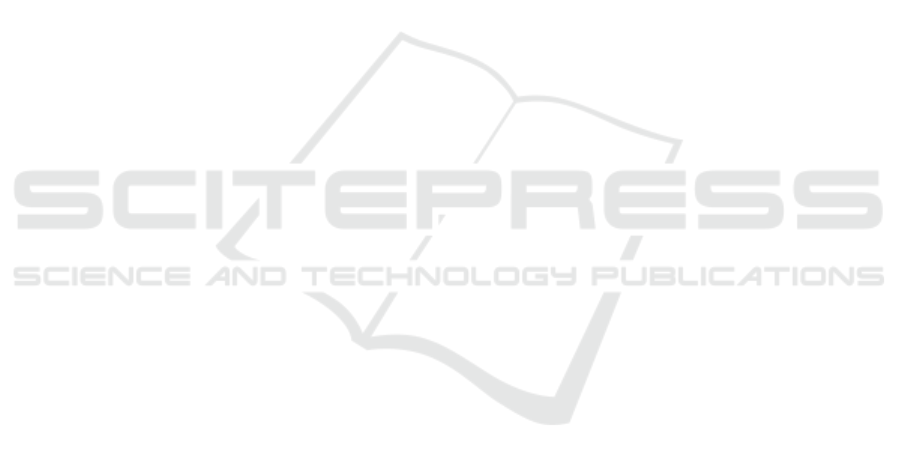
location information. Moreover, we believe that by
adding global context constraint to other FCN exten-
sion networks, better result can be achieved.
5 CONCLUSIONS
In this work, we propose the global context constraint
network, which allows the direct inclusion of global
semantic context constraint for the task of seman-
tic segmentation. We have explicitly demonstrated
that relying on constrained global context features can
largely improve the segmentation result and eliminate
semantic segmentation confusion because global con-
text constraint loss explicitly predicts the global con-
text information that merged into the final encoded
feature. The result presented on PASCAL VOC 2012
dataset shows that our approach can also reach the
state-of-the-art performance at the same training con-
ditions and its simplicity and robustness of learning
makes it more advantageous.
ACKNOWLEDGEMENTS
This work was supported by Institute for Informa-
tion & communications Technology Promotion(IITP)
grant funded by the Korea government(MSIT) (No.
R7117-16-0164, Development of wide area driving
environment awareness and cooperative driving tech-
nology which are based on V2X wireless communi-
cation.
REFERENCES
Badrinarayanan, V., Kendall, A., and Cipolla, R.
(2015). Segnet: A deep convolutional encoder-
decoder architecture for image segmentation. volume
abs/1511.00561.
Chen, L., Papandreou, G., Kokkinos, I., Murphy, K., and
Yuille, A. L. (2014). Semantic image segmentation
with deep convolutional nets and fully connected crfs.
volume abs/1412.7062.
Everingham, M., Gool, L., Williams, C. K., Winn, J., and
Zisserman, A. (2010). The pascal visual object classes
(voc) challenge. Int. J. Comput. Vision, 88(2):303–
338.
Glorot, X. and Bengio, Y. (2010). Understanding the dif-
ficulty of training deep feedforward neural networks.
In Proceedings of the Thirteenth International Con-
ference on Artificial Intelligence and Statistics, pages
249–256.
Hariharan, B., Arbelaez, P., Bourdev, L., Maji, S., and Ma-
lik, J. (2011). Semantic contours from inverse detec-
tors. In International Conference on Computer Vision
(ICCV).
He, K., Gkioxari, G., Doll
´
ar, P., and Girshick, R. (2017).
Mask R-CNN. In Proceedings of the International
Conference on Computer Vision (ICCV).
He, K., Zhang, X., Ren, S., and Sun, J. (2016). Deep resid-
ual learning for image recognition. In 2016 IEEE Con-
ference on Computer Vision and Pattern Recognition
(CVPR), pages 770–778.
Hong, S., Noh, H., and Han, B. (2015). Decoupled deep
neural network for semi-supervised semantic segmen-
tation. In Proceedings of the 28th International Con-
ference on Neural Information Processing Systems,
NIPS’15, pages 1495–1503, Cambridge, MA, USA.
MIT Press.
Hu, H., Lan, S., Jiang, Y., Cao, Z., and Sha, F. (2017). Fast-
mask: Segment multi-scale object candidates in one
shot. In 2017 IEEE Conference on Computer Vision
and Pattern Recognition, CVPR 2017, Honolulu, HI,
USA, July 21-26, 2017, pages 2280–2288.
Jia, Y., Shelhamer, E., Donahue, J., Karayev, S., Long, J.,
Girshick, R., Guadarrama, S., and Darrell, T. (2014).
Caffe: Convolutional architecture for fast feature em-
bedding. In Proceedings of the 22Nd ACM Inter-
national Conference on Multimedia, MM ’14, pages
675–678, New York, NY, USA. ACM.
Kr
¨
ahenb
¨
uhl, P. and Koltun, V. (2011). Efficient inference in
fully connected crfs with gaussian edge potentials. In
Shawe-Taylor, J., Zemel, R. S., Bartlett, P. L., Pereira,
F., and Weinberger, K. Q., editors, Advances in Neural
Information Processing Systems 24, pages 109–117.
Curran Associates, Inc.
Lin, T.-Y., Maire, M., Belongie, S., Hays, J., Perona, P.,
Ramanan, D., Doll
´
ar, P., and Zitnick, C. L. (2014).
Microsoft coco: Common objects in context. In Fleet,
D., Pajdla, T., Schiele, B., and Tuytelaars, T., editors,
Computer Vision – ECCV 2014: 13th European Con-
ference, Zurich, Switzerland, September 6-12, 2014,
Proceedings, Part V, pages 740–755, Cham. Springer
International Publishing.
Liu, W., Anguelov, D., Erhan, D., Szegedy, C., Reed, S. E.,
Fu, C., and Berg, A. C. (2016). SSD: single shot multi-
box detector. In Computer Vision - ECCV 2016 - 14th
European Conference, Amsterdam, The Netherlands,
October 11-14, 2016, Proceedings, Part I, pages 21–
37.
Liu, W., Rabinovich, A., and Berg, A. C. (2015). Parsenet:
Looking wider to see better. volume abs/1506.04579.
Long, J., Shelhamer, E., and Darrell, T. (2015). Fully con-
volutional networks for semantic segmentation. In
The IEEE Conference on Computer Vision and Pat-
tern Recognition (CVPR).
Mottaghi, R., Chen, X., Liu, X., Cho, N.-G., Lee, S.-W.,
Fidler, S., Urtasun, R., and Yuille, A. (2014). The
role of context for object detection and semantic seg-
mentation in the wild. In The IEEE Conference on
Computer Vision and Pattern Recognition (CVPR).
Noh, H., Hong, S., and Han, B. (2015). Learning decon-
volution network for semantic segmentation. In Pro-
ceedings of the 2015 IEEE International Conference
VEHITS 2019 - 5th International Conference on Vehicle Technology and Intelligent Transport Systems
386