models. [Online; accessed 05-July-2018].
Greitzer, F. L. and Hohimer, R. E. (2011). Modeling hu-
man behavior to anticipate insider attacks. Journal of
Strategic Security, 4(2):25–48.
Gudaitis, T. M. (1998). The missing link in information se-
curity: Three dimensional profiling. CyberPsychology
& Behavior, 1(4):321–340.
H2O.ai (2018a). Distributed Random Forest (DRF).
H2O.ai (2018b). H2O.ai.
Hvinden, B., Johanne Bang, K., Fjørtoft, K., Holand, I.,
Johnsen, R., Kolstad, I., Monsen, T., Nevøy, A.,
Sandmo, E., Skilbrei, M.-L., Staksrud, E., Tande,
K. M., Ulleberg, P., Øyum, L., and Enebakk, V.
(2016). Guidelines for research ethics in the social
sciences, humanities, law and theology.
Knafo, A. and Sagiv, L. (2004). Values and work environ-
ment: Mapping 32 occupations. European Journal of
Psychology of Education, 19(3):255–273.
Kosinski, M., Stillwell, D., and Graepel, T. (2013). Pri-
vate traits and attributes are predictable from digital
records of human behavior. Proceedings of the Na-
tional Academy of Sciences, page 201218772.
Krebs, B. (2012). Fbi: Smart meter hacks likely to spread.
[Online; accessed 26-June-2018].
Kulik, B. W., O’Fallon, M. J., and Salimath, M. S. (2008).
Do competitive environments lead to the rise and
spread of unethical behavior? parallels from enron.
Journal of Business Ethics, 83(4):703–723.
Liebowitz, S. J. and Margolis, S. E. (1994). Network exter-
nality: An uncommon tragedy. Journal of economic
perspectives, 8(2):133–150.
N.A. (2018). European social survey round 8 data. NSD -
Norwegian Centre for Research Data, Norway - Data
Archive and distributor of ESS data for ESS ERIC.
Nagelkerke, N. J. et al. (1991). A note on a general defi-
nition of the coefficient of determination. Biometrika,
78(3):691–692.
Patro, S. and Sahu, K. K. (2015). Normalization: A prepro-
cessing stage. arXiv preprint arXiv:1503.06462.
ICEIS 2019 - 21st International Conference on Enterprise Information Systems
386