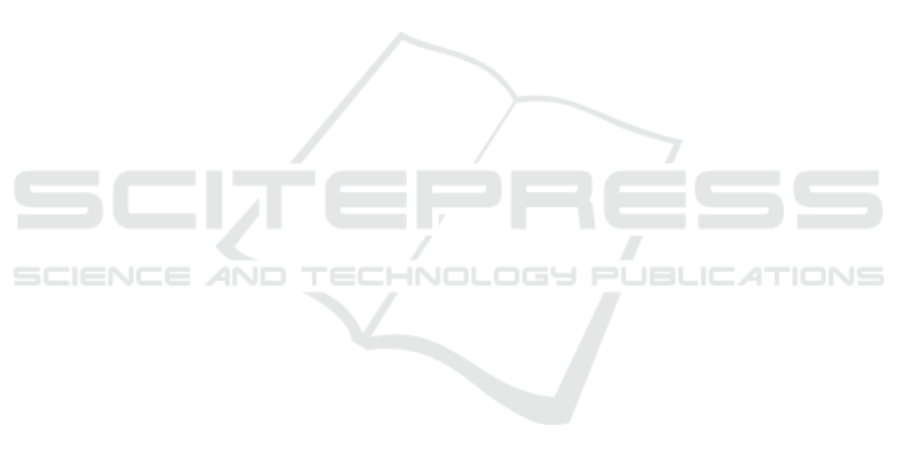
Ardagna, D., Ciavotta, M., Lancellotti, R., and Guerriero,
M. (2018). A hierarchical receding horizon algo-
rithm for qos-driven control of multi-iaas applications.
IEEE Transactions on Cloud Computing, pages 1–1.
Binitha, S., Sathya, S. S., et al. (2012). A survey of bio in-
spired optimization algorithms. International Journal
of Soft Computing and Engineering, 2(2):137–151.
Canali, C. and Lancellotti, R. (2017). Scalable and auto-
matic virtual machines placement based on behavioral
similarities. Computing, 99(6):575–595.
Cardellini, V., Grassi, V., Lo Presti, F., and Nardelli, M.
(2016). Optimal operator placement for distributed
stream processing applications. In Proceedings of the
10th ACM International Conference on Distributed
and Event-based Systems, DEBS ’16, pages 69–80,
New York, NY, USA. ACM.
DEAP (2018). DEAP: Distributed Evolutionary Algorithms
in Pyton. – https://deap.readthedocs.io.
Deng, R., Lu, R., Lai, C., Luan, T. H., and Liang, H. (2016).
Optimal Workload Allocation in Fog-Cloud Comput-
ing Toward Balanced Delay and Power Consumption.
IEEE Internet of Things Journal, 3(6):1171–1181.
Duan, H., Chen, C., Min, G., and Wu, Y. (2017). Energy-
aware scheduling of virtual machines in heteroge-
neous cloud computing systems. Future Generation
Computer Systems, 74:142 – 150.
Karimi, M. B., Isazadeh, A., and Rahmani, A. M. (2017).
Qos-aware service composition in cloud computing
using data mining techniques and genetic algorithm.
J. Supercomput., 73(4):1387–1415.
Liu, J., Li, J., Zhang, L., Dai, F., Zhang, Y., Meng, X., and
Shen, J. (2018). Secure intelligent traffic light control
using fog computing. Future Generation Computer
Systems, 78:817 – 824.
Noshy, M., Ibrahim, A., and Ali, H. (2018). Optimization
of live virtual machine migration in cloud computing:
A survey and future directions. Journal of Network
and Computer Applications, 110:1–10.
Sasaki, K., Suzuki, N., Makido, S., and Nakao, A. (2016).
Vehicle control system coordinated between cloud and
mobile edge computing. In 2016 55th Annual Confer-
ence of the Society of Instrument and Control Engi-
neers of Japan (SICE), pages 1122–1127.
Shojafar, M., Canali, C., and Lancellotti, R. (2017). A
Computation- and Network-Aware Energy Optimiza-
tion Model for Virtual Machines Allocation. In Proc.
of International Conference on Cloud Computing and
Services Science (CLOSER 2017), Porto, Portugal.
Tang, B., Chen, Z., Hefferman, G., Wei, T., He, H., and
Yang, Q. (2015). A hierarchical distributed fog com-
puting architecture for big data analysis in smart cities.
In Proceedings of the ASE BigData & SocialInfor-
matics 2015, ASE BD&SI ’15, pages 28:1–28:6, New
York, NY, USA. ACM.
Trafair Project Staff (2019). Forecast of the impact by lo-
cal emissions at an urban micro scale by the combi-
nation of lagrangian modelling and low cost sensing
technology: the trafair project. In Proc. of 19th In-
ternational conference on Harmionisation within At-
mospheric Dispersion Modelling for Regulatory Pur-
poses, Bruges, Belgium.
Wen, Z., Yang, R., Garraghan, P., Lin, T., Xu, J., and Rovat-
sos, M. (2017). Fog orchestration for internet of things
services. IEEE Internet Computing, 21(2):16–24.
Yi, S., Li, C., and Li, Q. (2015). A survey of fog comput-
ing: Concepts, applications and issues. In Proceed-
ings of the 2015 Workshop on Mobile Big Data, Mo-
bidata ’15, pages 37–42, New York, NY, USA. ACM.
Yousefpour, A., Ishigaki, G., and Jue, J. P. (2017). Fog
computing: Towards minimizing delay in the internet
of things. In 2017 IEEE International Conference on
Edge Computing (EDGE), pages 17–24.
Yusoh, Z. I. M. and Tang, M. (2010). A penalty-based
genetic algorithm for the composite saas placement
problem in the cloud. In IEEE Congress on Evolu-
tionary Computation, pages 1–8.
A Fog Computing Service Placement for Smart Cities based on Genetic Algorithms
89