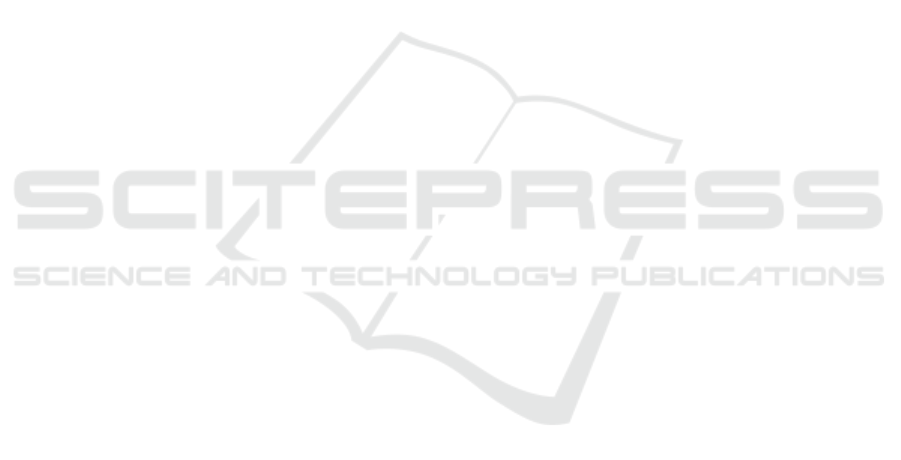
ference on Acoustics, Speech, and Signal Processing,
ICASSP 2004, Montreal, Quebec, Canada, May 17-
21, 2004, pages 521–524.
Bouguila, N. and Ziou, D. (2004b). A powerful finite
mixture model based on the generalized dirichlet dis-
tribution: Unsupervised learning and applications.
In 17th International Conference on Pattern Recog-
nition, ICPR 2004, Cambridge, UK, August 23-26,
2004., pages 280–283.
Bouguila, N. and Ziou, D. (2006). A hybrid SEM algorithm
for high-dimensional unsupervised learning using a fi-
nite generalized dirichlet mixture. IEEE Trans. Image
Processing, 15(9):2657–2668.
Bouguila, N. and Ziou, D. (2012). A countably infinite mix-
ture model for clustering and feature selection. Knowl.
Inf. Syst., 33(2):351–370.
Boutemedjet, S., Ziou, D., and Bouguila, N. (2007). Unsu-
pervised feature selection for accurate recommenda-
tion of high-dimensional image data. In Advances in
Neural Information Processing Systems 20, Proceed-
ings of the Twenty-First Annual Conference on Neural
Information Processing Systems, Vancouver, British
Columbia, Canada, December 3-6, 2007, pages 177–
184.
Cantner, U., Kr
¨
uger, J. J., and S
¨
ollner, R. (2012). Product
quality, product price, and share dynamics in the ger-
man compact car market. Industrial and Corporate
Change, 21(5):1085–1115.
Carri
`
ere-Swallow, Y. and Labb
´
e, F. (2013). Nowcasting
with google trends in an emerging market. Journal
of Forecasting, 32(4):289–298.
Chang, W.-Y., Gupta, R. D., and Richards, D. S. P. (2010).
Structural properties of the generalized dirichlet dis-
tributions. Contemp. Math, 516:109–124.
Choi, H. and Varian, H. (2012). Predicting the present with
google trends. Economic Record, 88:2–9.
Draper, N. R. and Smith, H. (2014). Applied regression
analysis, volume 326. John Wiley & Sons.
Dussauge, P., Garrette, B., Mitchell, W., et al. (2002). The
market-share impact of inter-partner learning in al-
liances: evidence from the global auto industry. Co-
operative strategies and alliances, pages 707–727.
Fan, W. and Bouguila, N. (2013a). Online learning of
a dirichlet process mixture of beta-liouville distribu-
tions via variational inference. IEEE transactions on
neural networks and learning systems, 24(11):1850–
1862.
