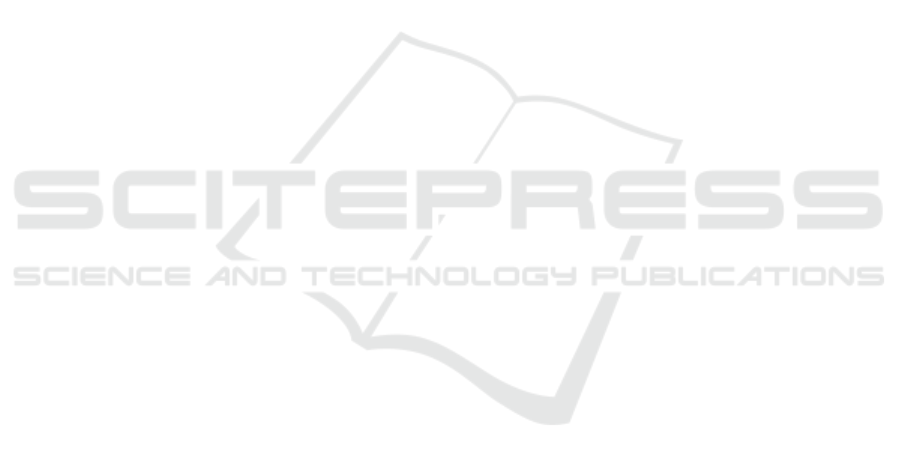
stage of signal acquisition, through biosensors, Ana-
log/Digital conversion through the Arduino, digital
signal transmission executed by a mobile applica-
tion, to the stage of ECG feature and data extrac-
tion, integrating and publishing, through Web Service
technology. Additionally, a collaborative database
is maintained to integrate and store ECG data com-
ing from heterogeneous sources and data on patients,
treatments, and drugs. The database is published us-
ing Linked Data standards. A case study was ana-
lyzed aiming at to demonstrate MobileECG proper-
ties. As future work, anonymizing patient data tech-
niques should be implemented, aiming at the protec-
tion of individual information. Moreover, machine
learning algorithms will be deployed for classifica-
tion/recognition of arrhythmia and other events.
ACKNOWLEDGEMENTS
Sources of funding: The authors acknowledge the
supports of the Brazilian Research Council, CNPq
(Grant n. 426002/2016-4), and Cear
´
a State Founda-
tion for the Support of Scientific and Technological
Development (BP3-0139-00284.01.00/18).
REFERENCES
Bond, R. R., Finlay, D. D., Nugent, C. D., and Moore, G.
(2011). A review of ECG storage formats. Int. journal
of medical informatics, 80(10):681–697.
da Silva, H. P., Carreiras, C., Lourenc¸o, A., Fred, A.,
das Neves, R. C., and Ferreira, R. (2015). Off-
the-person electrocardiography: performance assess-
ment and clinical correlation. Health and Technology,
4(4):309–318.
Daar, A. S., Singer, P. A., Persad, D. L., Pramming,
S. K., Matthews, D. R., Beaglehole, R., Bernstein,
A., Borysiewicz, L. K., Colagiuri, S., Ganguly, N.,
et al. (2007). Grand challenges in chronic non-
communicable diseases. Nature, 450(7169):494–496.
do Vale Madeiro, J. P., dos Santos, E. M. B. E., Cortez, P. C.,
da Silva Felix, J. H., and Schlindwein, F. S. (2017).
Evaluating gaussian and rayleigh-based mathematical
models for t and p-waves in ecg. IEEE Latin America
Transactions, 15(5):843–853.
Elhaj, F. A., Salim, N., Harris, A. R., Swee, T. T., and
Ahmed, T. (2016). Arrhythmia recognition and classi-
fication using combined linear and nonlinear features
of ecg signals. Computer methods and programs in
biomedicine, 127:52–63.
