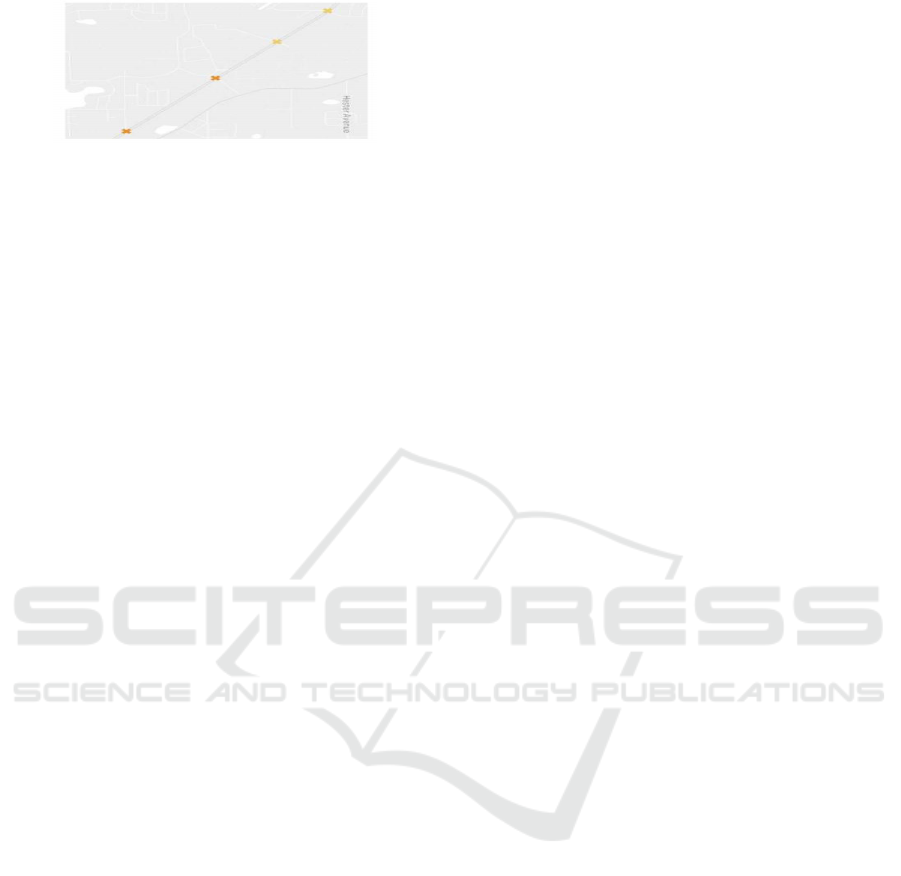
Figure 14: An example where two adjacent signals belong
to different clusters because they register slightly different
demand patterns.
slight differences in behavior, namely that while one
registers high demands till 8pm, the other registers de-
mands till 9pm on weekdays. This example demon-
strates that our clustering technique is sensitive to this
level of granularity. Thus our clustering approach can
be used to understand key behaviors in a grid or net-
work of signalized intersections and hence to improve
the deployed policy. It can also be used to understand
the hours or days for which the traffic patterns are
similar and the time periods for which their might be
some problems.
5 CONCLUSIONS
We developed a data driven approach to process high
resolution (ATSPM) data obtained from traffic con-
trollers. As part of the process, we use split failures
as an MOE and developed algorithms to characterize
the performance of an intersection. We used cluster-
ing as the method of choice for primary data process-
ing. This enabled us to group together signals exhibit-
ing similar behavior. As a result, we highlight signals
that do not belong to the group, but are part of the
same arterial network. Thus, the approach automati-
cally draws attention to signals that need attention in
terms of adjusting the timing plan or fixing of detec-
tor errors. We use a simple classifier to further clas-
sify the signals based on whether they cater to high
or low demand (recorded split failures) and high or
low utilization of green time (based on Arrivals on
Red/Green Ratios).
We visualized the results obtained by analyzing
real data from Florida. Thus, our approach acts as a
decision support system for traffic engineers and traf-
fic managers and informs them about the current per-
formance of the signalized intersection in a region.
The results can be used to easily identify problem-
atic signalized intersections in a proactive manner.
Our overall approach can be further enhanced by effi-
ciently compacting key signal measures in a network
(or region) along both spatial and temporal dimen-
sions.
ACKNOWLEDGEMENTS
The work was supported in part by Florida Depart-
ment of transportation. The opinions, findings and
conclusions expressed in this publication are those of
the author(s) and not necessarily those of the Florida
Department of Transportation or the U.S. Department
of Transportation
REFERENCES
Amini, S., Gerostathopoulos, I., and Prehofer, C. (2017).
Big data analytics architecture for real-time traffic
control. In 2017 5th IEEE International Conference
on Models and Technologies for Intelligent Trans-
portation Systems (MT-ITS), pages 710–715.
Day, C., Bullock, D., Li, H., Remias, S., Hainen, A.,
Freije, R., Stevens, A., Sturdevant, J., and Brennan,
T. (2014). Performance Measures for Traffic Signal
Systems: An Outcome-Oriented Approach.
Horvitz, E., Apacible, J., Sarin, R., and Liao, L. (July,
2005). Prediction, expectation, and surprise: Meth-
ods, designs, and study of a deployed traffic forecast-
ing service. 21st Conference on Uncertainty in Artifi-
cial Intelligence.
Huang, T., Poddar, S., Aguilar, C., Sharma, A., Smaglik,
E., Kothuri, S., and Koonce, P. (2018). Building intel-
ligence in automated traffic signal performance mea-
sures with advanced data analytics. Transportation
Research Record, 0(0):0361198118791380.
Li, H., M. Richardson, L., Day, C., Howard, J., and Bul-
lock, D. (2017). Scalable dashboard for identify-
ing split failures and heuristic for reallocating split
times. Transportation Research Record: Journal of
the Transportation Research Board, 2620:83–95.
Radivojevic, D. and Stevanovic, A. (2017). Framework
for quantitative annual evaluation of traffic signal sys-
tems. Traffic Signal Systems: Volume 1.
Smaglik, E. J., Bullock, D. M., Gettman, D., Day, C. M.,
and Premachandra, H. (2011). Comparison of alter-
native real-time performance measures for measuring
signal phase utilization and identifying oversaturation.
Transportation Research Record, 2259(1):123–131.
UDOT (2017). Udot automated traffic signal performance
measures - automated traffic signal performance met-
rics. https://udottraffic.utah.gov/atspm/. (Accessed on
12/26/2018).
US Department of Transportation, F. H. A. (2008).
Traffic signal timing manual. https://ops.fhwa.dot.
gov/publications/fhwahop08024/index.htm#toc. (Ac-
cessed on 12/26/2018).
US Department of Transportation, F. H. A. (2013). Mea-
sures of effectiveness and validation guidance for
adaptive signal control technologies. https://ops.fhwa.
dot.gov/publications/fhwahop13031/index.htm#toc.
(Accessed on 12/26/2018).
Wemegah, T. D. and Zhu, S. (2017). Big data challenges
in transportation: A case study of traffic volume count
from massive radio frequency identification(rfid) data.
In 2017 International Conference on the Frontiers and
Advances in Data Science (FADS), pages 58–63.
Analyzing Traffic Signal Performance Measures to Automatically Classify Signalized Intersections
147