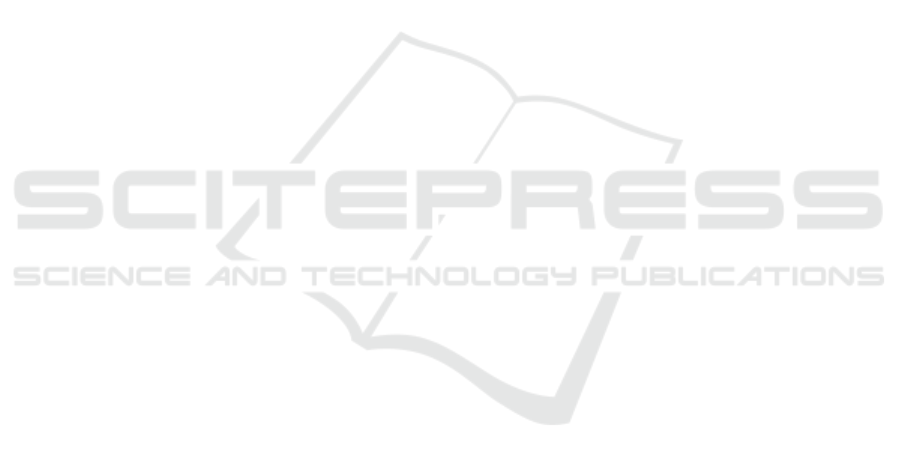
of the prototypes was informed by a review on in-
formation visualisation in and on existing visualisa-
tion dashboards. Some features of the prototypes
are borrowed from existing systems whereas others
are developed from scratch. This paper presented a
case study with findings from a stakeholders’ work-
shop that mainly demonstrated the need for visual-
isations that are as simple as possible and provide
the maximum information in easy-to-read interfaces.
While this may be retrospectively expected, the work-
shop emphasised the need for accompanying visu-
alised findings with recommendations that move be-
yond interpretation and help stakeholders make mean-
ingful fact-based decisions. To cover different needs
of teachers, educators and other stakeholders, a learn-
ing analytics system should be flexible to give them
the ability to choose the information they need to see
as well as the depth and the breadth of the information
presented at different levels of granularity.
With the specific design recommendations we are
making above, this work is a step towards an apprecia-
tion that data analysis cannot act alone in fulfilling the
purpose of communication as it may not convey the
intended messages in an actionable manner. While
further work is needed to understand how best to com-
municate learning analytics in a way that helps stake-
holders make the most out of these tools, the work
presented here demonstrates how an appropriate in-
strument can lead to design requirements that reflect
the actual stakeholders decision-making needs.
ACKNOWLEDGEMENTS
The research leading to these results is co-funded
from the European Union’s Horizon 2020 research
and innovation programme under grant agreement No
732489. For information about the CRISS project see
http://www.crissh2020.eu/
REFERENCES
Ali, L., Hatala, M., Ga
ˇ
sevi
´
c, D., and Jovanovi
´
c, J. (2012).
A qualitative evaluation of evolution of a learning an-
alytics tool. Computers & Education, 58(1):470–489.
Avella, J. T., Kebritchi, M., Nunn, S. G., and Kanai, T.
(2016). Learning analytics methods, benefits, and
challenges in higher education: A systematic litera-
ture review. Online Learning, 20(2):13–29.
Baker, R. S. and Inventado, P. S. (2014). Educational data
mining and learning analytics. In Learning analytics,
pages 61–75. Springer.
Browne, G. J. and Rogich, M. B. (2001). An empirical in-
vestigation of user requirements elicitation: Compar-
ing the effectiveness of prompting techniques. Journal
of Management Information Systems, 17(4):223–249.
Caddick, R. and Cable, S. (2011). Communicating the user
experience: A practical guide for creating useful UX
documentation. John Wiley & Sons.
Chatti, M. A., Dyckhoff, A. L., Schroeder, U., and Th
¨
us,
H. (2012). A reference model for learning analytics.
International Journal of Technology Enhanced Learn-
ing, 4(5-6):318–331.
Cooper, A. et al. (2012). What is analytics? definition
and essential characteristics. CETIS Analytics Series,
1(5):1–10.
Davenport, T. H. and Kim, J. (2013). Keeping up with the
quants: Your guide to understanding and using ana-
lytics. Harvard Business Review Press.
Dawson, S., Ga
ˇ
sevi
´
c, D., Siemens, G., and Joksimovic,
S. (2014). Current state and future trends: A cita-
tion network analysis of the learning analytics field.
In Proceedings of the fourth international conference
on learning analytics and knowledge, pages 231–240.
ACM.
Dietz-Uhler, B. and Hurn, J. E. (2013). Using learning ana-
lytics to predict (and improve) student success: A fac-
ulty perspective. Journal of Interactive Online Learn-
ing, 12(1):17–26.
Dollinger, M. and Lodge, J. M. (2018). Co-creation strate-
gies for learning analytics. In Proceedings of the 8th
International Conference on Learning Analytics and
Knowledge, pages 97–101. ACM.
Drachsler, H. and Greller, W. (2012). Confidence in learn-
ing analytics.
Ferguson, R. (2012). Learning analytics: drivers, develop-
ments and challenges. International Journal of Tech-
nology Enhanced Learning, 4(5/6):304–317.
Fernandes, J., Duarte, D., Ribeiro, C., Farinha, C., Pereira,
J. M., and da Silva, M. M. (2012). ithink: A game-
based approach towards improving collaboration and
participation in requirement elicitation. Procedia
Computer Science, 15:66–77.
Gottesdiener, E. (2003). Requirements by collaboration:
getting it right the first time. IEEE Software, 20(2):52–
55.
Govaerts, S., Verbert, K., Duval, E., and Pardo, A. (2012).
The student activity meter for awareness and self-
reflection. In CHI’12 Extended Abstracts on Human
Factors in Computing Systems, pages 869–884. ACM.
Hickey, A. M. and Davis, A. M. (2004). A unified model
of requirements elicitation. Journal of Management
Information Systems, 20(4):65–84.
Hofmann, H. F. and Lehner, F. (2001). Requirements engi-
neering as a success factor in software projects. IEEE
software, 18(4):58.
Holstein, K., McLaren, B. M., and Aleven, V. (2017). In-
telligent tutors as teachers’ aides: exploring teacher
needs for real-time analytics in blended classrooms.
In Proceedings of the Seventh International Learning
Analytics & Knowledge Conference, pages 257–266.
ACM.
Karkalas, S., Mavrikis, M., et al. (2016). Towards an-
alytics for educational interactive e-books: the case
of the reflective designer analytics platform (rdap).
In Proceedings of the Sixth International Conference
Communicating Learning Analytics: Stakeholder Participation and Early Stage Requirement Analysis
345