Fan, W. and Bouguila, N. (2013b). Variational learning of a
dirichlet process of generalized dirichlet distributions
for simultaneous clustering and feature selection. Pat-
tern Recognition, 46(10):2754–2769.
Fan, W., Bouguila, N., and Ziou, D. (2011). Unsupervised
anomaly intrusion detection via localized bayesian
feature selection. In 11th IEEE International Confer-
ence on Data Mining, ICDM 2011, Vancouver, BC,
Canada, December 11-14, 2011, pages 1032–1037.
Fan, W., Bouguila, N., and Ziou, D. (2013). Unsuper-
vised hybrid feature extraction selection for high-
dimensional non-gaussian data clustering with vari-
ational inference. IEEE Trans. Knowl. Data Eng.,
25(7):1670–1685.
Ferrari, S. and Cribari-Neto, F. (2004). Beta regression for
modelling rates and proportions. Journal of Applied
Statistics, 31(7):799–815.
Fox, J. and Monette, G. (2002). An R and S-Plus companion
to applied regression. Sage.
Haaf, C. G., Michalek, J. J., Morrow, W. R., and Liu, Y.
(2014). Sensitivity of vehicle market share predictions
to discrete choice model specification. Journal of Me-
chanical Design, 136(12):121402.
Hijazi, R. H. (2006). Residuals and diagnostics in dirichlet
regression. ASA Proceedings of the General Method-
ology Section, pages 1190–1196.
Hijazi, R. H. and Jernigan, R. W. (2009). Modelling compo-
sitional data using dirichlet regression models. Jour-
nal of Applied Probability & Statistics, 4(1):77–91.
Hutcheson, G. D. (2011). Ordinary least-squares regres-
sion. The SAGE Dictionary of Quantitative Manage-
ment Research, pages 224–228.
Kullback, S. and Leibler, R. A. (1951). On information
and sufficiency. The annals of mathematical statistics,
22(1):79–86.
Lancaster, H. (1965). The helmert matrices. The American
Mathematical Monthly, 72(1):4–12.
Maier, M. J. (2014). Dirichletreg: Dirichlet regression for
compositional data in r.
Martin-Fernandez, J. A., Bren, M., Barcelo-Vidal, C., and
Pawlowsky Glahn, V. (1999). A measure of differ-
ence for compositional data based on measures of di-
vergence. Proceedings of IAMG, 99:211–216.
Morais, J., Thomas-Agnan, C., and Simioni, M. (2018).
Using compositional and dirichlet models for mar-
ket share regression. Journal of Applied Statistics,
45(9):1670–1689.
Moraisab, J., Thomas-Agnana, C., and Simionic, M.
(2016). A tour of regression models for explaining
shares.
Tay, R. S. and Mc Carthy, P. S. (1991). Demand ori-
ented policies for improving market share in the us
automobile industry. International Journal of Trans-
port Economics/Rivista internazionale di economia
dei trasporti, pages 151–166.
Tsagris, M. (2015). Regression analysis with composi-
tional data containing zero values. arXiv preprint
arXiv:1508.01913.
Tsagris, M. and Stewart, C. (2018). A dirichlet re-
gression model for compositional data with zeros.
Lobachevskii Journal of Mathematics, 39(3):398–
412.
Vosen, S. and Schmidt, T. (2011). Forecasting private con-
sumption: survey-based indicators vs. google trends.
Journal of Forecasting, 30(6):565–578.
Watt, J., Borhani, R., and Katsaggelos, A. K. (2016). Ma-
chine Learning Refined: Foundations, Algorithms,
and Applications. Cambridge University Press.
Zhang, Y., Zhou, H., Zhou, J., and Sun, W. (2017). Regres-
sion models for multivariate count data. Journal of
Computational and Graphical Statistics, 26(1):1–13.
ICEIS 2019 - 21st International Conference on Enterprise Information Systems
166