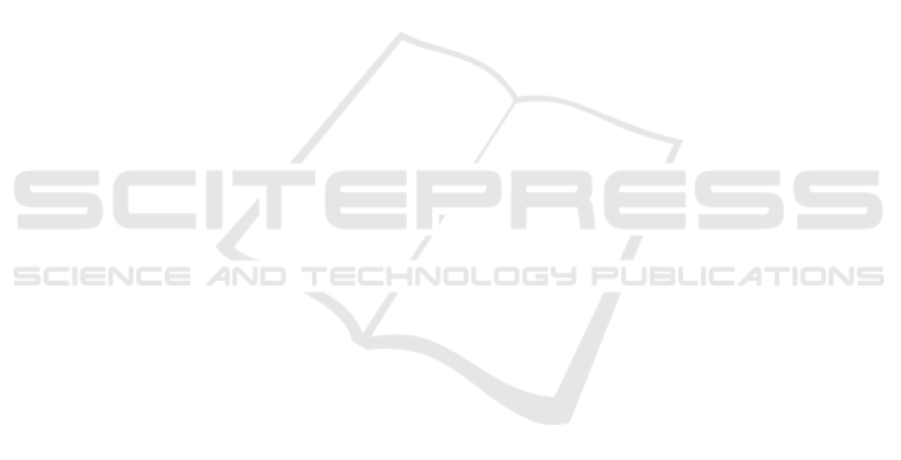
est results with an accuracy of 83.40% which is fol-
lowed by audio modality that achieves an accuracy of
75.19%. The least results are obtained when using
textual modality with an accuracy of 67.18%. Com-
bining the standalone modalities leads to improve the
results significantly in all cases using bimodal fusion
and trimodal fusion. The highest results of LR are ob-
tained from the combination of the three modalities
with an accuracy of 90.27%.
Overall, the highest accuracy of 91.6% is obtained
when using audio-visual support vector recognition
system.
4 CONCLUSIONS
Joint recognition of gender and sentiment polarity is
addressed in this paper as a multi-class classification
problem. A video corpus of Arabic speakers is col-
lected and processed. Two machine learning classi-
fiers are evaluated using various modalities. Features
are extracted and evaluated individually and after fu-
sion. The experimental work using 10-fold cross val-
idation showed that significant improvements can be
achieved when combining modalities and using a sup-
port vector machine classifier. As future work, we
suggest exploring parameter optimization and deep
learning approaches to further improve the results.
ACKNOWLEDGEMENTS
The authors would like to acknowledge the sup-
port provided by the Deanship of Scientific Research
at King Fahd University of Petroleum & Minerals
(KFUPM) during this work.
REFERENCES
Abouelenien, M., P
´
erez-Rosas, V., Mihalcea, R., and
Burzo, M. (2017). Multimodal gender detection. In
Proceedings of the 19th ACM International Confer-
ence on Multimodal Interaction, pages 302–311.
Al-Azani, S. and El-Alfy, E.-S. M. (2017). Using word em-
bedding and ensemble learning for highly imbalanced
data sentiment analysis in short arabic text. Procedia
Computer Science, 109:359–366.
Al-Azani, S. and El-Alfy, E.-S. M. (2018). Combining emo-
jis with arabic textual features for sentiment classifica-
tion. In 9th IEEE International Conference on Infor-
mation and Communication Systems (ICICS), pages
139–144.
Alexandre, L. A. (2010). Gender recognition: A multiscale
decision fusion approach. Pattern recognition letters,
31(11):1422–1427.
Carcagn
`
ı, P., Coco, M., Leo, M., and Distante, C. (2015).
Facial expression recognition and histograms of ori-
ented gradients: a comprehensive study. Springer-
Plus, 4(1):645.
Cellerino, A., Borghetti, D., and Sartucci, F. (2004). Sex
differences in face gender recognition in humans.
Brain research bulletin, 63(6):443–449.
Dalal, N., Triggs, B., and Schmid, C. (2006). Human de-
tection using oriented histograms of flow and appear-
ance. In European conference on computer vision,
pages 428–441. Springer.
Garrido-Moreno, A., Lockett, N., and Garc
´
ıa-Morales, V.
(2018). Social media use and customer engagement.
In Encyclopedia of Information Science and Technol-
ogy, Fourth Edition, pages 5775–5785. IGI Global.
Giannakopoulos, T. (2015). pyaudioanalysis: An open-
source python library for audio signal analysis. PloS
one, 10(12).
Jain, N., Kumar, S., Kumar, A., Shamsolmoali, P., and
Zareapoor, M. (2018). Hybrid deep neural networks
for face emotion recognition. Pattern Recognition Let-
ters.
Li, M., Han, K. J., and Narayanan, S. (2013). Automatic
speaker age and gender recognition using acoustic and
prosodic level information fusion. Computer Speech
& Language, 27(1):151–167.
Liu, B. (2012). Sentiment analysis and opinion mining.
Synthesis Lectures on Human Language Technologies,
5(1):1–167.
Mikolov, T., Chen, K., Corrado, G., and Dean, J. (2013a).
Efficient estimation of word representations in vector
space. In Proceedings of Workshop at International
Conference on Learning Representations.
Mikolov, T., Sutskever, I., Chen, K., Corrado, G. S., and
Dean, J. (2013b). Distributed representations of words
and phrases and their compositionality. In Advances in
Neural Information Processing Systems, pages 3111–
3119.
Misirlis, N. and Vlachopoulou, M. (2018). Social media
metrics and analytics in marketing–s3m: A mapping
literature review. International Journal of Information
Management, 38(1):270–276.
Padilla, R., Costa Filho, C., and Costa, M. (2012). Evalu-
ation of haar cascade classifiers designed for face de-
tection. World Academy of Science, Engineering and
Technology, 64:362–365.
Ravi, K. and Ravi, V. (2015). A survey on opinion mining
and sentiment analysis: Tasks, approaches and appli-
cations. Knowledge-Based Systems, 89:14–46.
Shan, C. (2012). Learning local binary patterns for gen-
der classification on real-world face images. Pattern
recognition letters, 33(4):431–437.
Soleymani, M., Garcia, D., Jou, B., Schuller, B., Chang, S.-
F., and Pantic, M. (2017). A survey of multimodal sen-
timent analysis. Image and Vision Computing, 65:3–
14.
Soliman, A. B., Eissa, K., and El-Beltagy, S. R. (2017).
Aravec: A set of arabic word embedding models for
use in arabic nlp. In Proceedings of the 3rd Interna-
tional Conference on Arabic Computational Linguis-
tics (ACLing 2017), volume 117, pages 256–265.
Multimodal Sentiment and Gender Classification for Video Logs
913