Goldberger, A. L., Amaral, L. A., Glass, L., Hausdorff,
J. M., Ivanov, P. C., Mark, R. G., Mietus, J. E., Moody,
G. B., Peng, C.-K., and Stanley, H. E. (2000a). Phys-
iobank, physiotoolkit, and physionet. Circulation,
101(23):e215–e220.
Goldberger, A. L., Amaral, L. A., Glass, L., Hausdorff,
J. M., Ivanov, P. C., Mark, R. G., Mietus, J. E., Moody,
G. B., Peng, C.-K., and Stanley, H. E. (2000b). Phys-
iobank, physiotoolkit, and physionet. Circulation,
101(23):e215–e220.
Gonc¸alves, B., Guizzardi, G., and Pereira Filho, J. G.
(2007). An electrocardiogram (ECG) domain ontol-
ogy. In Workshop on Ontologies and Metamodels for
Software and Data Engineering, Jo
˜
ao Pessoa, Brazil.
Gonc¸alves, B., Guizzardi, G., and Pereira Filho, J. G.
(2011). Using an ecg reference ontology for seman-
tic interoperability of ecg data. Journal of Biomedical
Informatics, 44(1):126–136.
Islam, M., Tangim, G., Ahammad, T., Khondokar, M., et al.
(2012). Study and analysis of ecg signal using matlab
&labview as effective tools. International journal of
Computer and Electrical engineering, 4(3):404.
Luz, E. J. d. S., Schwartz, W. R., C
´
amara-Ch
´
avez, G., and
Menotti, D. (2016). Ecg-based heartbeat classification
for arrhythmia detection: A survey. Computer meth-
ods and programs in biomedicine, 127:144–164.
Madeiro, J. P., Cortez, P. C., Marques, J. A., Seisdedos,
C. R., and Sobrinho, C. R. (2012). An innovative ap-
proach of QRS segmentation based on first-derivative,
hilbert and wavelet transforms. Medical engineering
& physics, 34(9):1236–1246.
Mandellos, G. J., Koukias, M. N., Styliadis, I. S., and Lym-
beropoulos, D. K. (2010). e-scp-ecg+ protocol: An
expansion on SCP-ECG protocol for health telemon-
itoring—pilot implementation. International journal
of telemedicine and applications, 2010:1.
Martis, R. J., Acharya, U. R., and Adeli, H. (2014). Current
methods in electrocardiogram characterization. Com-
puters in biology and medicine, 48:133–149.
Ngo, D. and Veeravalli, B. (2014). Applied semantic tech-
nologies in ecg interpretation and cardiovascular di-
agnosis. In Bioinformatics and Biomedicine (BIBM),
2014 IEEE Int. Conf. on, pages 17–24. IEEE.
Orwat, C., Graefe, A., and Faulwasser, T. (2008). Towards
pervasive computing in health care – a literature re-
view. BMC Medical Informatics and Decision Mak-
ing, 8(1):26.
Priyatna, F., Corcho, O., and Sequeda, J. (2014). Formal-
isation and experiences of r2rml-based sparql to sql
query translation using morph. In Proc. of the 23rd
Int. Conf. on World wide web, pages 479–490. ACM.
Venkatachalam, K., Herbrandson, J. E., and Asirvatham,
S. J. (2011). Signals and signal processing for the
electrophysiologist. Circulation: Arrhythmia and
Electrophysiology, 4(6):965–973.
Wen, C., Yeh, M.-F., Chang, K.-C., and Lee, R.-G. (2008).
Real-time ecg telemonitoring system design with mo-
bile phone platform. Measurement, 41(4):463–470.
Worringham, C., Rojek, A., and Stewart, I. (2011). Devel-
opment and feasibility of a smartphone, ecg and gps
based system for remotely monitoring exercise in car-
diac rehabilitation. PloS one, 6(2):e14669.
ICEIS 2019 - 21st International Conference on Enterprise Information Systems
586