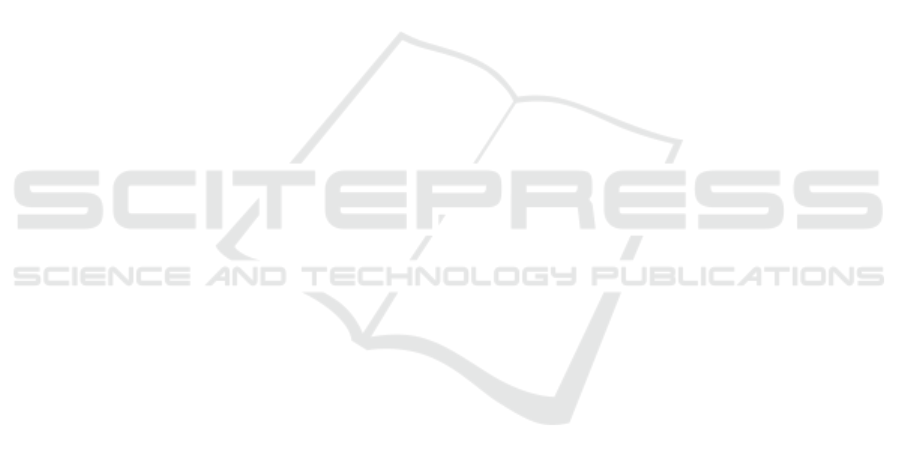
Bavarian Ministry of Living, Building and
Transportation.
REFERENCES
Canziani, A., Paszke, A., Culurciello, E., 2016. An
Analysis of Deep Neural Network Models for
Practical Applications. arXiv:1605.07678 [cs].
de la Escalera, A., Moreno, L.E., Salichs, M.A., Armingol,
J.M., 1997. Road traffic sign detection and
classification. IEEE Transactions on Industrial
Electronics 44, 848–859.
https://doi.org/10.1109/41.649946
Fang, C.-Y., Chen, S.-W., Fuh, C.-S., 2003. Road-sign
detection and tracking. IEEE Transactions on
Vehicular Technology 52, 1329–1341.
https://doi.org/10.1109/TVT.2003.810999
Garriga, N., Franch, M., Santos, X., Montori, A., Llorente,
G.A., 2017. Seasonal variation in vertebrate traffic
casualties and its implications for mitigation measures.
Landscape and Urban Planning 157, 36–44.
https://doi.org/10.1016/j.landurbplan.2016.05.029
Greenhalgh, J., Mirmehdi, M., 2012. Real-Time Detection
and Recognition of Road Traffic Signs. IEEE
Transactions on Intelligent Transportation Systems 13,
1498–1506.
https://doi.org/10.1109/TITS.2012.2208909
Hothorn, T., Müller, J., Held, L., Möst, L., Mysterud, A.,
2015. Temporal patterns of deer–vehicle collisions
consistent with deer activity pattern and density
increase but not general accident risk. Accident
Analysis & Prevention 81, 143–152.
https://doi.org/10.1016/j.aap.2015.04.037
Huijser, M.P., Fairbank, E.R., Camel-Means, W., Graham,
J., Watson, V., Basting, P., Becker, D., 2016.
Effectiveness of short sections of wildlife fencing and
crossing structures along highways in reducing
wildlife–vehicle collisions and providing safe crossing
opportunities for large mammals. Biological
Conservation 197, 61–68.
https://doi.org/10.1016/j.biocon.2016.02.002
Krizhevsky, A., Sutskever, I., Hinton, G.E., 2017.
ImageNet classification with deep convolutional
neural networks. Communications of the ACM 60,
84–90. https://doi.org/10.1145/3065386
Kruuse, M., Enno, S.-E., Oja, T., 2016. Temporal patterns
of wild boar-vehicle collisions in Estonia, at the
northern limit of its range. European Journal of
Wildlife Research 62, 787–791.
https://doi.org/10.1007/s10344-016-1042-9
Lagunas, M., Garces, E., 2018. Transfer Learning for
Illustration Classification. arXiv:1806.02682 [cs, stat].
https://doi.org/10.2312/ceig.20171213
Obaidat, M.T., Al-kheder, S.A., 2006. Integration of
geographic information systems and computer vision
systems for pavement distress classification.
Construction and Building Materials 20, 657–672.
https://doi.org/10.1016/j.conbuildmat.2005.02.009
Šegvic, S., Brkić, K., Kalafatić, Z., Stanisavljević, V.,
Ševrović, M., Budimir, D., Dadić, I., 2010. A
computer vision assisted geoinformation inventory for
traffic infrastructure, in: 13th International IEEE
Conference on Intelligent Transportation Systems.
Presented at the 13th International IEEE Conference
on Intelligent Transportation Systems, pp. 66–73.
https://doi.org/10.1109/ITSC.2010.5624979
Villalva, P., Reto, D., Santos-Reis, M., Revilla, E., Grilo,
C., 2013. Do dry ledges reduce the barrier effect of
roads? Ecological Engineering 57, 143–148.
https://doi.org/10.1016/j.ecoleng.2013.04.005
Vitabile, S., Gentile, A., Sorbello, F., 2002. A neural
network based automatic road signs recognizer, in:
Proceedings of the 2002 International Joint
Conference on Neural Networks. IJCNN’02 (Cat.
No.02CH37290). IEEE, Honolulu, HI, USA, pp.
2315–2320.
https://doi.org/10.1109/IJCNN.2002.1007503
Wu, Y., Liu, Y., Li, J., Liu, H., Hu, X., 2013. Traffic sign
detection based on convolutional neural networks, in:
The 2013 International Joint Conference on Neural
Networks (IJCNN). IEEE, Dallas, TX, USA, pp. 1–7.
https://doi.org/10.1109/IJCNN.2013.6706811
GISTAM 2019 - 5th International Conference on Geographical Information Systems Theory, Applications and Management
76